Improving Speech Recognition for African American English With Audio Classification
ICASSP 2024 - 2024 IEEE International Conference on Acoustics, Speech and Signal Processing (ICASSP)(2023)
摘要
Automatic speech recognition (ASR) systems have been shown to have large quality disparities between the language varieties they are intended or expected to recognize. One way to mitigate this is to train or fine-tune models with more representative datasets. But this approach can be hindered by limited in-domain data for training and evaluation. We propose a new way to improve the robustness of a US English short-form speech recognizer using a small amount of out-of-domain (long-form) African American English (AAE) data. We use CORAAL, YouTube and Mozilla Common Voice to train an audio classifier to approximately output whether an utterance is AAE or some other variety including Mainstream American English (MAE). By combining the classifier output with coarse geographic information, we can select a subset of utterances from a large corpus of untranscribed short-form queries for semi-supervised learning at scale. Fine-tuning on this data results in a 38.5% relative word error rate disparity reduction between AAE and MAE without reducing MAE quality.
更多查看译文
关键词
US English,African American English,dialect classifier,equity,automatic speech recognition
AI 理解论文
溯源树
样例
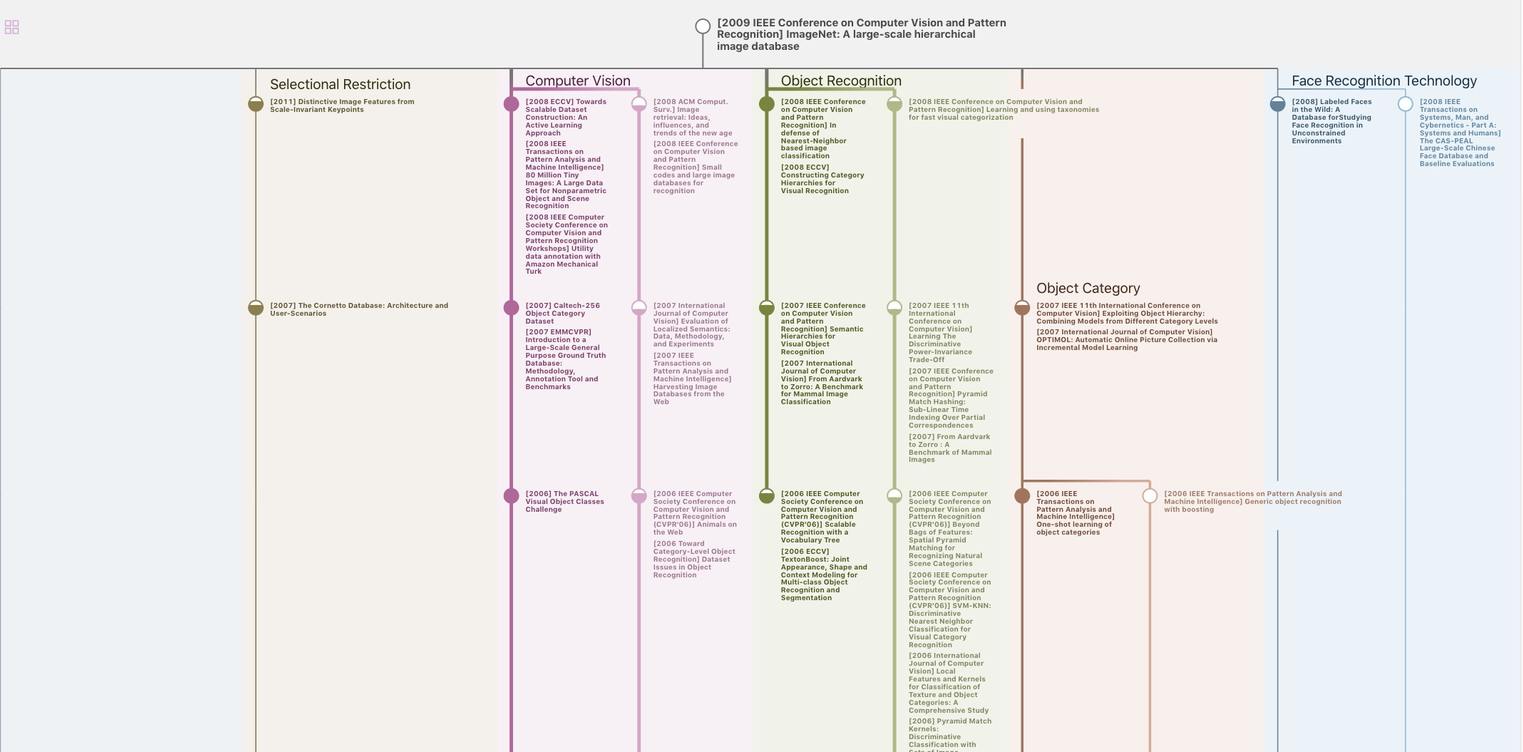
生成溯源树,研究论文发展脉络
Chat Paper
正在生成论文摘要