Construction of Paired Knowledge Graph-Text Datasets Informed by Cyclic Evaluation
CoRR(2023)
摘要
Datasets that pair Knowledge Graphs (KG) and text together (KG-T) can be used to train forward and reverse neural models that generate text from KG and vice versa. However models trained on datasets where KG and text pairs are not equivalent can suffer from more hallucination and poorer recall. In this paper, we verify this empirically by generating datasets with different levels of noise and find that noisier datasets do indeed lead to more hallucination. We argue that the ability of forward and reverse models trained on a dataset to cyclically regenerate source KG or text is a proxy for the equivalence between the KG and the text in the dataset. Using cyclic evaluation we find that manually created WebNLG is much better than automatically created TeKGen and T-REx. Guided by these observations, we construct a new, improved dataset called LAGRANGE using heuristics meant to improve equivalence between KG and text and show the impact of each of the heuristics on cyclic evaluation. We also construct two synthetic datasets using large language models (LLMs), and observe that these are conducive to models that perform significantly well on cyclic generation of text, but less so on cyclic generation of KGs, probably because of a lack of a consistent underlying ontology.
更多查看译文
关键词
knowledge,datasets,cyclic
AI 理解论文
溯源树
样例
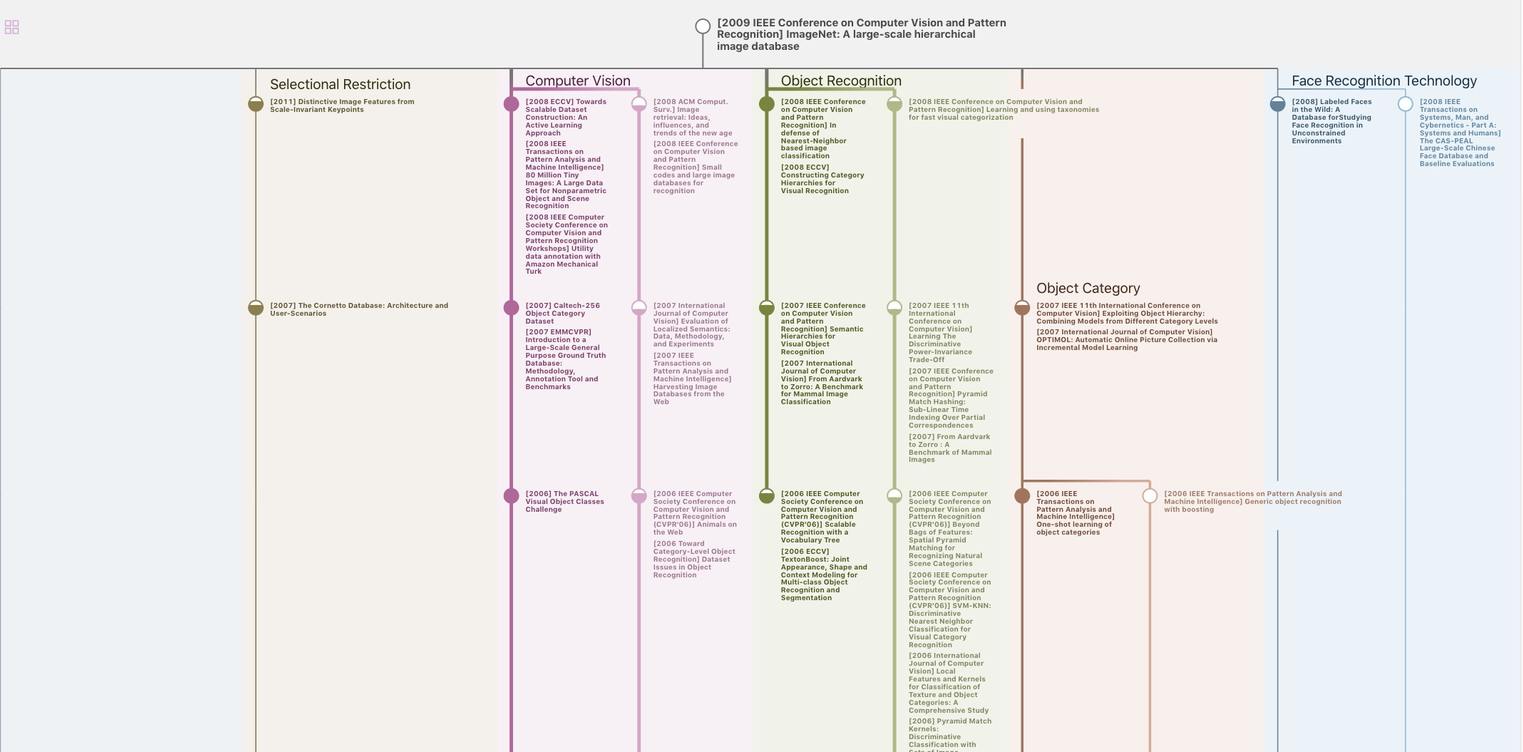
生成溯源树,研究论文发展脉络
Chat Paper
正在生成论文摘要