Independence by Competition: Computational Social Science in the Age of Data Brokers (Preprint)
crossref(2023)
摘要
UNSTRUCTURED When Reddit and Twitter announced they are shutting down their application programming interfaces, the ability for computational social scientists to research online human behavior was severely impacted. But this has been a long time coming. Unlike ten years ago, the social media landscape stretches beyond Facebook and Twitter to TikTok, SnapChat, and Instagram. Parent companies often have divergent views of data use and API access. Moreover, we don’t expect financial institutions to hand out anonymized data, why would we (legally) expect the same from tech companies? While sharing data was an unspoken agreement, this was more business ethos than legal obligation. The recent Meta meta-studies on the 2020 Presidential Elections present a grim view of access (Wagner, 2023). Meta reached out to select researchers to pilot a new paradigm of social science research– Meta provided the data, and two leads picked researchers to conduct an independent analysis. Four years later, over 15 studies have been produced from these data. Though an incredible feat of science, this model of “independence by permission” where select researchers conduct research has called into scrutiny paradigms for online research. A growing trend may provide an alternative. Media Intelligence is the growing industry of collecting and analyzing billions of online conversations to drive business decisions. For researchers, this presents an opportunity. Over the past three years, our team based at the University of Southern California tracked influencers who post about e-cigarette products across multiple platforms, including TikTok, Instagram, and YouTube. We began working completely in-house then transitioned to collaboration with media intelligence companies. Our project measures the portrayal of e-cigarette products on social media and their impact on adolescent tobacco use. It includes multiple components– training deep learning models to identify the age, gender, and e-cigarette; conducting social network analysis; surveying adolescents about their exposure to tobacco. The biggest hurdle for our work is the very first step– data acquisition. Once the Instagram API retired, it was very difficult to scrape posts, captions, comments, and metadata. That’s when we chanced upon Meltwater, the first media intelligence company. Already, they provided enough data to publish profiling influencers. However, this merely provided a starting point. Once we identified the influencers, we proceeded to scrape their posts and social networks. By relying on pre-identified influencers, we skipped the resource-extensive task of scouring the platforms to find the most influential users. One weakness to this approach lies in the proprietary nature of commercial metrics. We do not know the parameters Klear uses, which may have downstream implications for replicability. We assuaged this with manual audits on each suggested profile. If these services can provide an explanation of their method, this would lend to greater transparency. As influencer marketing tactics mature and API access declines, the capabilities of data brokers will grow. What matters is leveraging targeted services as stepping stones toward answering meaningful research questions. The days of free data may be over, but researchers may find a reasonable substitute via “independence by competition” in the market of data brokers.
更多查看译文
AI 理解论文
溯源树
样例
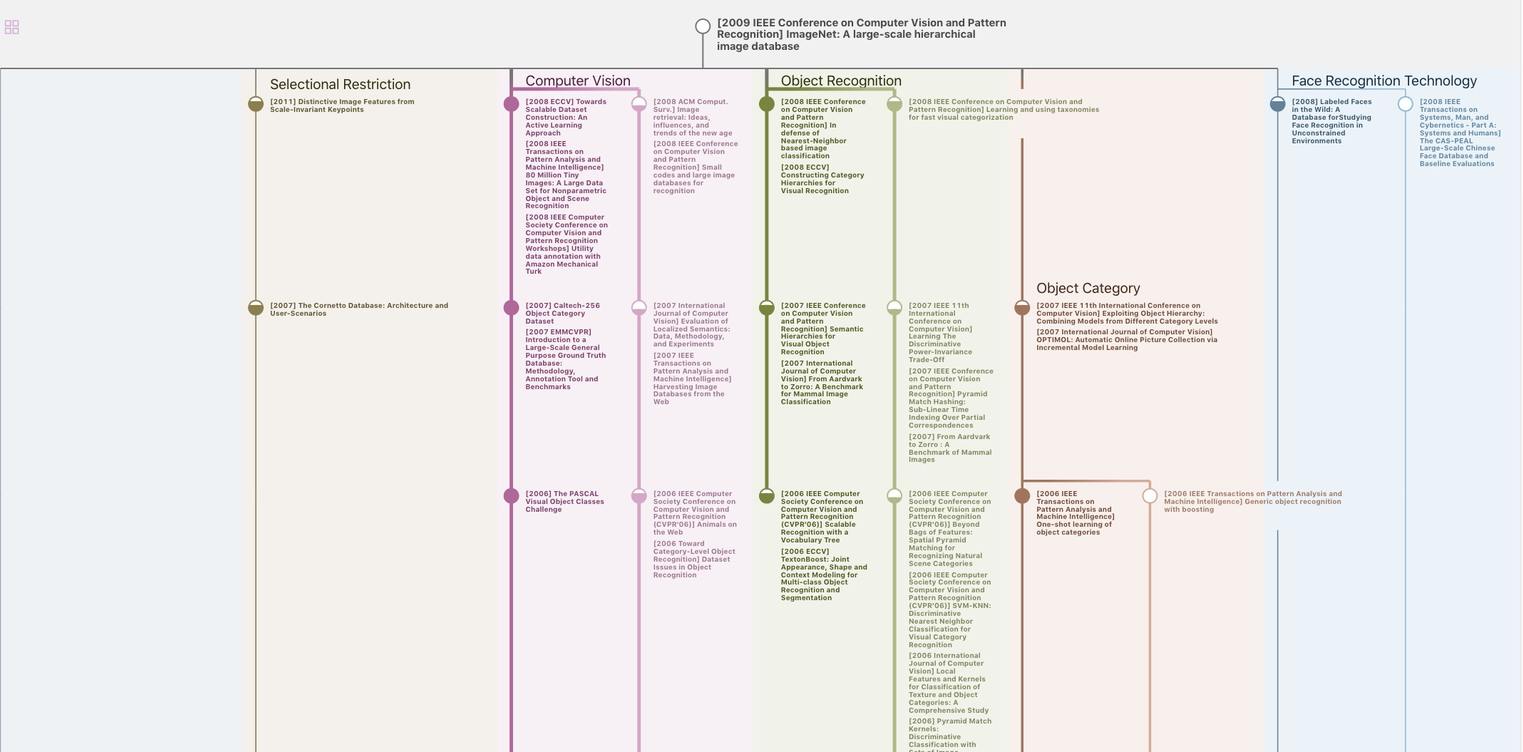
生成溯源树,研究论文发展脉络
Chat Paper
正在生成论文摘要