Actively Learning Reinforcement Learning: A Stochastic Optimal Control Approach
CoRR(2023)
摘要
In this paper we propose a framework towards achieving two intertwined
objectives: (i) equipping reinforcement learning with active exploration and
deliberate information gathering, such that it regulates state and parameter
uncertainties resulting from modeling mismatches and noisy sensory; and (ii)
overcoming the huge computational cost of stochastic optimal control. We
approach both objectives by using reinforcement learning to attain the
stochastic optimal control law. On one hand, we avoid the curse of
dimensionality prohibiting the direct solution of the stochastic dynamic
programming equation. On the other hand, the resulting stochastic control
inspired reinforcement learning agent admits the behavior of a dual control,
namely, caution and probing, that is, regulating the state estimate together
with its estimation quality. Unlike exploration and exploitation, caution and
probing are employed automatically by the controller in real-time, even after
the learning process is concluded. We use the proposed approach on a numerical
example of a model that belongs to an emerging class in system identification.
We show how, for the dimensionality of the stochastic version of this model,
Dynamic Programming is prohibitive, Model Predictive Control requires an
expensive nonlinear optimization, and a Linear Quadratic Regulator with the
certainty equivalence assumption leads to poor performance and filter
divergence, all contrasting our approach which is shown to be both:
computationally convenient, stabilizing and of an acceptable performance.
更多查看译文
关键词
stochastic optimal control
AI 理解论文
溯源树
样例
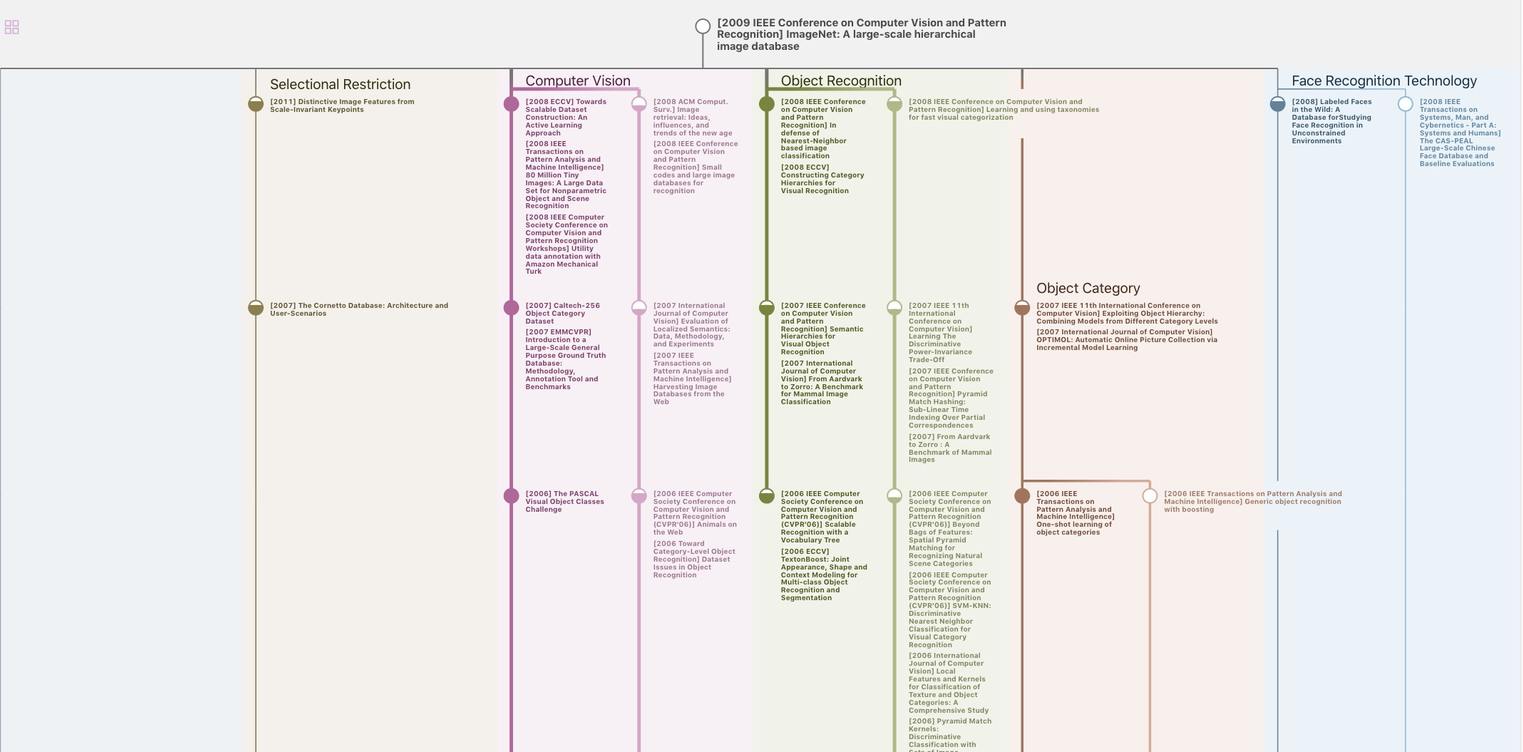
生成溯源树,研究论文发展脉络
Chat Paper
正在生成论文摘要