Real-Time Multispectral Pedestrian Detection with Weakly Aligned Cross-Modal Learning
2023 IEEE International Conference on Real-time Computing and Robotics (RCAR)(2023)
摘要
Over the past ten years, multispectral pedestrian detection has attracted a lot of interest. The RGB-thermal image pairs used in existing methods are well-aligned by default, but there is a weak alignment issue between both image pairs captured by different sensors, which leads to the inaccuracy of pedestrian detection. To alleviate the problem of weak alignment in multispectral tasks, a cross-modal learning network (CMLNet) is proposed in this paper. A novel spatial-semantic alignment strategy is firstly designed to align the RGB-thermal features with the spatial transformation and semantic mapping between both modalities. A feature reselection module is implemented to filter the redundant features before the fusion. Finally, YOLOX is chosen as the detection framework. The open KAIST dataset is used to validate the suggested technique. Experimental results demonstrate that the proposed method can be applied in real-time applications, i.e., the pedestrian can be detected in 16 ms for each pair of RGB-thermal images. And the miss rate of pedestrian detection can reach 18.12% with competitive performance, compared with the state-of-the-art approaches.
更多查看译文
AI 理解论文
溯源树
样例
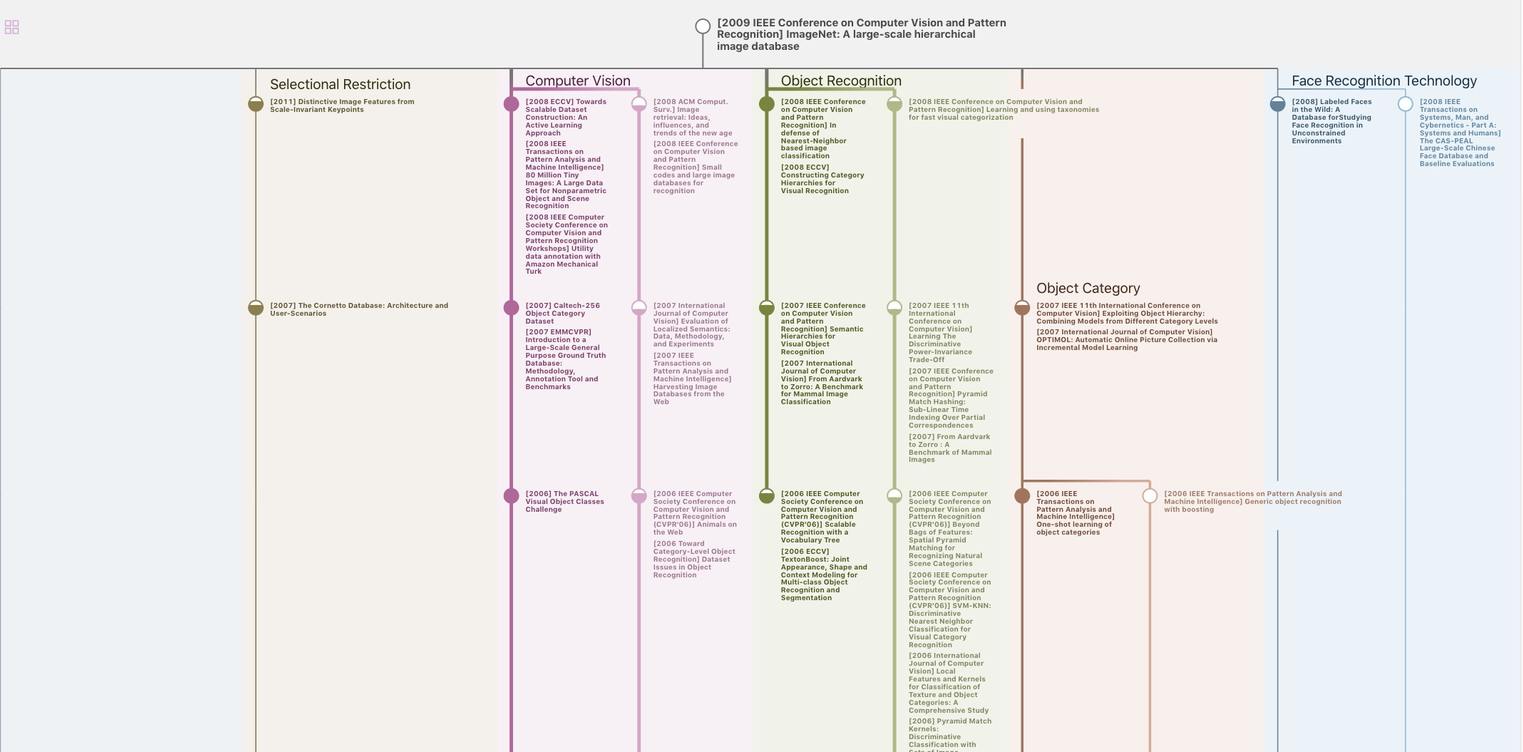
生成溯源树,研究论文发展脉络
Chat Paper
正在生成论文摘要