Harmonization of supervised machine learning practices for efficient source attribution of Listeria monocytogenes based on genomic data
BMC Genomics(2023)
摘要
Background Genomic data-based machine learning tools are promising for real-time surveillance activities performing source attribution of foodborne bacteria such as Listeria monocytogenes . Given the heterogeneity of machine learning practices, our aim was to identify those influencing the source prediction performance of the usual holdout method combined with the repeated k-fold cross-validation method. Methods A large collection of 1 100 L. monocytogenes genomes with known sources was built according to several genomic metrics to ensure authenticity and completeness of genomic profiles. Based on these genomic profiles (i.e. 7-locus alleles, core alleles, accessory genes, core SNPs and pan kmers), we developed a versatile workflow assessing prediction performance of different combinations of training dataset splitting (i.e. 50, 60, 70, 80 and 90%), data preprocessing (i.e. with or without near-zero variance removal), and learning models (i.e. BLR, ERT, RF, SGB, SVM and XGB). The performance metrics included accuracy, Cohen’s kappa, F1-score, area under the curves from receiver operating characteristic curve, precision recall curve or precision recall gain curve, and execution time. Results The testing average accuracies from accessory genes and pan kmers were significantly higher than accuracies from core alleles or SNPs. While the accuracies from 70 and 80% of training dataset splitting were not significantly different, those from 80% were significantly higher than the other tested proportions. The near-zero variance removal did not allow to produce results for 7-locus alleles, did not impact significantly the accuracy for core alleles, accessory genes and pan kmers, and decreased significantly accuracy for core SNPs. The SVM and XGB models did not present significant differences in accuracy between each other and reached significantly higher accuracies than BLR, SGB, ERT and RF, in this order of magnitude. However, the SVM model required more computing power than the XGB model, especially for high amount of descriptors such like core SNPs and pan kmers. Conclusions In addition to recommendations about machine learning practices for L. monocytogenes source attribution based on genomic data, the present study also provides a freely available workflow to solve other balanced or unbalanced multiclass phenotypes from binary and categorical genomic profiles of other microorganisms without source code modifications.
更多查看译文
关键词
Source attribution,Listeria monocytogenes,Machine learning,Genomic data
AI 理解论文
溯源树
样例
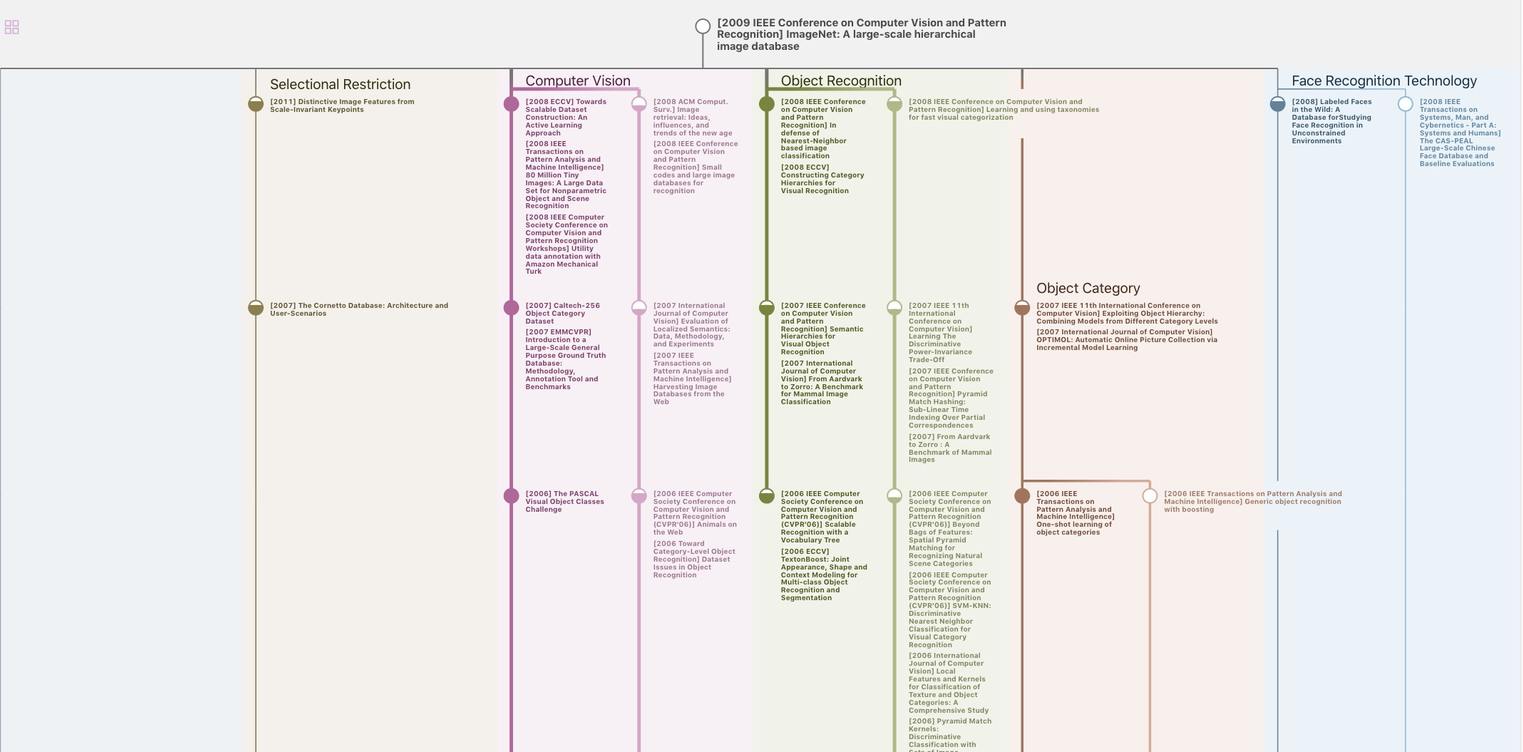
生成溯源树,研究论文发展脉络
Chat Paper
正在生成论文摘要