Drug-target binding affinity prediction using message passing neural network and self supervised learning
BMC genomics(2023)
摘要
Background Drug-target binding affinity (DTA) prediction is important for the rapid development of drug discovery. Compared to traditional methods, deep learning methods provide a new way for DTA prediction to achieve good performance without much knowledge of the biochemical background. However, there are still room for improvement in DTA prediction: (1) only focusing on the information of the atom leads to an incomplete representation of the molecular graph; (2) the self-supervised learning method could be introduced for protein representation. Results In this paper, a DTA prediction model using the deep learning method is proposed, which uses an undirected-CMPNN for molecular embedding and combines CPCProt and MLM models for protein embedding. An attention mechanism is introduced to discover the important part of the protein sequence. The proposed method is evaluated on the datasets Ki and Davis, and the model outperformed other deep learning methods. Conclusions The proposed model improves the performance of the DTA prediction, which provides a novel strategy for deep learning-based virtual screening methods.
更多查看译文
关键词
Drug-target binding affinity, Self-supervised learning method, Molecular representation, Protein representation
AI 理解论文
溯源树
样例
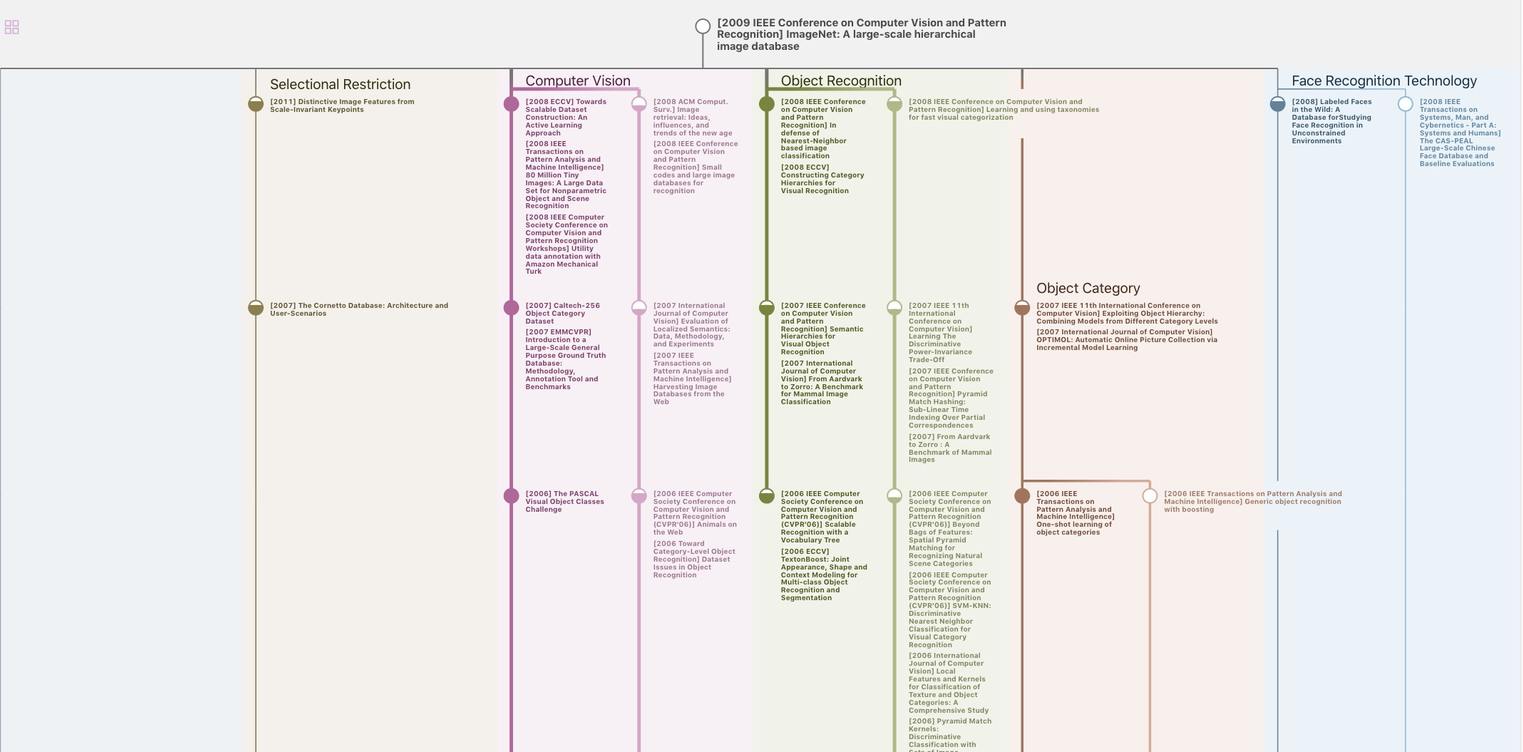
生成溯源树,研究论文发展脉络
Chat Paper
正在生成论文摘要