SPANet: A Self-Balancing Position Attention Network for Anchor-Free SAR Ship Detection
IEEE Journal of Selected Topics in Applied Earth Observations and Remote Sensing(2023)
摘要
Synthetic aperture radar (SAR) images of ships have complex background interference, multi-scale targets, and irregular distribution characteristics. However, existing mainstream SAR ship detection algorithms rely on manually designed hyperparameters. This results in poor robustness, which makes it difficult to effectively balance the detection accuracy and speed. To solve these problems, a novel anchor-free SAR ship detection algorithm based on self-balancing position attention (SBPA) is proposed. First, a lightweight feature extraction backbone (GhostVS-Net) is designed by FOCUS, ghost, and separable convolution modules to extract feature information. This helps to extract the contour features of ships and suppress unrelated interference, making it more suitable for the scattering characteristics of SAR images. Second, an SBPA module is designed, which balances the local image features under multiple receptive fields, and aggregates the global position information and spatial context information. The proposed SBPA module considers the characteristics of background, scale, and distribution of SAR images to obtain greatly improved positioning accuracy. Finally, the feature pyramid network is applied to fuse the scale context information, and further improve the accuracy of detection. Experimental results on the SSDD and HRSID datasets show that the proposed network bears feature of accurate detection and strong robustness, with the mean average precision reaches 99.72% and 95.30%, which reveals that it outperforms all current state-of-the-art algorithms with super high performances.
更多查看译文
关键词
Feature extraction, Marine vehicles, Radar polarimetry, Convolution, Detection algorithms, Prediction algorithms, Signal processing algorithms, Anchor-free mechanism, self-attention, small target detection, synthetic aperture radar (SAR)
AI 理解论文
溯源树
样例
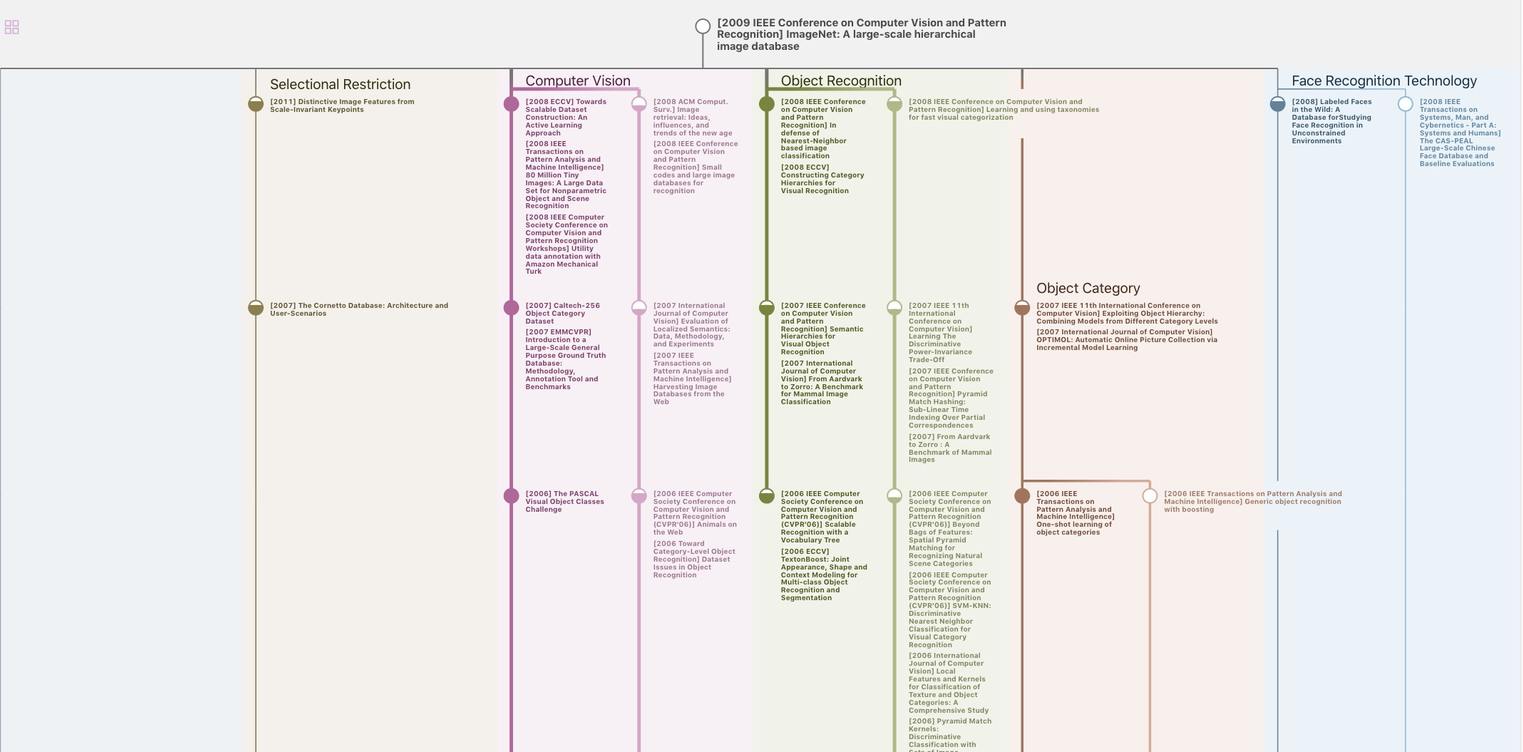
生成溯源树,研究论文发展脉络
Chat Paper
正在生成论文摘要