Robot Skill Generalization: Feature-Selected Adaptation Transfer for Peg-in-Hole Assembly
IEEE TRANSACTIONS ON INDUSTRIAL ELECTRONICS(2024)
摘要
Skill generalization across different tasks is currently a challenging task for robots. As for recent works based on robot learning, substantial environmental interaction costs or abundant expert data are usually needed, thus causing great harm to the robot or the operating object. In this article, feature-selected adaptation transfer is proposed, aiming at accelerating the network learning process, and reducing the harm caused by the interaction process. Based on the domain adaptation, the source domain data with small maximum mean discrepancy to the target domain are extracted to pretrain the target domain policy. By extracting the shared features of the source domain and the target domain, the knowledge transfer between old task and new task is realized. Moreover, the data, more favorable to the target domain, are selected to update the network and further improve the stability of network training. Besides, a series of peg-in-hole tasks is conducted in simulation, and they can be transferred to the real world without directly interacting with the environment.
更多查看译文
关键词
Domain adaptation,feature selected,knowledge transfer,peg-in-hole,skill generalization
AI 理解论文
溯源树
样例
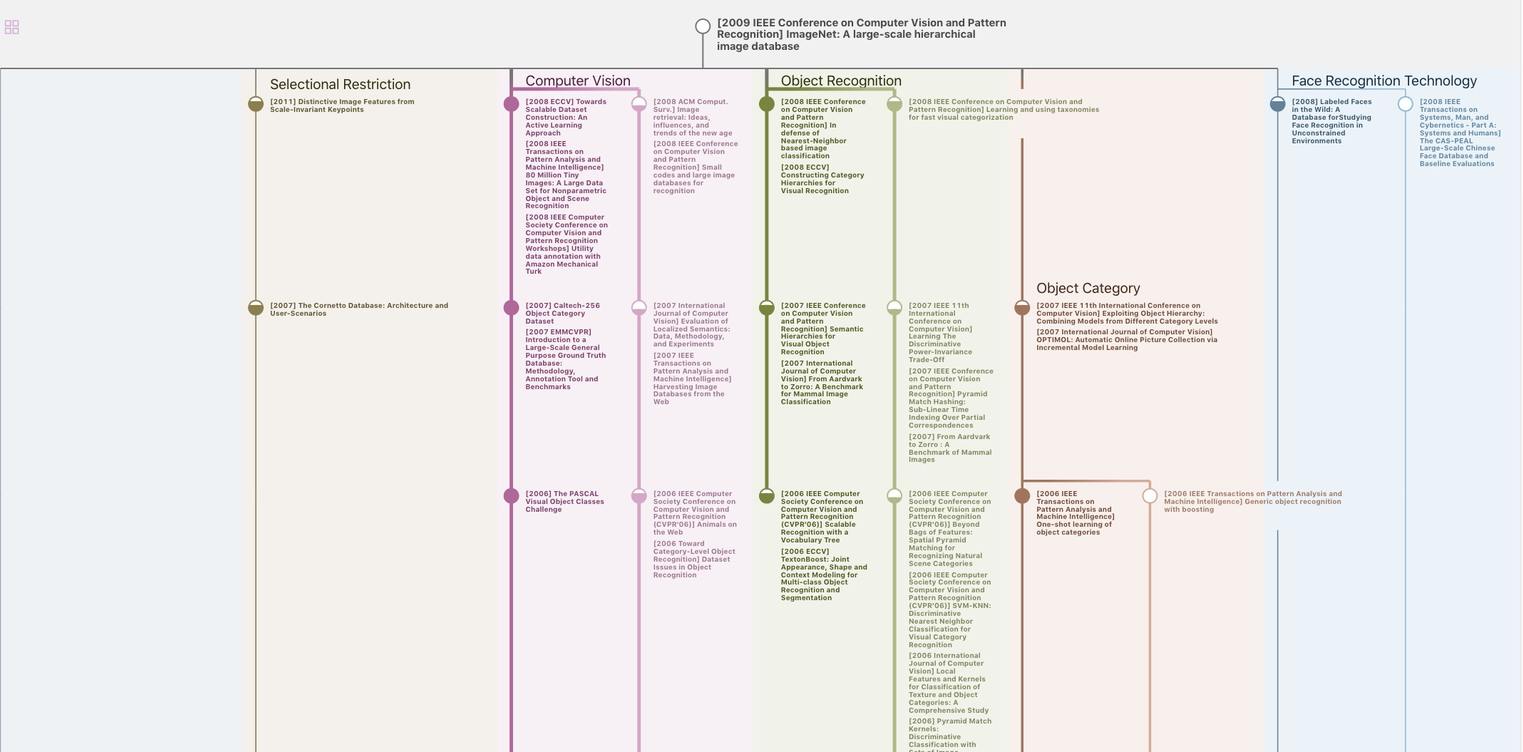
生成溯源树,研究论文发展脉络
Chat Paper
正在生成论文摘要