Investigating End-to-End ASR Architectures for Long Form Audio Transcription
arXiv (Cornell University)(2023)
摘要
This paper presents an overview and evaluation of some of the end-to-end ASR models on long-form audios. We study three categories of Automatic Speech Recognition(ASR) models based on their core architecture: (1) convolutional, (2) convolutional with squeeze-and-excitation and (3) convolutional models with attention. We selected one ASR model from each category and evaluated Word Error Rate, maximum audio length and real-time factor for each model on a variety of long audio benchmarks: Earnings-21 and 22, CORAAL, and TED-LIUM3. The model from the category of self-attention with local attention and global token has the best accuracy comparing to other architectures. We also compared models with CTC and RNNT decoders and showed that CTC-based models are more robust and efficient than RNNT on long form audio.
更多查看译文
关键词
transcription,long form audio,end-to-end
AI 理解论文
溯源树
样例
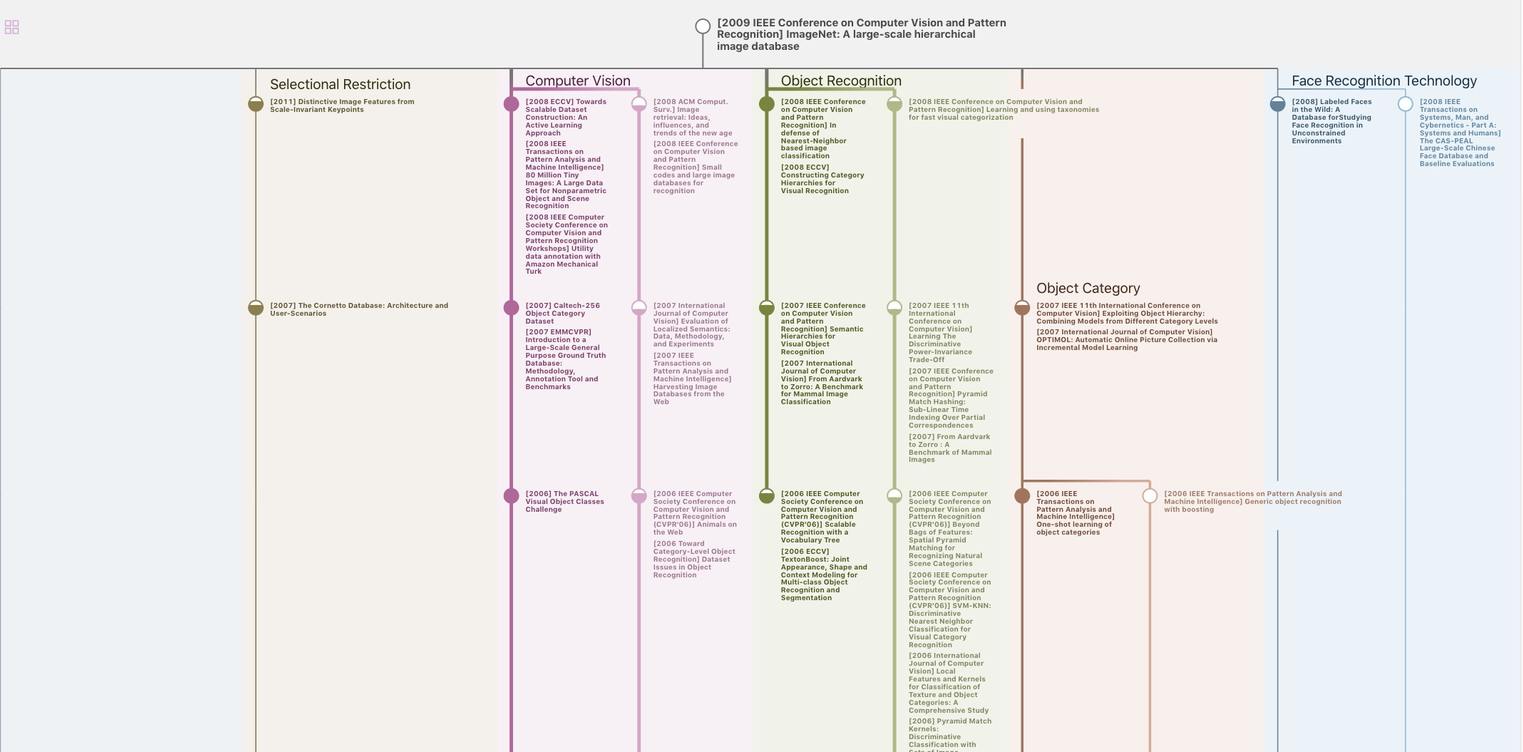
生成溯源树,研究论文发展脉络
Chat Paper
正在生成论文摘要