PanoMixSwap Panorama Mixing via Structural Swapping for Indoor Scene Understanding
CoRR(2023)
摘要
The volume and diversity of training data are critical for modern deep learningbased methods. Compared to the massive amount of labeled perspective images, 360 panoramic images fall short in both volume and diversity. In this paper, we propose PanoMixSwap, a novel data augmentation technique specifically designed for indoor panoramic images. PanoMixSwap explicitly mixes various background styles, foreground furniture, and room layouts from the existing indoor panorama datasets and generates a diverse set of new panoramic images to enrich the datasets. We first decompose each panoramic image into its constituent parts: background style, foreground furniture, and room layout. Then, we generate an augmented image by mixing these three parts from three different images, such as the foreground furniture from one image, the background style from another image, and the room structure from the third image. Our method yields high diversity since there is a cubical increase in image combinations. We also evaluate the effectiveness of PanoMixSwap on two indoor scene understanding tasks: semantic segmentation and layout estimation. Our experiments demonstrate that state-of-the-art methods trained with PanoMixSwap outperform their original setting on both tasks consistently.
更多查看译文
关键词
panorama,indoor scene
AI 理解论文
溯源树
样例
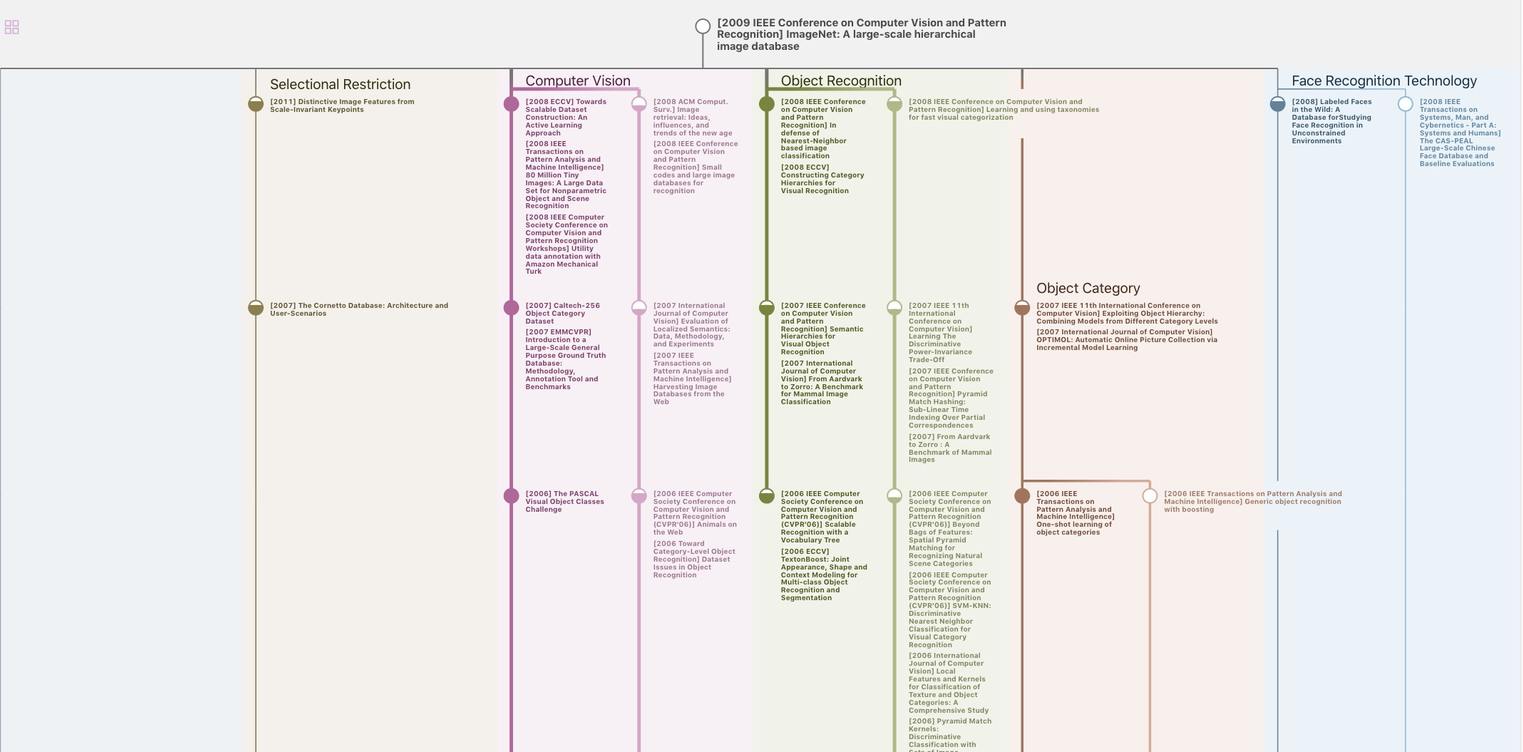
生成溯源树,研究论文发展脉络
Chat Paper
正在生成论文摘要