Evaluation of Robustness Metrics for Defense of Machine Learning Systems
2023 International Conference on Military Communications and Information Systems (ICMCIS)(2023)
摘要
In this paper we explore some of the potential applications of robustness criteria for machine learning (ML) systems by way of tangible “demonstrator” scenarios. In each demonstrator, ML robustness metrics are applied to real-world scenarios with military relevance, indicating how they might be used to help detect and handle possible adversarial attacks on ML systems. We conclude by sketching promising future avenues of research in order to: (1) help establish useful verification methodologies to facilitate ML robustness compliance assessment; (2) support development of ML accountability mechanisms; and (3) reliably detect, repel, and mitigate adversarial attack.
更多查看译文
AI 理解论文
溯源树
样例
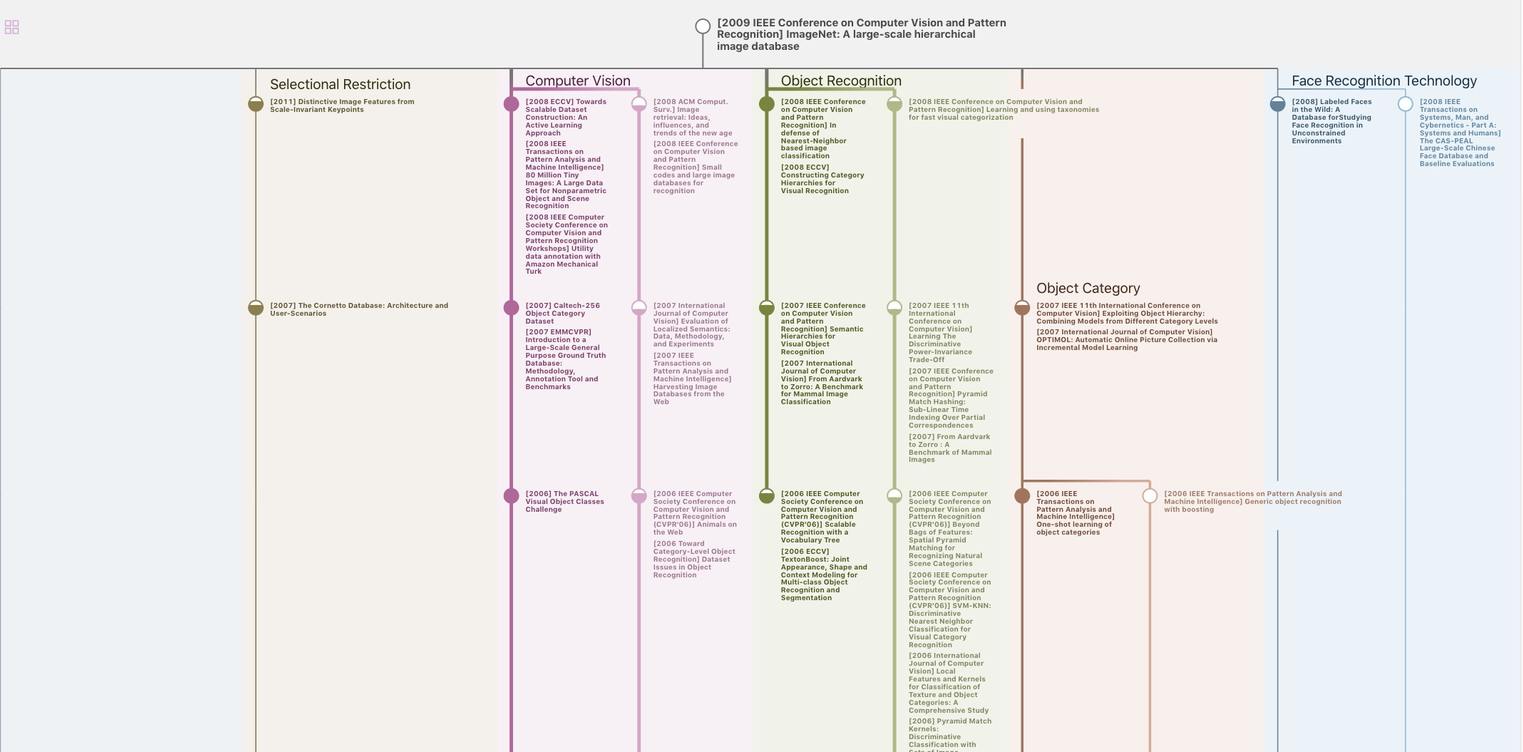
生成溯源树,研究论文发展脉络
Chat Paper
正在生成论文摘要