Evolutionary Interest Representation Network for Click-Through Rate Prediction
2023 IEEE International Conference on Web Services (ICWS)(2023)
摘要
The rapid development of the Internet has revo-lutionized our lives, providing us with an array of convenient services. However, this revolution has also led to the problem of data overload. Personalized recommendation services have been widely recognized as an effective solution to this problem by both the industrial and academic communities. Accurate prediction of click-through rate (CTR) is a critical task in personalized recommendation systems since it can improve the user’s shopping experience, ultimately increasing revenue for the platform. To achieve accurate CTR prediction, it is crucial to capture user interests. While several methods for interest modeling exist, challenges related to user interest evolution and user behavior noise need further attention. This paper proposes a novel CTR prediction model named the Evolutionary Interest Representation Network (EIRN), which learns the evolving interest representation of users from their behaviors, profiles, and environmental attributes. In the model, we introduce an advertisement correlation graph decomposition layer and a noise filter layer to enhance and purify the user behavior representation. An interest extraction layer is designed to capture sequential correlations and common characteristics between user behaviors, representing their interests. Additionally, as user interests evolve over time and their importance changes, an attention-based interest evolution layer is designed to track the evolution of user interests. Our proposed method is validated using four public datasets and an industrial dataset, showing its superiority over existing CTR prediction methods.
更多查看译文
关键词
internet advertising,click-through rate prediction,user behavior,representation enhancement,filter
AI 理解论文
溯源树
样例
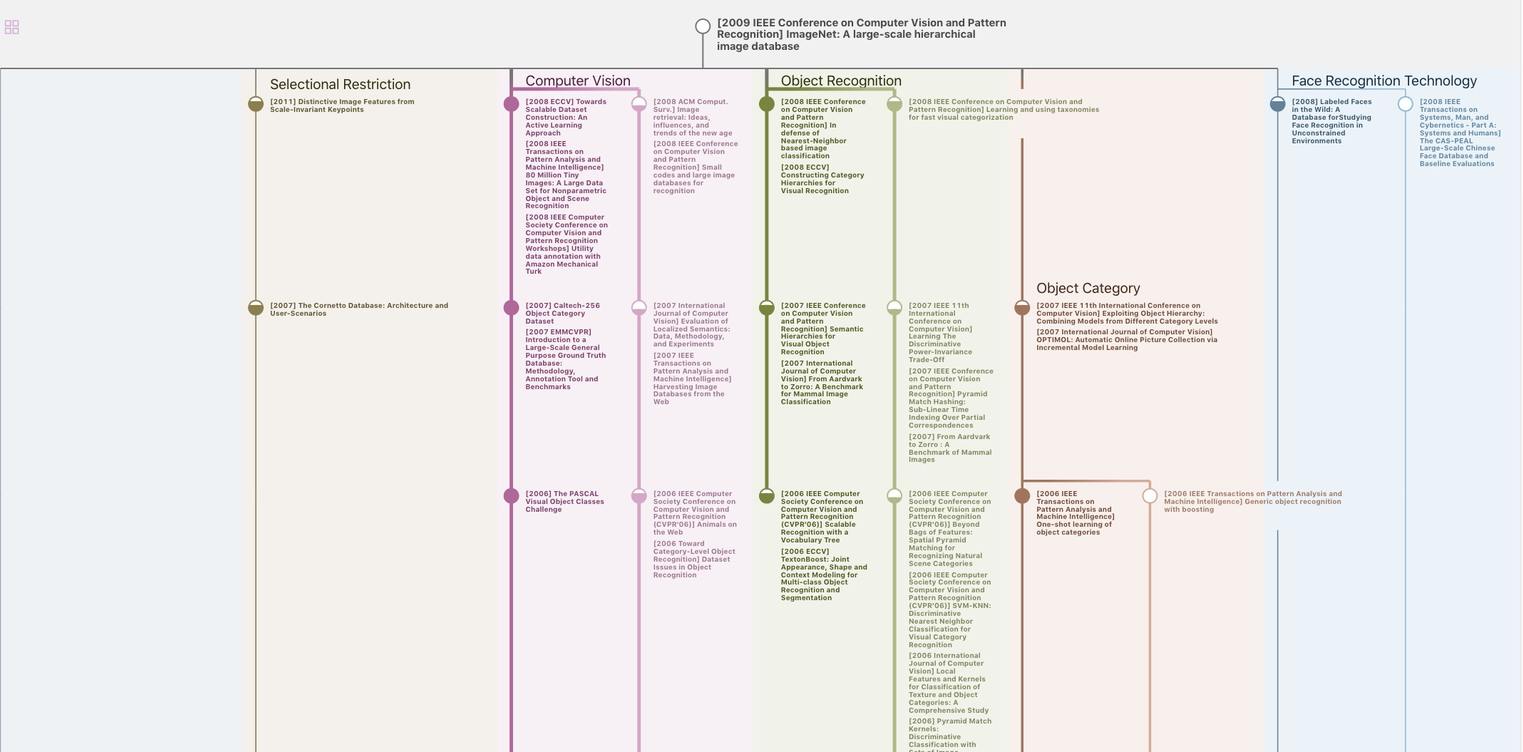
生成溯源树,研究论文发展脉络
Chat Paper
正在生成论文摘要