Development of a novel chemoinformatic tool for natural product databases
Future Drug Discovery(2023)
摘要
Aim: This study aimed to develop a chemoinformatic tool for extracting natural product information from academic literature. Materials & methods: Machine learning graph embeddings were used to extract knowledge from a knowledge graph, connecting properties, molecular data and BERTopic topics. Results: Metapath2Vec performed best in extracting compound names and showed improvement over evaluation stages. Embedding Propagation on Heterogeneous Networks achieved the best performance in extracting bioactivity information. Metapath2Vec excelled in extracting species information, while DeepWalk and Node2Vec performed well in one stage for species location extraction. Embedding Propagation on Heterogeneous Networks consistently improved performance and achieved the best overall scores. Unsupervised embeddings effectively extracted knowledge, with different methods excelling in different scenarios. Conclusion: This research establishes a foundation for frameworks in knowledge extraction, benefiting sustainable resource use.
更多查看译文
关键词
novel chemoinformatic tool,natural product,databases
AI 理解论文
溯源树
样例
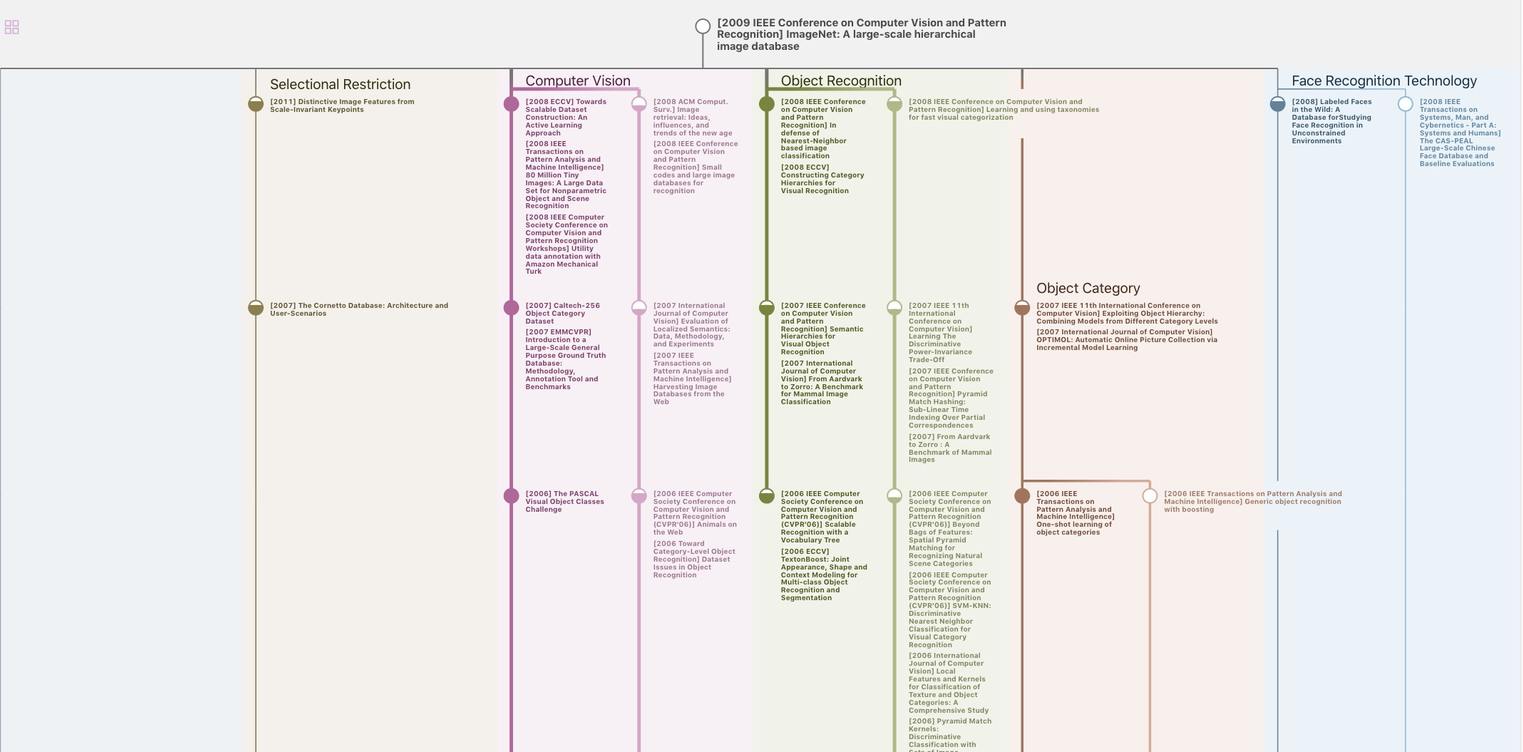
生成溯源树,研究论文发展脉络
Chat Paper
正在生成论文摘要