SIT-Net: Surgical Image Translation Network Using a Generative Adversarial Method with Visual Feature Disentanglement
2023 IEEE International Conference on Real-time Computing and Robotics (RCAR)(2023)
摘要
With flourishing advancements of surgical robots and learning-driven algorithms, surgical automation that enables robots with autonomous functions for certain subtasks by learning experts’ skills is attracting tremendous attention. To facilitate its development, introducing a high-quality surgical image translation method from current scenes to desired domains can not only augment the data for model training but also alleviate the problems that may frequently encounter using traditional approaches, like visual blurs or incomplete instrument generations. To this end, we propose a new surgical image generation method called Surgical Image Translation Network (SIT-Net). Considering complex features entangled within surgical images, a novel generator that leverages tool masks to disentangle the foreground and background characteristics is proposed. Besides, to retain the completeness of instruments, a novel loss function that can preserve tools’ features is introduced to further supervise the model training process. We extensively evaluated our method in terms of the visual appearance of translated images and adopted an evaluation indicator Fréchet Inception Distance (FID) to verify whether the generated images are close to the target domain or not. Both the qualitative and quantitative outcomes demonstrate that our SIT-Net is superior to the traditional approach regarding surgical image translation and generation in desired scenes.
更多查看译文
AI 理解论文
溯源树
样例
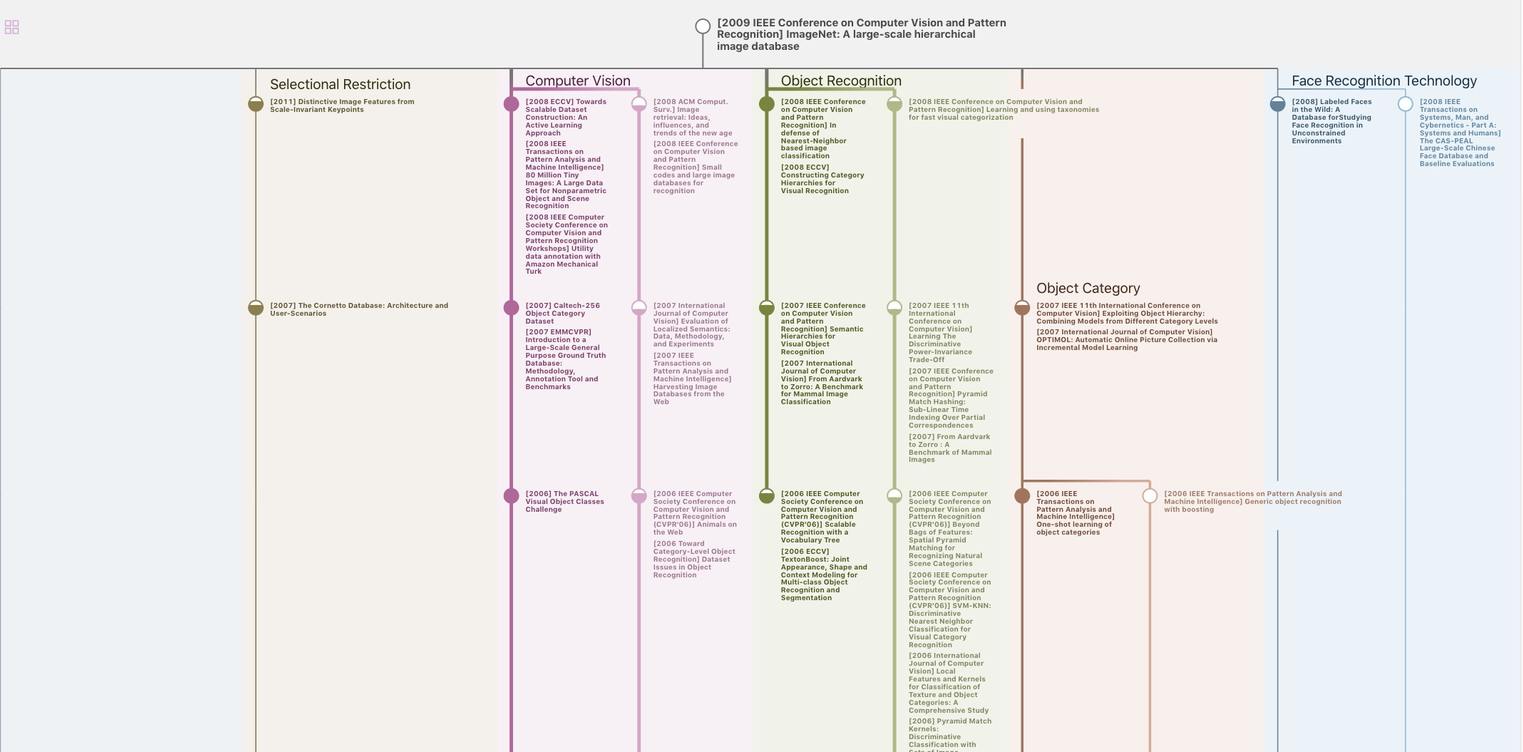
生成溯源树,研究论文发展脉络
Chat Paper
正在生成论文摘要