TinyCLIP: CLIP Distillation via Affinity Mimicking and Weight Inheritance
Proceedings of the IEEE/CVF International Conference on Computer Vision (ICCV)(2023)
摘要
In this paper, we propose a novel cross-modal distillation method, called TinyCLIP, for large-scale language-image pre-trained models. The method introduces two core techniques: affinity mimicking and weight inheritance. Affinity mimicking explores the interaction between modalities during distillation, enabling student models to mimic teachers' behavior of learning cross-modal feature alignment in a visual-linguistic affinity space. Weight inheritance transmits the pre-trained weights from the teacher models to their student counterparts to improve distillation efficiency. Moreover, we extend the method into a multi-stage progressive distillation to mitigate the loss of informative weights during extreme compression. Comprehensive experiments demonstrate the efficacy of TinyCLIP, showing that it can reduce the size of the pre-trained CLIP ViT-B/32 by 50%, while maintaining comparable zero-shot performance. While aiming for comparable performance, distillation with weight inheritance can speed up the training by 1.4 - 7.8 $\times$ compared to training from scratch. Moreover, our TinyCLIP ViT-8M/16, trained on YFCC-15M, achieves an impressive zero-shot top-1 accuracy of 41.1% on ImageNet, surpassing the original CLIP ViT-B/16 by 3.5% while utilizing only 8.9% parameters. Finally, we demonstrate the good transferability of TinyCLIP in various downstream tasks. Code and models will be open-sourced at https://aka.ms/tinyclip.
更多查看译文
关键词
tinyclip distillation,affinity mimicking
AI 理解论文
溯源树
样例
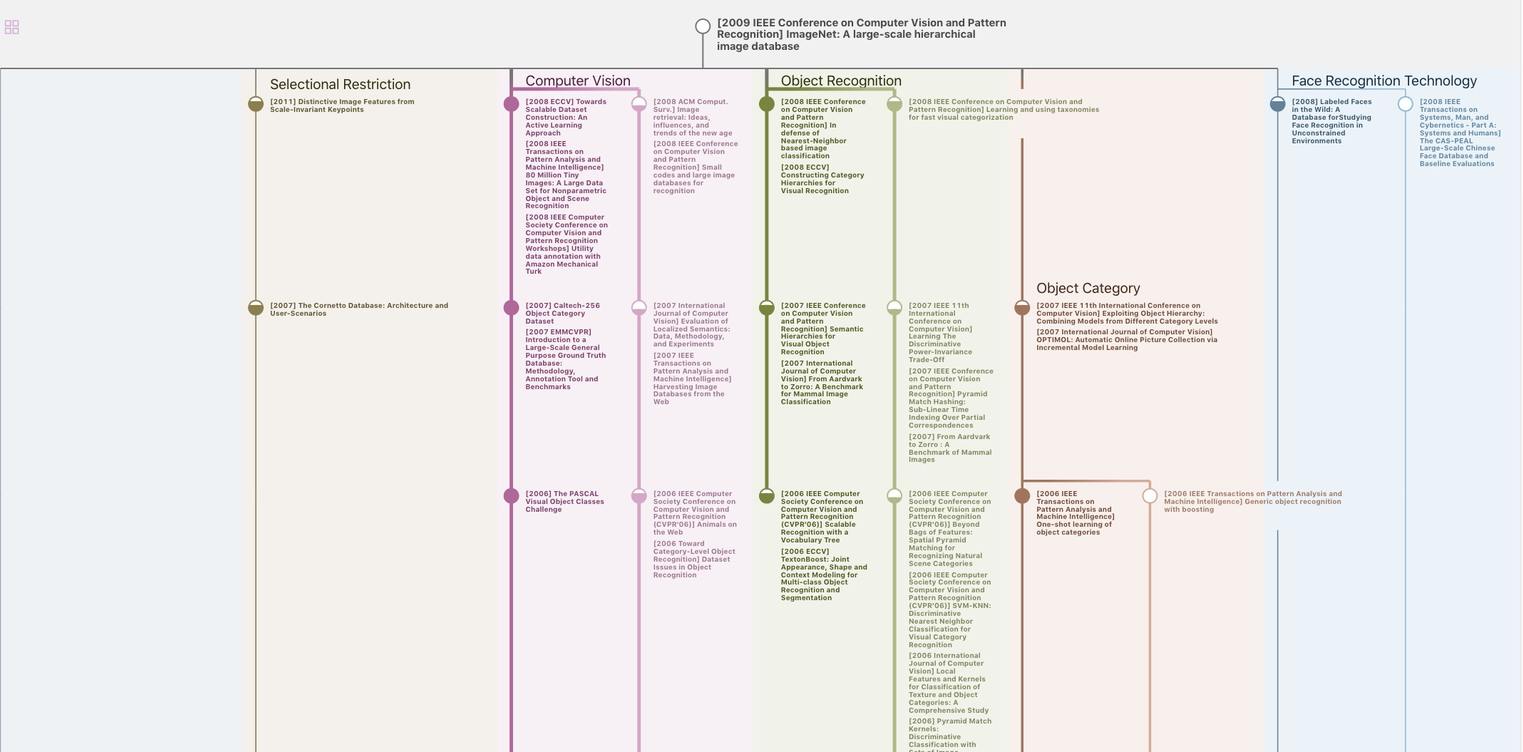
生成溯源树,研究论文发展脉络
Chat Paper
正在生成论文摘要