Offline Model-Based Adaptable Policy Learning for Decision-Making in Out-of-Support Regions
IEEE TRANSACTIONS ON PATTERN ANALYSIS AND MACHINE INTELLIGENCE(2023)
摘要
In reinforcement learning, a promising direction to avoid online trial-and-error costs is learning from an offline dataset. Current offline reinforcement learning methods commonly learn in the policy space constrained to in-support regions by the offline dataset, in order to ensure the robustness of the outcome policies. Such constraints, however, also limit the potential of the outcome policies. In this paper, to release the potential of offline policy learning, we investigate the decision-making problems in out-of-support regions directly and propose offline Model-based Adaptable Policy LEarning (MAPLE). By this approach, instead of learning in in-support regions, we learn an adaptable policy that can adapt its behavior in out-of-support regions when deployed. We give a practical implementation of MAPLE via meta-learning techniques and ensemble model learning techniques. We conduct experiments on MuJoCo locomotion tasks with offline datasets. The results show that the proposed method can make robust decisions in out-of-support regions and achieve better performance than SOTA algorithms.
更多查看译文
关键词
Adaptation models,Uncertainty,Predictive models,Behavioral sciences,Extrapolation,Trajectory,Reinforcement learning,Adaptable policy learning,meta learning,model-based reinforcement learning,offline reinforcement learning
AI 理解论文
溯源树
样例
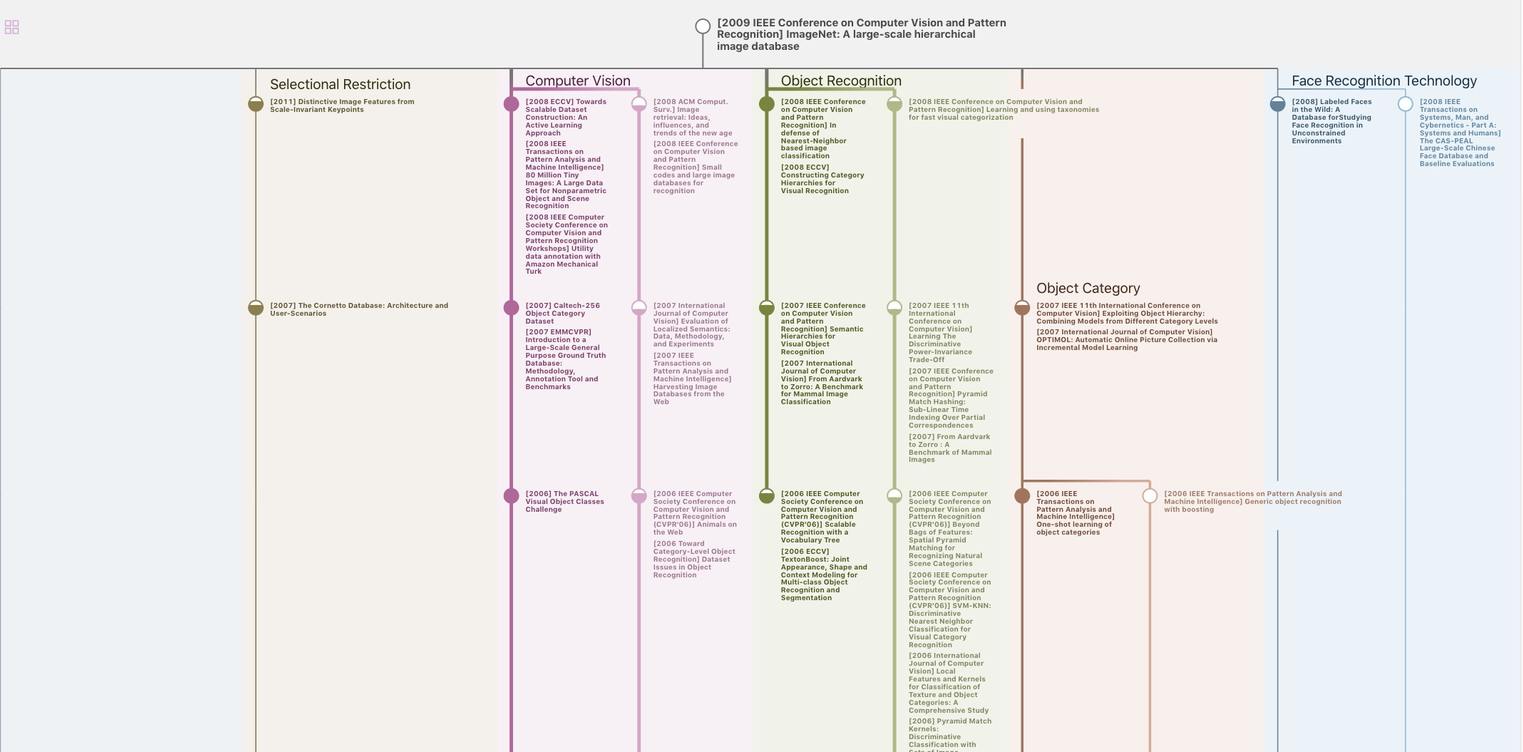
生成溯源树,研究论文发展脉络
Chat Paper
正在生成论文摘要