Towards Robust Few-shot Point Cloud Semantic Segmentation.
CoRR(2023)
摘要
Few-shot point cloud semantic segmentation aims to train a model to quickly adapt to new unseen classes with only a handful of support set samples. However, the noise-free assumption in the support set can be easily violated in many practical real-world settings. In this paper, we focus on improving the robustness of few-shot point cloud segmentation under the detrimental influence of noisy support sets during testing time. To this end, we first propose a Component-level Clean Noise Separation (CCNS) representation learning to learn discriminative feature representations that separates the clean samples of the target classes from the noisy samples. Leveraging the well separated clean and noisy support samples from our CCNS, we further propose a Multi-scale Degree-based Noise Suppression (MDNS) scheme to remove the noisy shots from the support set. We conduct extensive experiments on various noise settings on two benchmark datasets. Our results show that the combination of CCNS and MDNS significantly improves the performance. Our code is available at https://github.com/Pixie8888/R3DFSSeg.
更多查看译文
关键词
cloud,few-shot
AI 理解论文
溯源树
样例
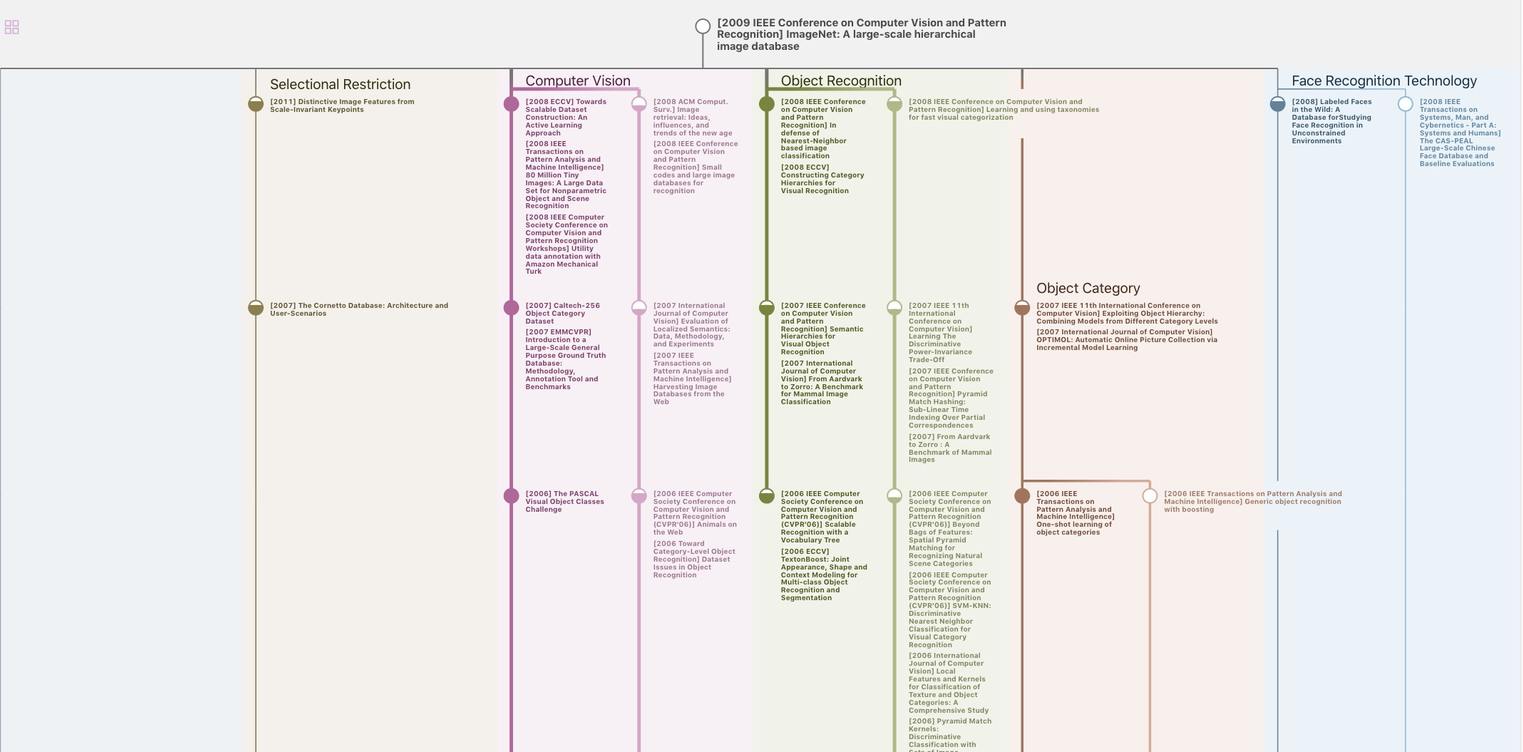
生成溯源树,研究论文发展脉络
Chat Paper
正在生成论文摘要