Graph Neural Networks for Dynamic Modeling of Roller Bearing
CoRR(2023)
摘要
In the presented work, we propose to apply the framework of graph neural networks (GNNs) to predict the dynamics of a rolling element bearing. This approach offers generalizability and interpretability, having the potential for scalable use in real-time operational digital twin systems for monitoring the health state of rotating machines. By representing the bearing's components as nodes in a graph, the GNN can effectively model the complex relationships and interactions among them. We utilize a dynamic spring-mass-damper model of a bearing to generate the training data for the GNN. In this model, discrete masses represent bearing components such as rolling elements, inner raceways, and outer raceways, while a Hertzian contact model is employed to calculate the forces between these components. We evaluate the learning and generalization capabilities of the proposed GNN framework by testing different bearing configurations that deviate from the training configurations. Through this approach, we demonstrate the effectiveness of the GNN-based method in accurately predicting the dynamics of rolling element bearings, highlighting its potential for real-time health monitoring of rotating machinery.
更多查看译文
关键词
graph neural networks,neural networks,bearing,dynamic modeling
AI 理解论文
溯源树
样例
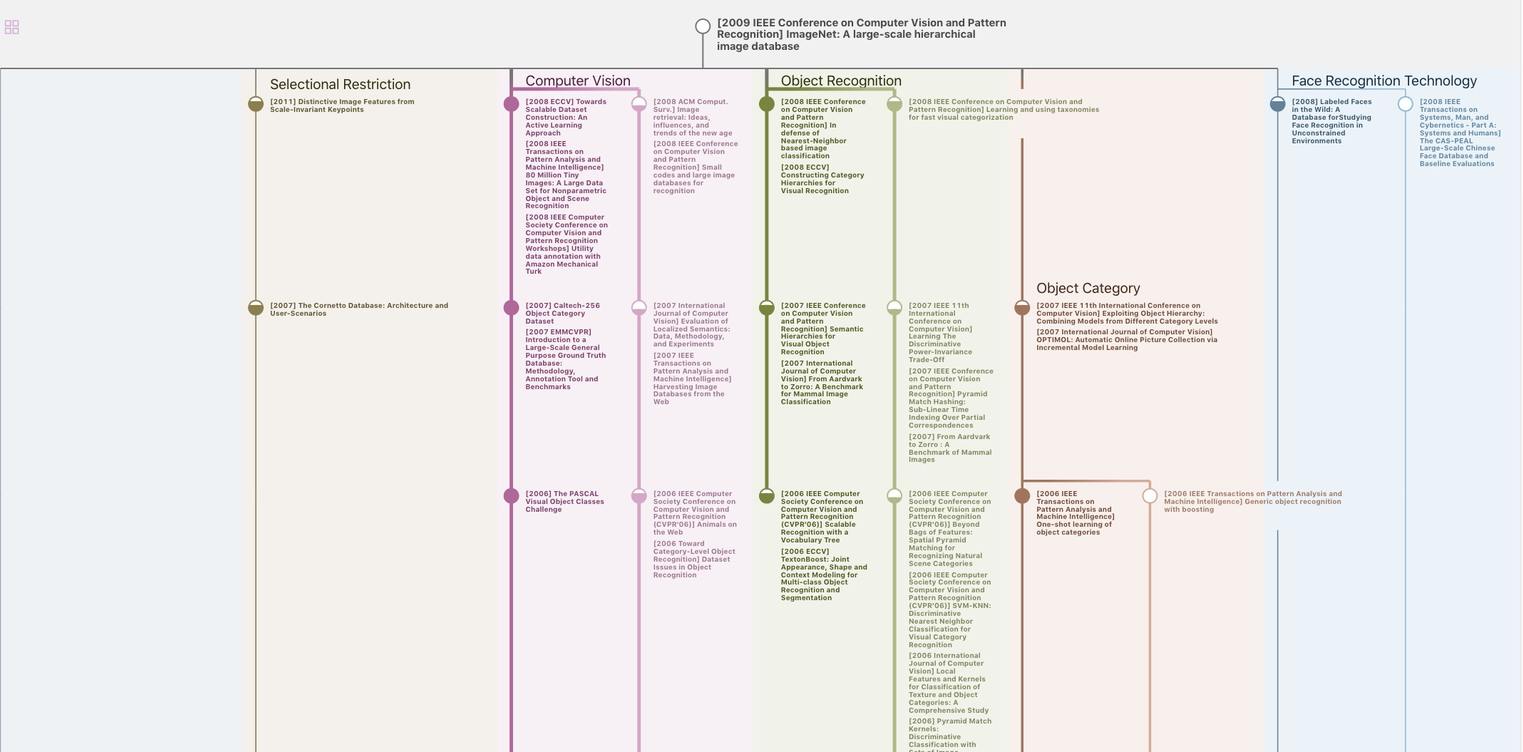
生成溯源树,研究论文发展脉络
Chat Paper
正在生成论文摘要