Sample Size in Floor Sensor-based Gait Recognition for Smart Home and Access Control Scenarios
2023 IEEE Sensors Applications Symposium (SAS)(2023)
摘要
Floor sensor-based gait recognition is an emerging biometric technology that can be used in a variety of scenarios ranging from access control to personalized recognition for smart home systems. These distinct scenarios can differ based on: (1) the number of users in the system, N-user, and (2) the number of training footstep samples per user made available during the enrollment process, N-step. In this study, the effect of these two parameters on the accuracy of state-of-the-art machine learning (ML) and deep learning (DL) models was investigated. For the smart home scenario (small N-user, large N-step), a best person verification performance of 99.77% accuracy was found using a lightweight convolutional neural network (CNN) model with spatial image features. For the access control scenario (large N-user, small N-step), on the other hand, a highest accuracy of 90.70% was found using a shallow k-nearest neighbor classifier with spatiotemporal information, and not end-to-end CNNs or transfer learning based on a pre-trained ResNet-50. Finally, a learning curve analysis is conducted to inform how many training footsteps are needed for both ML and DL approaches to person verification based on different numbers of users.
更多查看译文
关键词
biometric,convolutional neural network,CNN,deep learning,gait recognition,plantar pressure
AI 理解论文
溯源树
样例
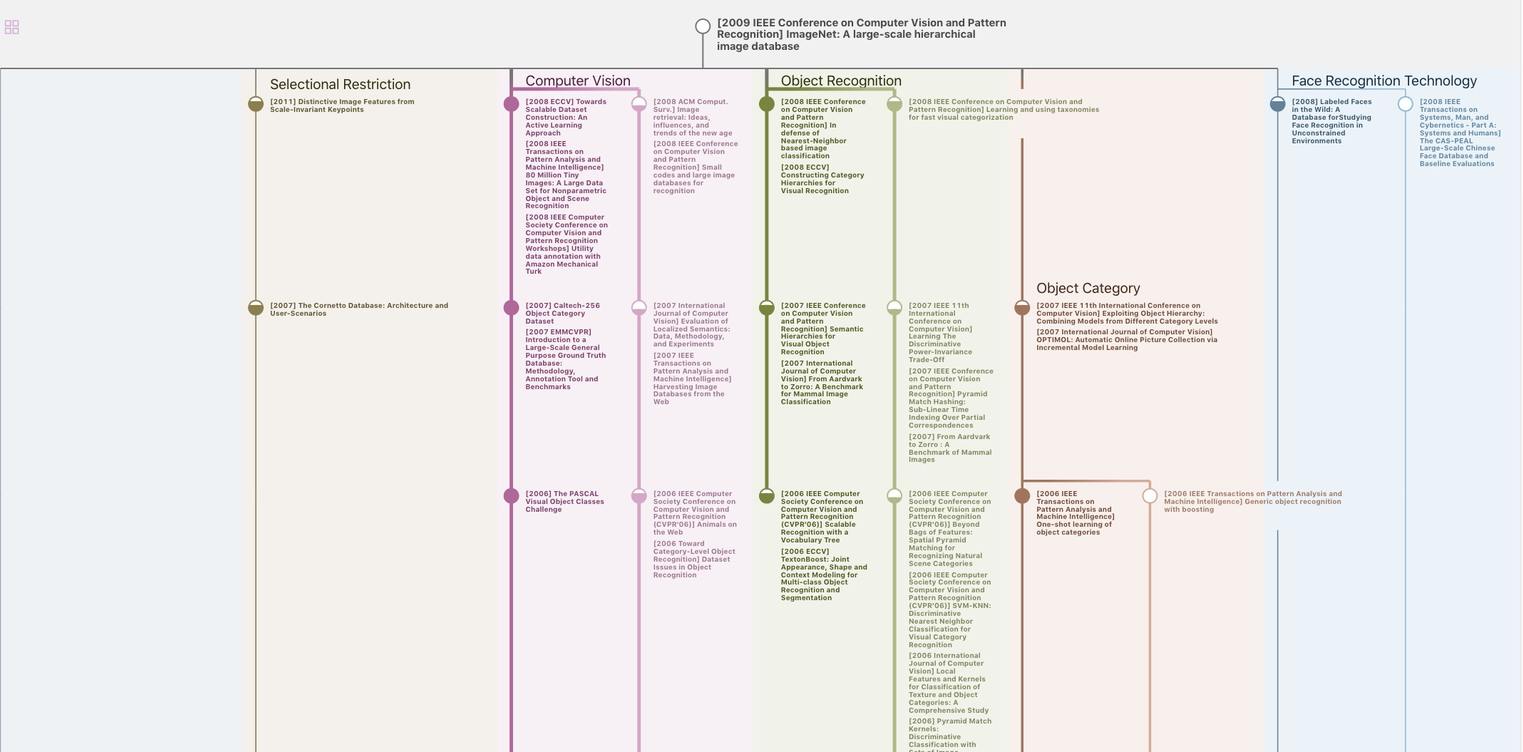
生成溯源树,研究论文发展脉络
Chat Paper
正在生成论文摘要