Predicate Classification Using Optimal Transport Loss in Scene Graph Generation
CoRR(2023)
摘要
In scene graph generation (SGG), learning with cross-entropy loss yields biased predictions owing to the severe imbalance in the distribution of the relationship labels in the dataset. Thus, this study proposes a method to generate scene graphs using optimal transport as a measure for comparing two probability distributions. We apply learning with the optimal transport loss, which reflects the similarity between the labels in terms of transportation cost, for predicate classification in SGG. In the proposed approach, the transportation cost of the optimal transport is defined using the similarity of words obtained from the pre-trained model. The experimental evaluation of the effectiveness demonstrates that the proposed method outperforms existing methods in terms of mean Recall@50 and 100. Furthermore, it improves the recall of the relationship labels scarcely available in the dataset.
更多查看译文
关键词
scene graph
AI 理解论文
溯源树
样例
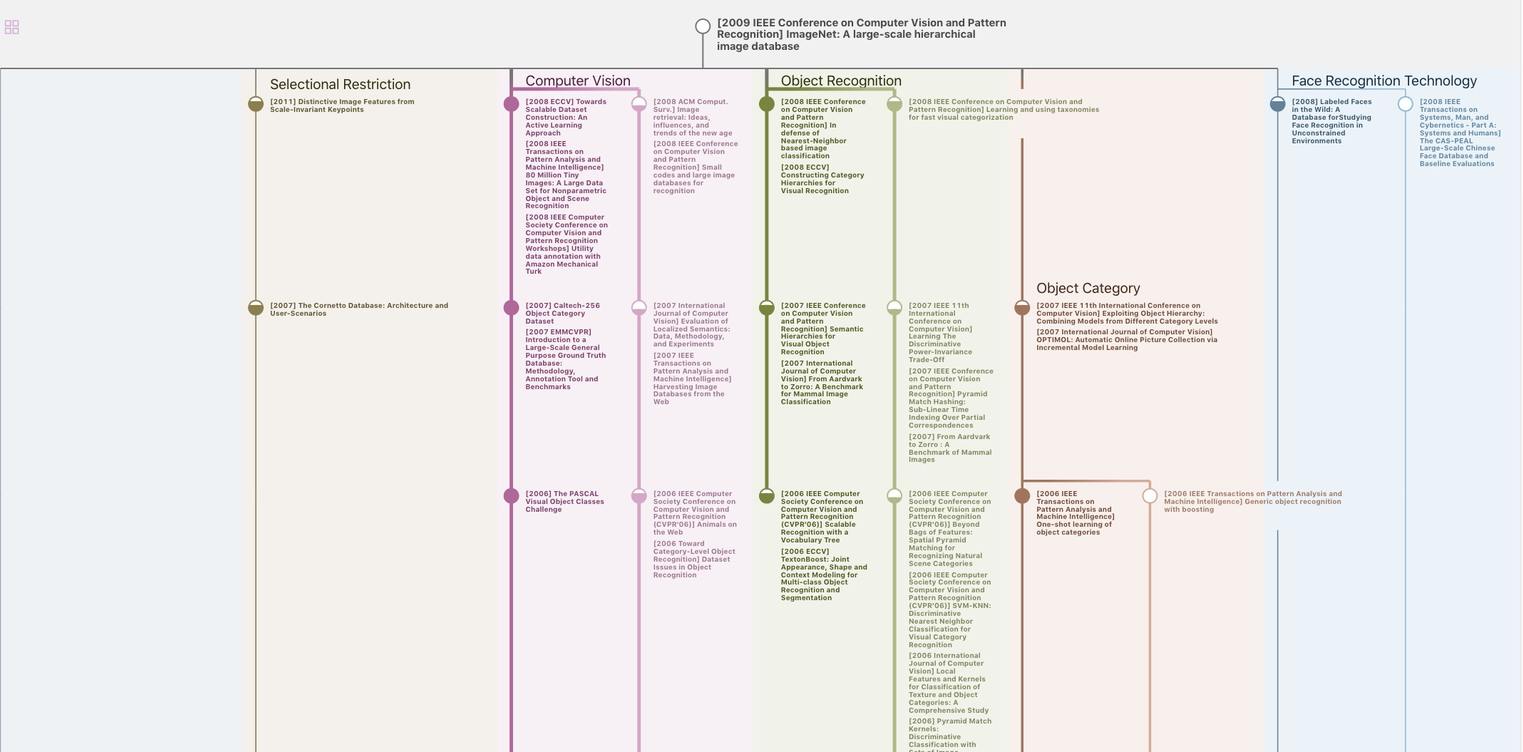
生成溯源树,研究论文发展脉络
Chat Paper
正在生成论文摘要