ContextRef: Evaluating Referenceless Metrics For Image Description Generation
arXiv (Cornell University)(2023)
摘要
Referenceless metrics (e.g., CLIPScore) use pretrained vision--language models to assess image descriptions directly without costly ground-truth reference texts. Such methods can facilitate rapid progress, but only if they truly align with human preference judgments. In this paper, we introduce ContextRef, a benchmark for assessing referenceless metrics for such alignment. ContextRef has two components: human ratings along a variety of established quality dimensions, and ten diverse robustness checks designed to uncover fundamental weaknesses. A crucial aspect of ContextRef is that images and descriptions are presented in context, reflecting prior work showing that context is important for description quality. Using ContextRef, we assess a variety of pretrained models, scoring functions, and techniques for incorporating context. None of the methods is successful with ContextRef, but we show that careful fine-tuning yields substantial improvements. ContextRef remains a challenging benchmark though, in large part due to the challenge of context dependence.
更多查看译文
关键词
image description,referenceless metrics
AI 理解论文
溯源树
样例
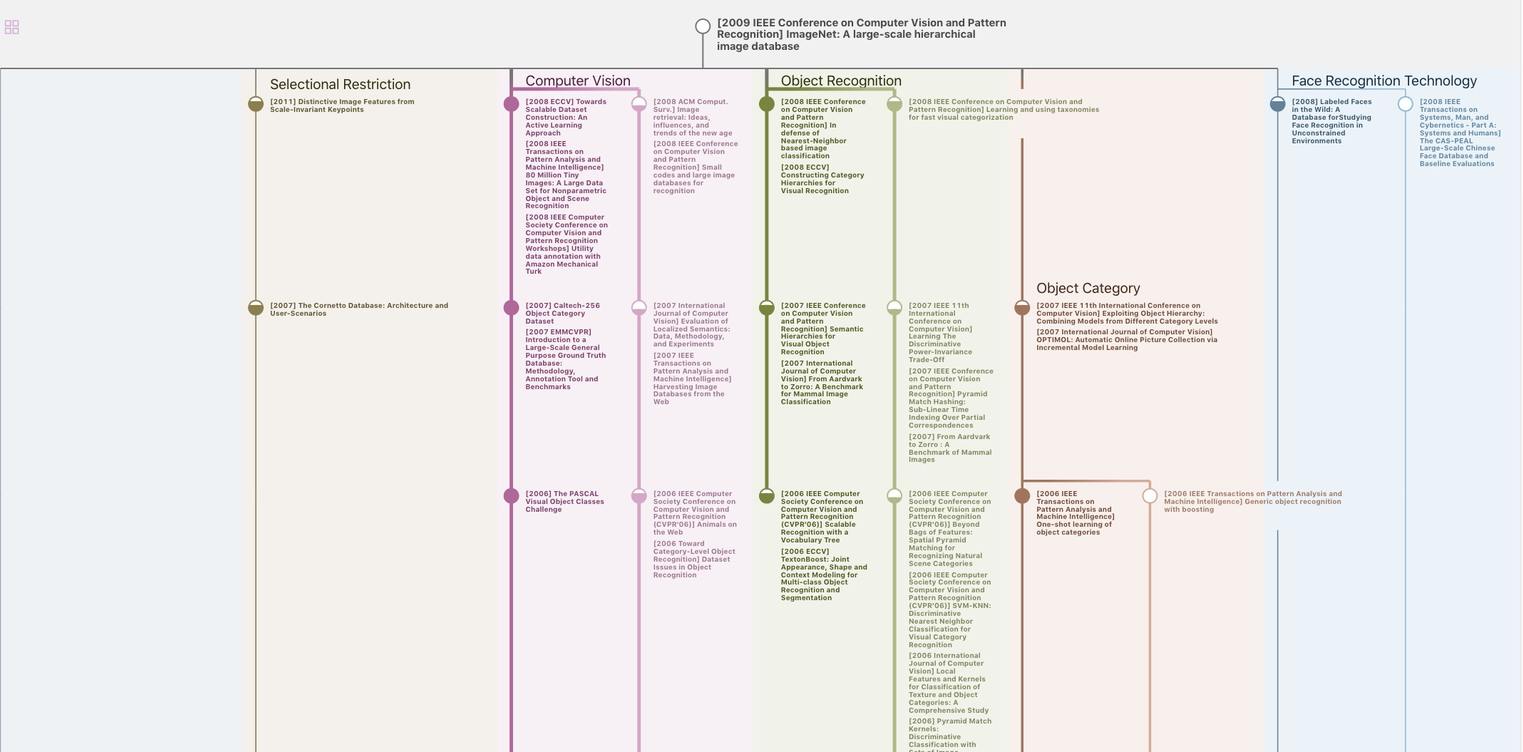
生成溯源树,研究论文发展脉络
Chat Paper
正在生成论文摘要