A Multi Constrained Transformer-BiLSTM Guided Network for Automated Sleep Stage Classification from Single-Channel EEG
arXiv (Cornell University)(2023)
摘要
Sleep stage classification from electroencephalogram (EEG) is significant for the rapid evaluation of sleeping patterns and quality. A novel deep learning architecture, ``DenseRTSleep-II'', is proposed for automatic sleep scoring from single-channel EEG signals. The architecture utilizes the advantages of Convolutional Neural Network (CNN), transformer network, and Bidirectional Long Short Term Memory (BiLSTM) for effective sleep scoring. Moreover, with the addition of a weighted multi-loss scheme, this model is trained more implicitly for vigorous decision-making tasks. Thus, the model generates the most efficient result in the SleepEDFx dataset and outperforms different state-of-the-art (IIT-Net, DeepSleepNet) techniques by a large margin in terms of accuracy, precision, and F1-score.
更多查看译文
关键词
sleep stage classification,eeg,transformer-bilstm,single-channel
AI 理解论文
溯源树
样例
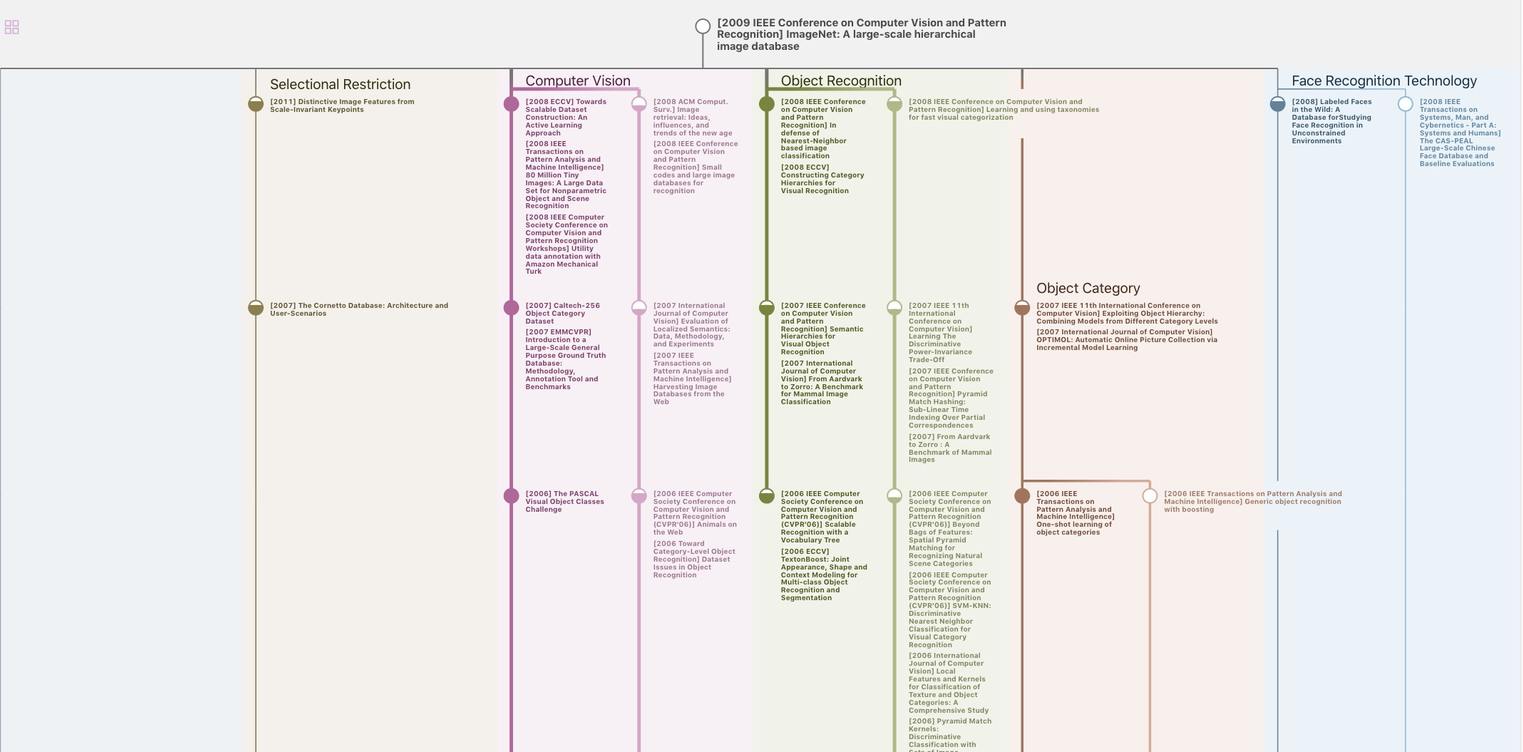
生成溯源树,研究论文发展脉络
Chat Paper
正在生成论文摘要