Unsupervised Landmark Discovery Using Consistency Guided Bottleneck
CoRR(2023)
摘要
We study a challenging problem of unsupervised discovery of object landmarks. Many recent methods rely on bottlenecks to generate 2D Gaussian heatmaps however, these are limited in generating informed heatmaps while training, presumably due to the lack of effective structural cues. Also, it is assumed that all predicted landmarks are semantically relevant despite having no ground truth supervision. In the current work, we introduce a consistency-guided bottleneck in an image reconstruction-based pipeline that leverages landmark consistency, a measure of compatibility score with the pseudo-ground truth to generate adaptive heatmaps. We propose obtaining pseudo-supervision via forming landmark correspondence across images. The consistency then modulates the uncertainty of the discovered landmarks in the generation of adaptive heatmaps which rank consistent landmarks above their noisy counterparts, providing effective structural information for improved robustness. Evaluations on five diverse datasets including MAFL, AFLW, LS3D, Cats, and Shoes demonstrate excellent performance of the proposed approach compared to the existing state-of-the-art methods. Our code is publicly available at https://github.com/MamonaAwan/CGB_ULD.
更多查看译文
关键词
unsupervised landmark discovery,consistency guided bottleneck
AI 理解论文
溯源树
样例
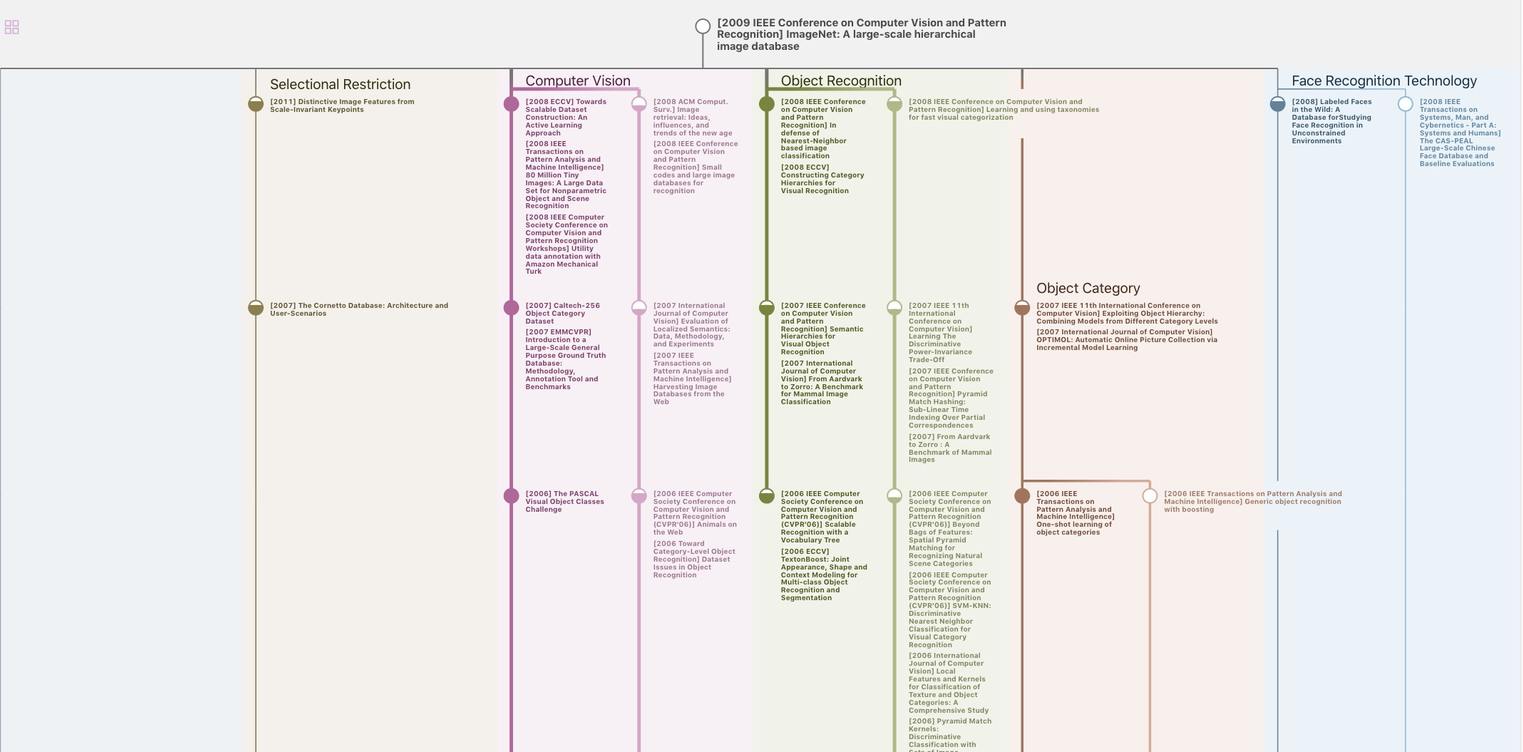
生成溯源树,研究论文发展脉络
Chat Paper
正在生成论文摘要