Conformalized Multimodal Uncertainty Regression and Reasoning
ICASSP 2024 - 2024 IEEE International Conference on Acoustics, Speech and Signal Processing (ICASSP)(2023)
摘要
This paper introduces a lightweight uncertainty estimator capable of predicting multimodal (disjoint) uncertainty bounds by integrating conformal prediction with a deep-learning regressor. We specifically discuss its application for visual odometry (VO), where environmental features such as flying domain symmetries and sensor measurements under ambiguities and occlusion can result in multimodal uncertainties. Our simulation results show that uncertainty estimates in our framework adapt sample-wise against challenging operating conditions such as pronounced noise, limited training data, and limited parametric size of the prediction model. We also develop a reasoning framework that leverages these robust uncertainty estimates and incorporates optical flow-based reasoning to improve prediction prediction accuracy. Thus, by appropriately accounting for predictive uncertainties of data-driven learning and closing their estimation loop via rule-based reasoning, our methodology consistently surpasses conventional deep learning approaches on all these challenging scenarios--pronounced noise, limited training data, and limited model size-reducing the prediction error by 2-3x.
更多查看译文
关键词
Conformal inference,Visual odometry
AI 理解论文
溯源树
样例
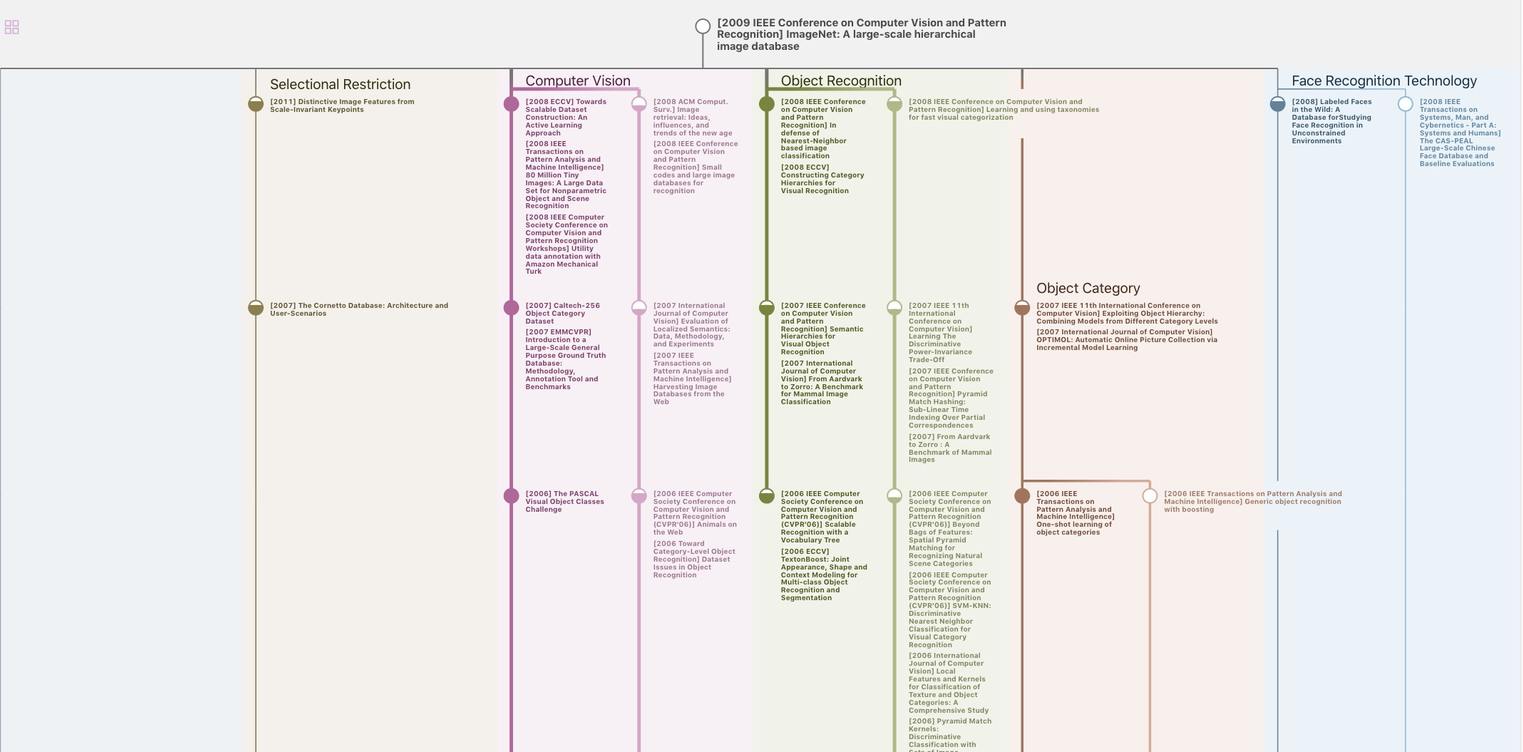
生成溯源树,研究论文发展脉络
Chat Paper
正在生成论文摘要