Evaluation of Continual Learning Methods for Object Hardness Recognition
2023 IEEE International Conference on Real-time Computing and Robotics (RCAR)(2023)
摘要
How to solve the problem of continual learning of robotic tactile perception in an open, dynamic environment is a pressing task. Although continual learning has been extensively studied in computer vision, the evaluation of those algorithms does not provide insights on whether their solutions may help continual learning in the context of tactile robotics. In this paper, we propose a continual learning scenario in the robotic tactile domain, and design experiments with tactile hardness dataset that allow us to compare the performance of many continual learning algorithms in the tactile domain. We examine the performance of regularization-based and replay methods in the tactile domain and discuss the effect of replay number on model performance. We show that regularization-based methods do not perform well on the tactile dataset. On the contrary, replay methods can maintain better anti-forgetting performance, but the number of model parameters will be larger, and the training time will be longer. We also found that the replay ratio should be higher than 0.2 to maintain the performance of the replay method on the tactile hardness dataset.
更多查看译文
关键词
Continual Learning,Robotic Tactile Perception,Catastrophic Forgetting
AI 理解论文
溯源树
样例
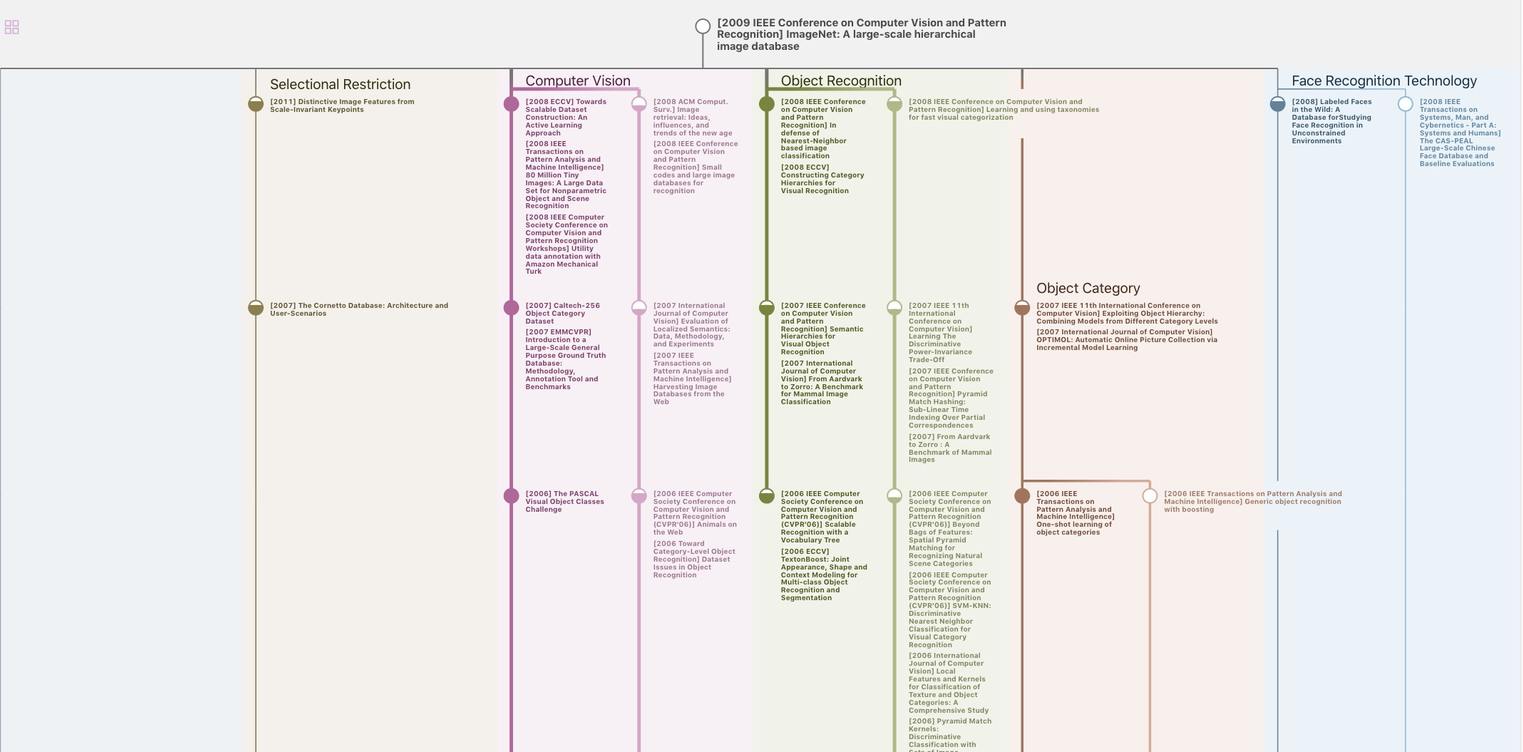
生成溯源树,研究论文发展脉络
Chat Paper
正在生成论文摘要