Multimodal Glioma Segmentation with Fusion of Multiple Self-attention and Deformable Convolutions
Chinese Journal of Magnetic Resonance(2023)
摘要
The magnetic resonance image segmentation of glioma is significant in disease diagnosis, surgical planning, and determination of treatment plans such as radiotherapy. In response to the problem of low segmentation accuracy, inaccurate edge segmentation, and prone to false positives in existing brain tumor segmentation algorithms, an improved Unet model based on multi-head self-attention and deformable convolution is proposed. The model replaces the standard convolution of the original Unet framework with residual modules to prevent vanishing gradient during model training. Multi-head self-attention modules based on Transformer are added in the bottleneck layer to extract local features and global context information for better exploration of correlations between pixels. Deformable convolution is used at cross-layer connections to enhance the model's sensitivity to shape perception and improve the ability to extract tumor edge features. Experimental results show that the segmentation evaluation metrics of the proposed algorithm are higher than those of other comparative literature and models using the same dataset, with more precise segmentation of tumor edges. This indicates that the algorithm proposed in this paper is an effective automatic glioma segmentation algorithm.
更多查看译文
关键词
image segmentation,glioma,unet,transformer self-attention,deformable convolution
AI 理解论文
溯源树
样例
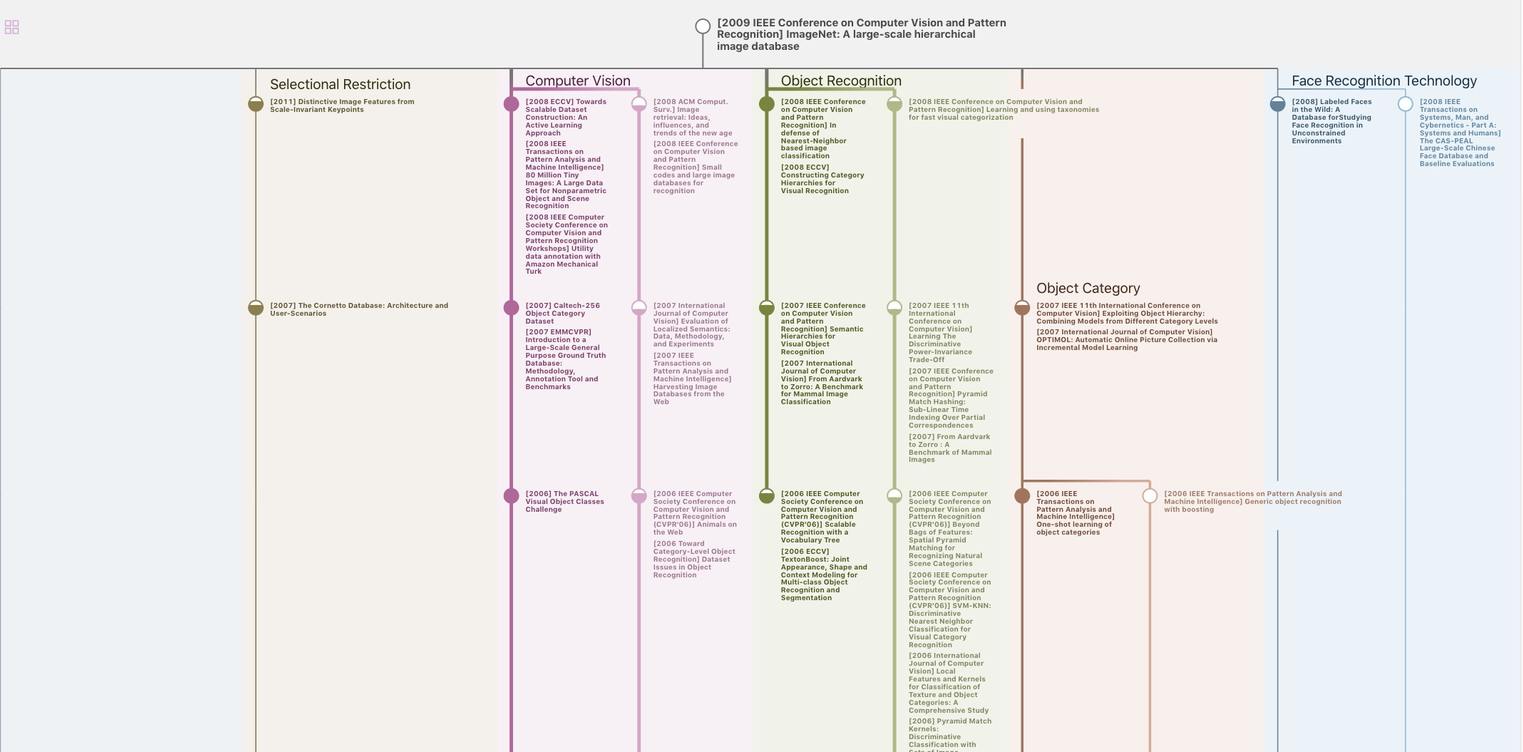
生成溯源树,研究论文发展脉络
Chat Paper
正在生成论文摘要