Raman spectrum model transfer method based on Cycle-GAN
SPECTROCHIMICA ACTA PART A-MOLECULAR AND BIOMOLECULAR SPECTROSCOPY(2024)
摘要
The disparity in hardware quality among various models of Raman spectrometers gives rise to variations in the acquired Raman spectral data, even when the same substance is collected under identical external conditions. Conventionally, models constructed using data obtained from a particular instrument exhibit issues such as limited applicability or poor performance when deployed to different instruments. Currently, numerous model transfer algorithms grounded in chemometrics have been developed, all aiming to establish a mapping relationship capable of transforming spectral data from the source domain to the target domain. With the advancement of deep learning techniques, the utilization of deep learning enables the effective resolution of nonlinear mapping relationships between two spectral vectors. In the field of image translation, the CycleConsistent Adversarial Networks, Cycle-GAN, has already achieved mutual transformation between two distinct style images. However, due to images being multidimensional matrix data, unlike one-dimensional spectral data vectors, we have constructed a deep learning network based on Cycle-GAN for vector-to-vector transformation. This network allows the direct conversion of spectral data from the source domain to the target domain, without requiring parameter adjustments or other operations. Compared with traditional chemometric methods, our method is more intelligent and efficient. Finally, the cosine similarity between the source domain data and the transformed target domain data exceeds 99%.
更多查看译文
关键词
Raman spectroscopy,Chemometrics,Model transfer,Mapping relationship,Deep learning
AI 理解论文
溯源树
样例
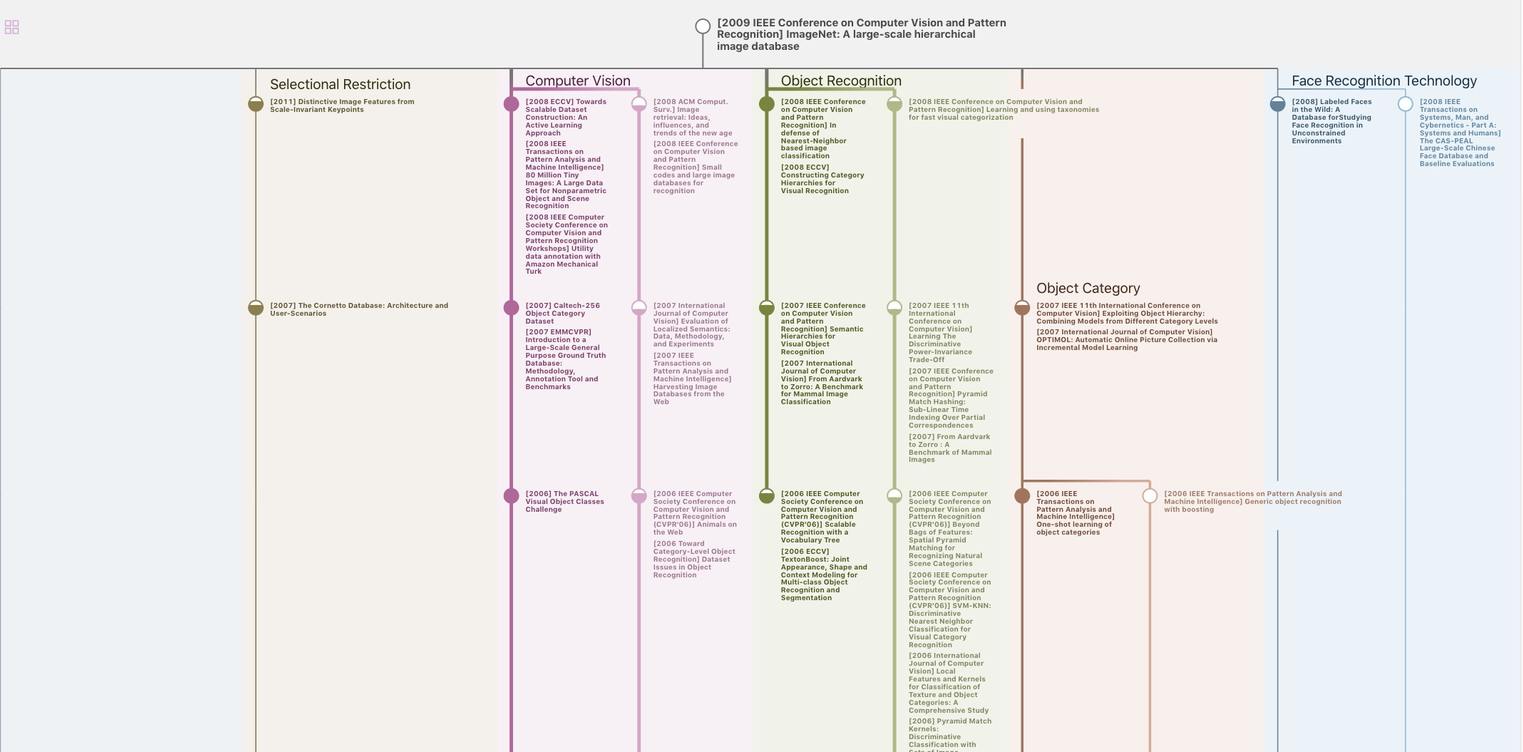
生成溯源树,研究论文发展脉络
Chat Paper
正在生成论文摘要