Sinogram domain angular upsampling of sparse-view micro-CT with dense residual hierarchical transformer and attention-weighted loss.
Computer methods and programs in biomedicine(2023)
摘要
Reduced angular sampling is a key strategy for increasing scanning efficiency of micron-scale computed tomography (micro-CT). Despite boosting throughput, this strategy introduces noise and extrapolation artifacts due to undersampling. In this work, we present a solution to this issue, by proposing a novel Dense Residual Hierarchical Transformer (DRHT) network to recover high-quality sinograms from 2×, 4× and 8× undersampled scans. DRHT is trained to utilize limited information available from sparsely angular sampled scans and once trained, it can be applied to recover higher-resolution sinograms from shorter scan sessions. Our proposed DRHT model aggregates the benefits of a hierarchical- multi-scale structure along with the combination of local and global feature extraction through dense residual convolutional blocks and non-overlapping window transformer blocks respectively. We also propose a novel noise-aware loss function named KL-L1 to improve sinogram restoration to full resolution. KL-L1, a weighted combination of pixel-level and distribution-level cost functions, leverages inconsistencies in noise distribution and uses learnable spatial weight maps to improve the training of the DRHT model. We present ablation studies and evaluations of our method against other state-of-the-art (SOTA) models over multiple datasets. Our proposed DRHT network achieves an average increase in peak signal to noise ratio (PSNR) of 17.73 dB and a structural similarity index (SSIM) of 0.161, for 8× upsampling, across the three diverse datasets, compared to their respective Bicubic interpolated versions. This novel approach can be utilized to decrease radiation exposure to patients and reduce imaging time for large-scale CT imaging projects.
更多查看译文
关键词
Micro-CT,Sparse view,Attention weighted loss
AI 理解论文
溯源树
样例
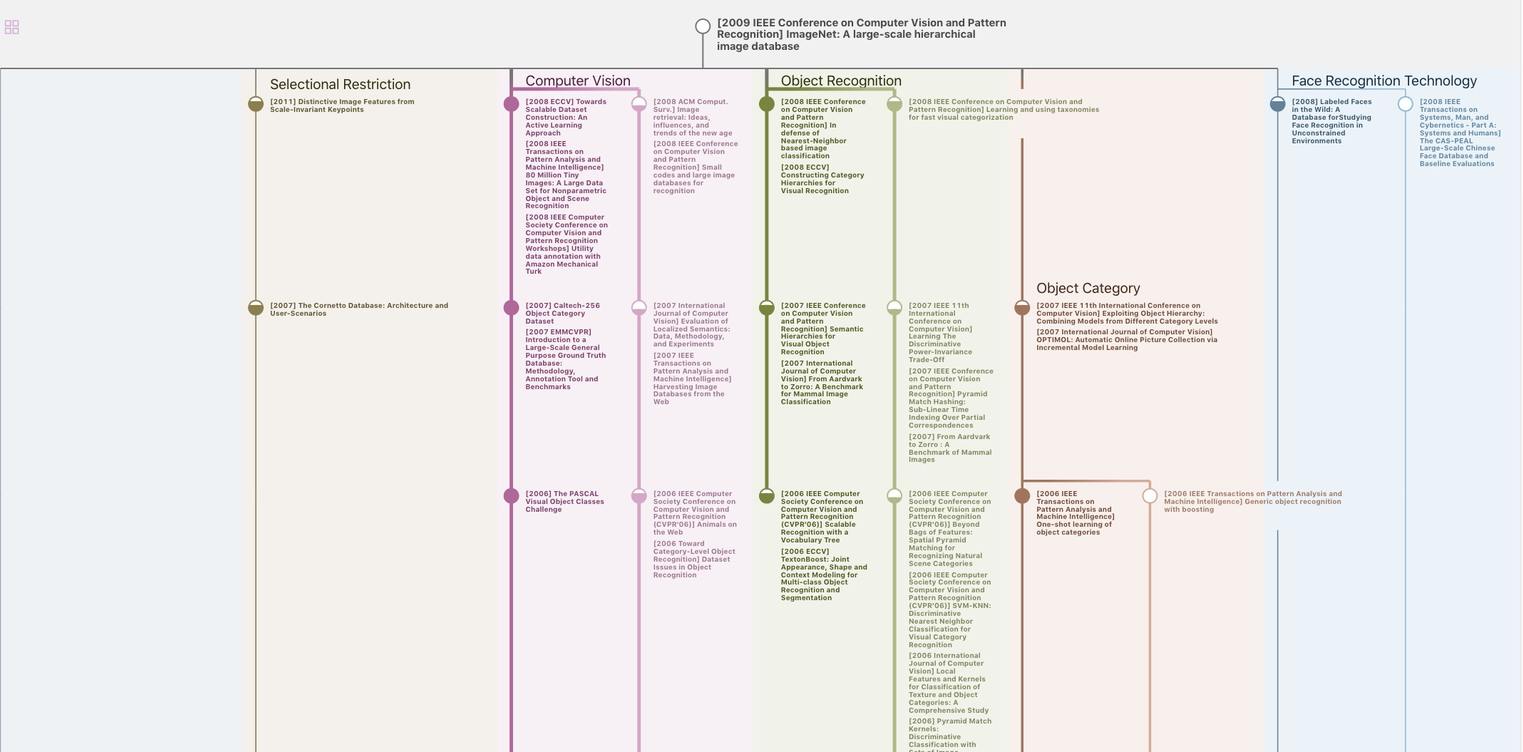
生成溯源树,研究论文发展脉络
Chat Paper
正在生成论文摘要