Simulation of In-Situ Training in Spike Neural Network Based on Non-Ideal Memristors
IEEE Journal of the Electron Devices Society(2023)
摘要
In this paper, we proposed a multilayer supervised training method based on Multi-Resume and memristor synaptic properties. We use a new differential equation describe the dynamic of Pt/HfO2/Al2O3/Ti devices and update weights based on present conductance. Specifically, the weight update is achieved by applying just one pulse to the device, which will simplify the peripheral circuits. In addition, the algorithm training method, asymmetric nonlinear weight update and synaptic variation measured from experiments are investigated for the impact on network accuracy. Our results show that the nonlinearity of devices does not much affect the network accuracy; The hybrid training is a better method for ensuring the accuracy; The spiking neural network (SNN) shows remarkable high tolerance to the variation of the device. This work will lay the foundation for later on-chip learning of SNN based on memristors. (Optdigits dataset:
https://archive.ics.uci.edu/ml
)
更多查看译文
关键词
Memristor, synaptic plasticity, nonlinearity, asymmetry, spike neural network
AI 理解论文
溯源树
样例
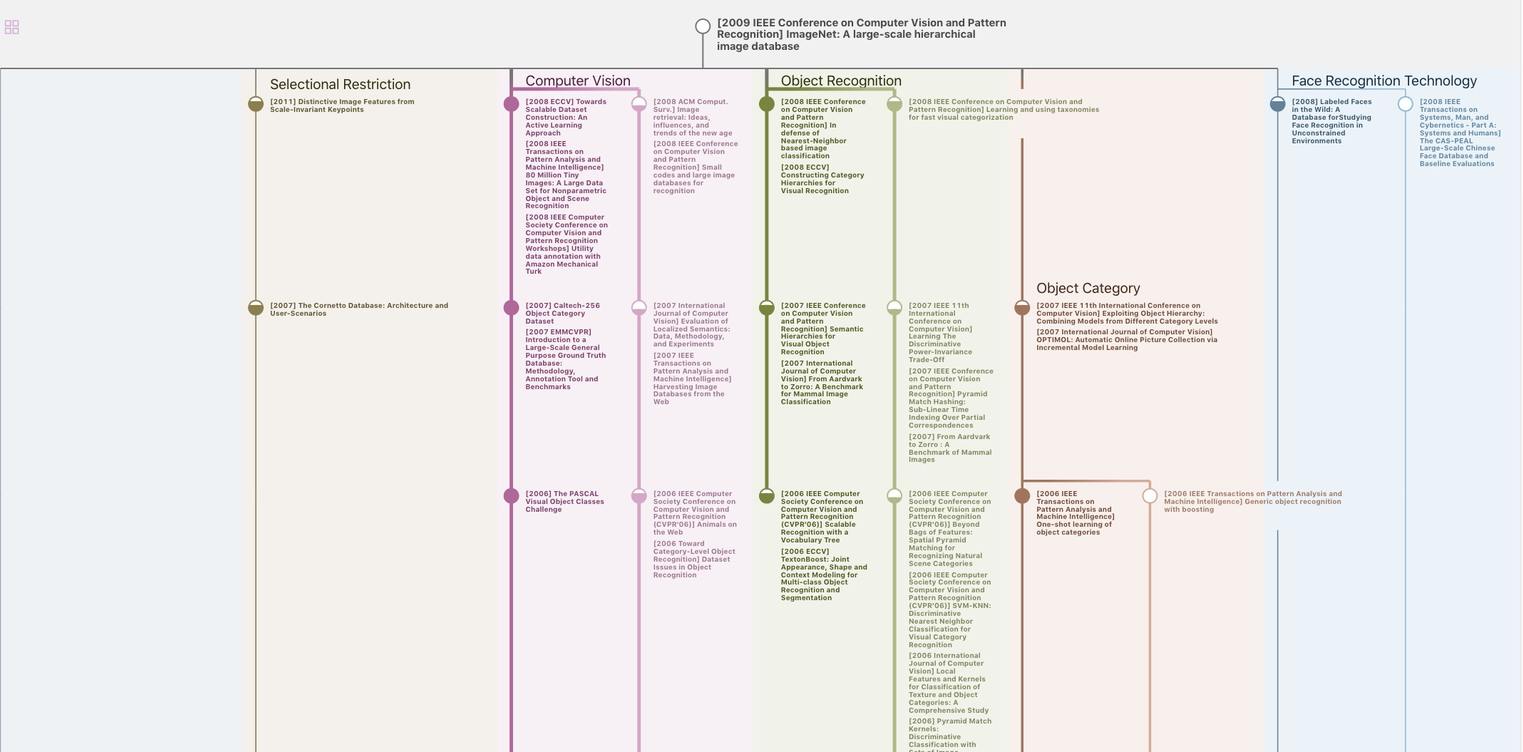
生成溯源树,研究论文发展脉络
Chat Paper
正在生成论文摘要