Bridging the Gaps of Both Modality and Language: Synchronous Bilingual CTC for Speech Translation and Speech Recognition
ICASSP 2024 - 2024 IEEE International Conference on Acoustics, Speech and Signal Processing (ICASSP)(2023)
摘要
In this study, we present synchronous bilingual Connectionist Temporal Classification (CTC), an innovative framework that leverages dual CTC to bridge the gaps of both modality and language in the speech translation (ST) task. Utilizing transcript and translation as concurrent objectives for CTC, our model bridges the gap between audio and text as well as between source and target languages. Building upon the recent advances in CTC application, we develop an enhanced variant, BiL-CTC+, that establishes new state-of-the-art performances on the MuST-C ST benchmarks under resource-constrained scenarios. Intriguingly, our method also yields significant improvements in speech recognition performance, revealing the effect of cross-lingual learning on transcription and demonstrating its broad applicability. The source code is available at https://github.com/xuchennlp/S2T.
更多查看译文
关键词
Connectionist Temporal Classification,Bilingual Prediction,Speech Translation,Speech Recognition
AI 理解论文
溯源树
样例
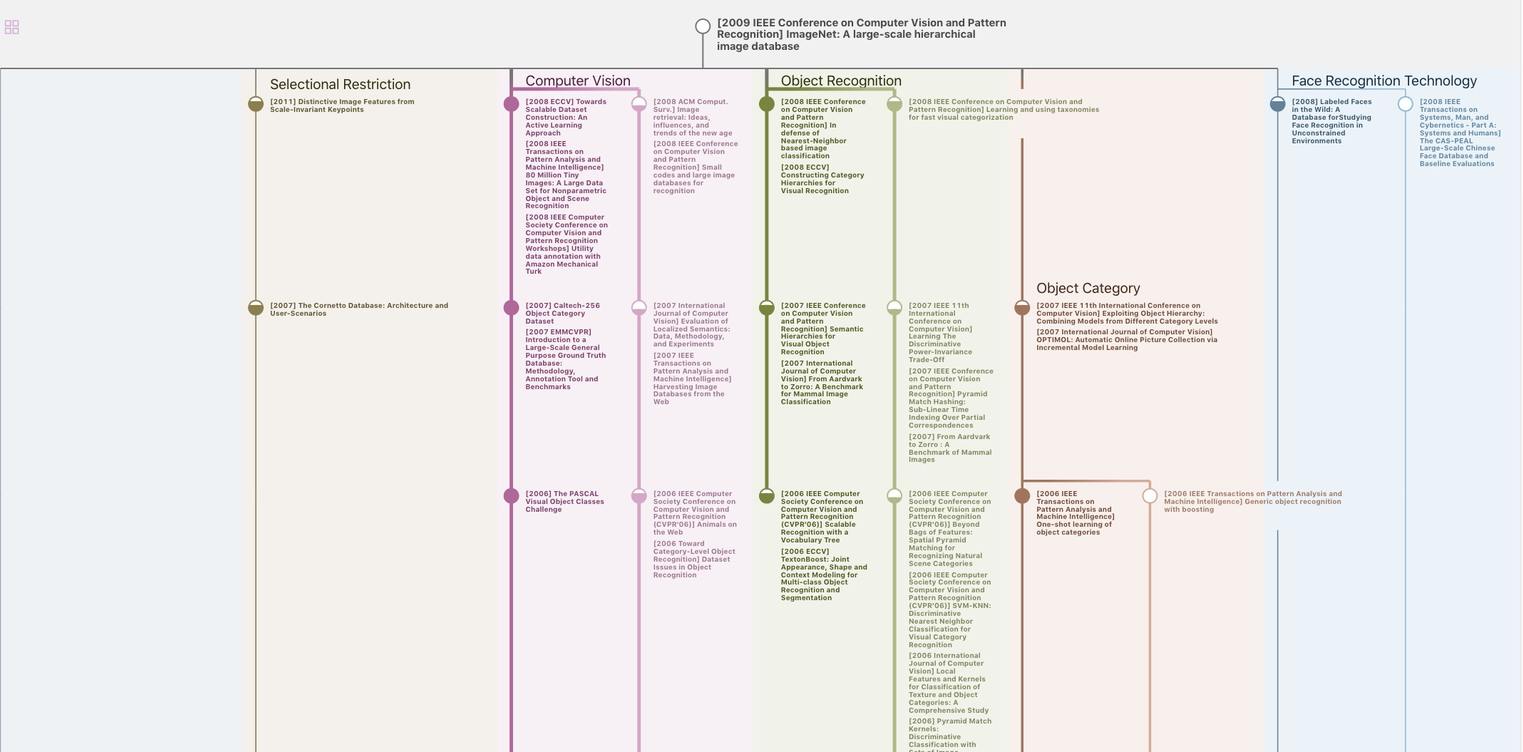
生成溯源树,研究论文发展脉络
Chat Paper
正在生成论文摘要