Neural Quantized Obstacle Avoidance Control for Underactuated USVs based on Control Barrier Functions
2023 42nd Chinese Control Conference (CCC)(2023)
摘要
Safety-critical control has received extensive attention in mobile robotic systems such as unmanned surface vehicles (USVs), whose main objective is to make the vessels accomplish their desired tasks while avoiding unsafe areas, also known as obstacle avoidance control. In this paper, for a class of underactuated USV systems with collision avoidance requirements, a neural quantized obstacle avoidance tracking control algorithm based on control barrier functions (CBFs) is developed, which is implemented by backstepping technique and can guarantee the semi-globally asymptotically stable of the whole closed-loop system subject to the safety conditions. Moreover, by introducing RBF neural networks and quantizers, the proposed control strategy allows the USV systems to have unknown parameters, unknown nonlinearities and input quantization concurrently. Simulation results demonstrate the efficiency of the presented approach.
更多查看译文
关键词
Safety-critical Control,Control Barrier Functions,RBF Neural Networks,Quantized Control,Unmanned Surface Vehicles
AI 理解论文
溯源树
样例
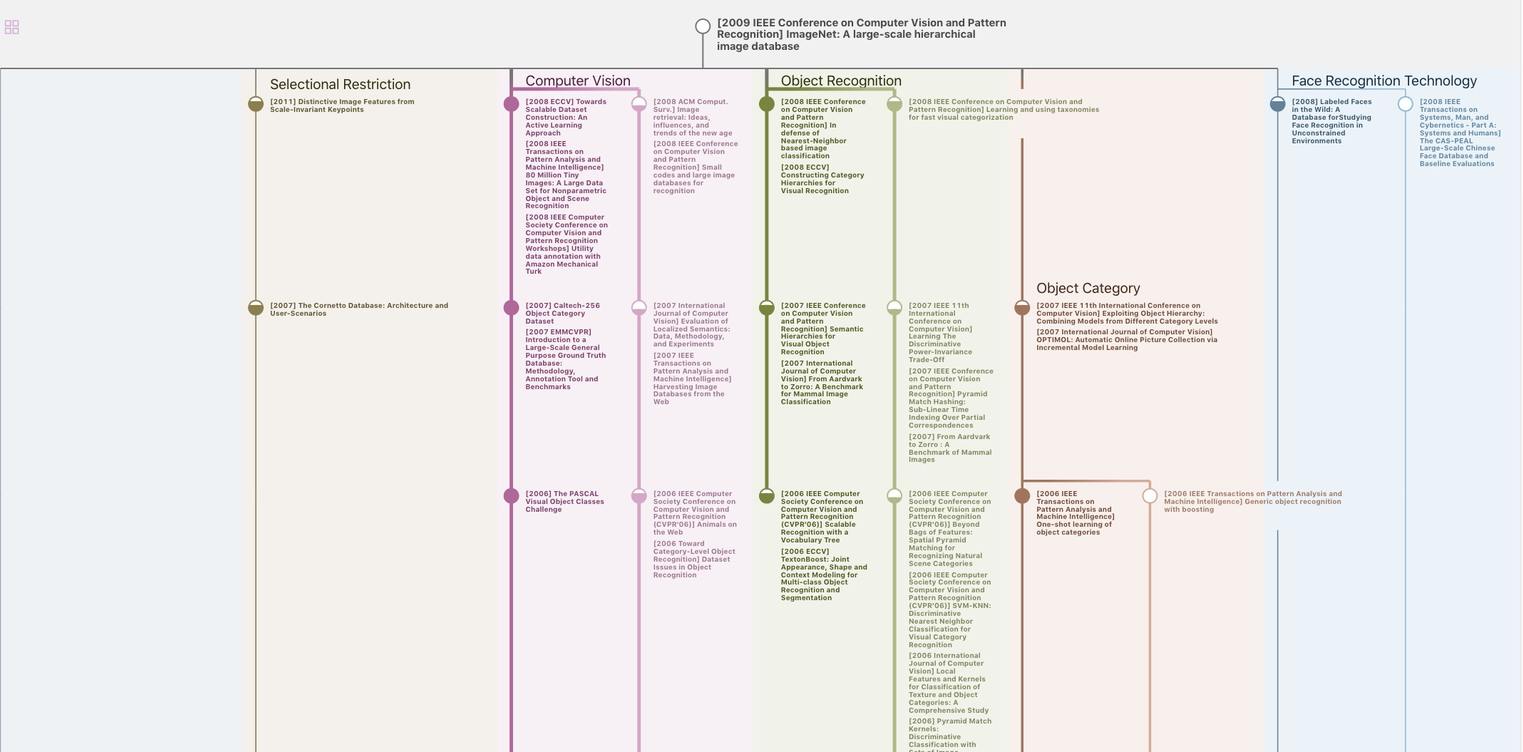
生成溯源树,研究论文发展脉络
Chat Paper
正在生成论文摘要