Change of Scenery: Unsupervised LiDAR Change Detection for Mobile Robots
CoRR(2023)
摘要
This paper presents a fully unsupervised deep change detection approach for mobile robots with 3D LiDAR. In unstructured environments, it is infeasible to define a closed set of semantic classes. Instead, semantic segmentation is reformulated as binary change detection. We develop a neural network, RangeNetCD, that uses an existing point-cloud map and a live LiDAR scan to detect scene changes with respect to the map. Using a novel loss function, existing point-cloud semantic segmentation networks can be trained to perform change detection without any labels or assumptions about local semantics. We demonstrate the performance of this approach on data from challenging terrains; mean intersection over union (mIoU) scores range between 67.4% and 82.2% depending on the amount of environmental structure. This outperforms the geometric baseline used in all experiments. The neural network runs faster than 10Hz and is integrated into a robot's autonomy stack to allow safe navigation around obstacles that intersect the planned path. In addition, a novel method for the rapid automated acquisition of per-point ground-truth labels is described. Covering changed parts of the scene with retroreflective materials and applying a threshold filter to the intensity channel of the LiDAR allows for quantitative evaluation of the change detector.
更多查看译文
关键词
unsupervised lidar change detection,mobile robots,scenery
AI 理解论文
溯源树
样例
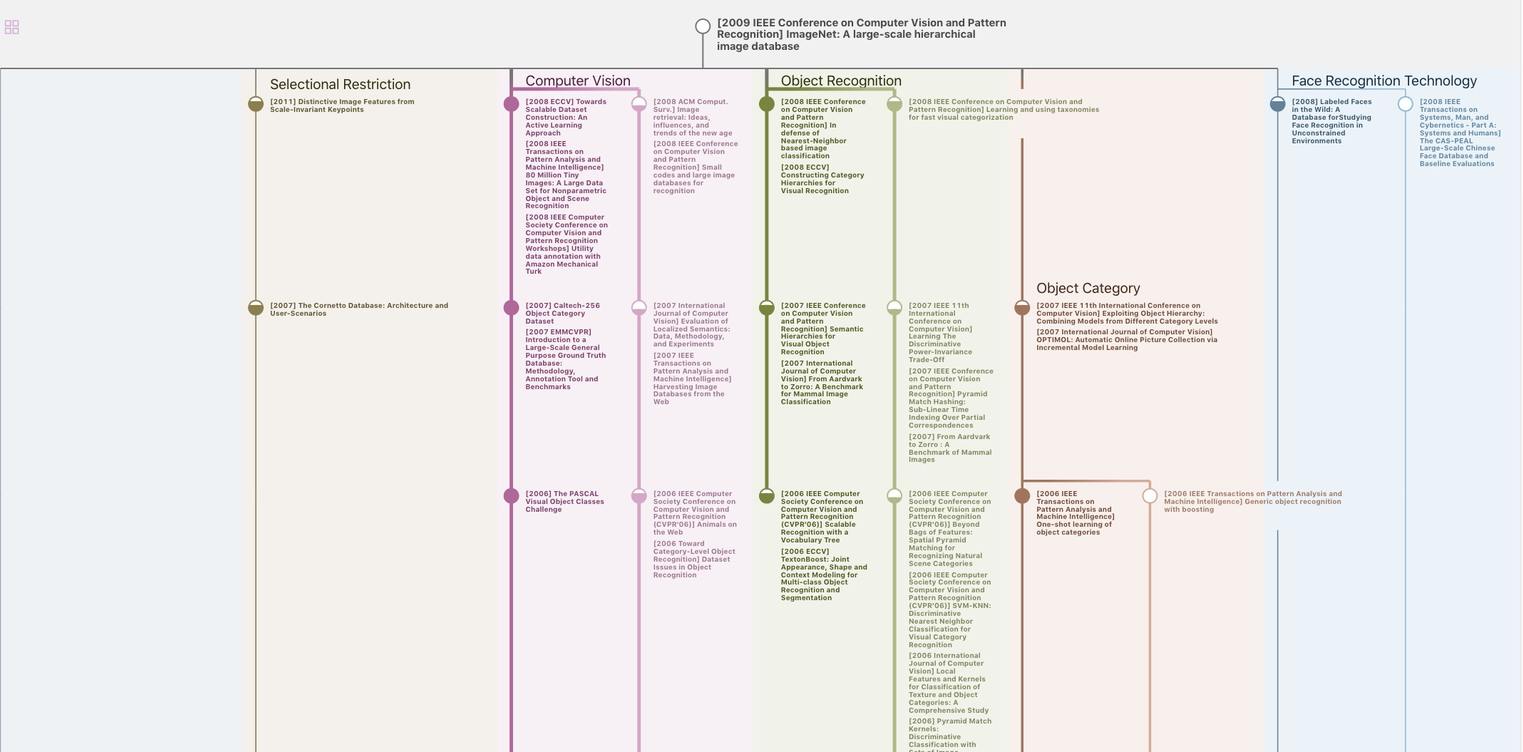
生成溯源树,研究论文发展脉络
Chat Paper
正在生成论文摘要