Kinematics-aware Trajectory Generation and Prediction with Latent Stochastic Differential Modeling
CoRR(2023)
摘要
Trajectory generation and trajectory prediction are two critical tasks for autonomous vehicles, which generate various trajectories during development and predict the trajectories of surrounding vehicles during operation, respectively. However, despite significant advances in improving their performance, it remains a challenging problem to ensure that the generated/predicted trajectories are realistic, explainable, and physically feasible. Existing model-based methods provide explainable results, but are constrained by predefined model structures, limiting their capabilities to address complex scenarios. Conversely, existing deep learning-based methods have shown great promise in learning various traffic scenarios and improving overall performance, but they often act as opaque black boxes and lack explainability. In this work, we integrate kinematic knowledge with neural stochastic differential equations (SDE) and develop a variational autoencoder based on a novel latent kinematics-aware SDE (LK-SDE) to generate vehicle motions. Our approach combines the advantages of both model-based and deep learning-based techniques. Experimental results demonstrate that our method significantly outperforms baseline approaches in producing realistic, physically-feasible, and precisely-controllable vehicle trajectories, benefiting both generation and prediction tasks.
更多查看译文
关键词
stochastic differential modeling,prediction,kinematics-aware
AI 理解论文
溯源树
样例
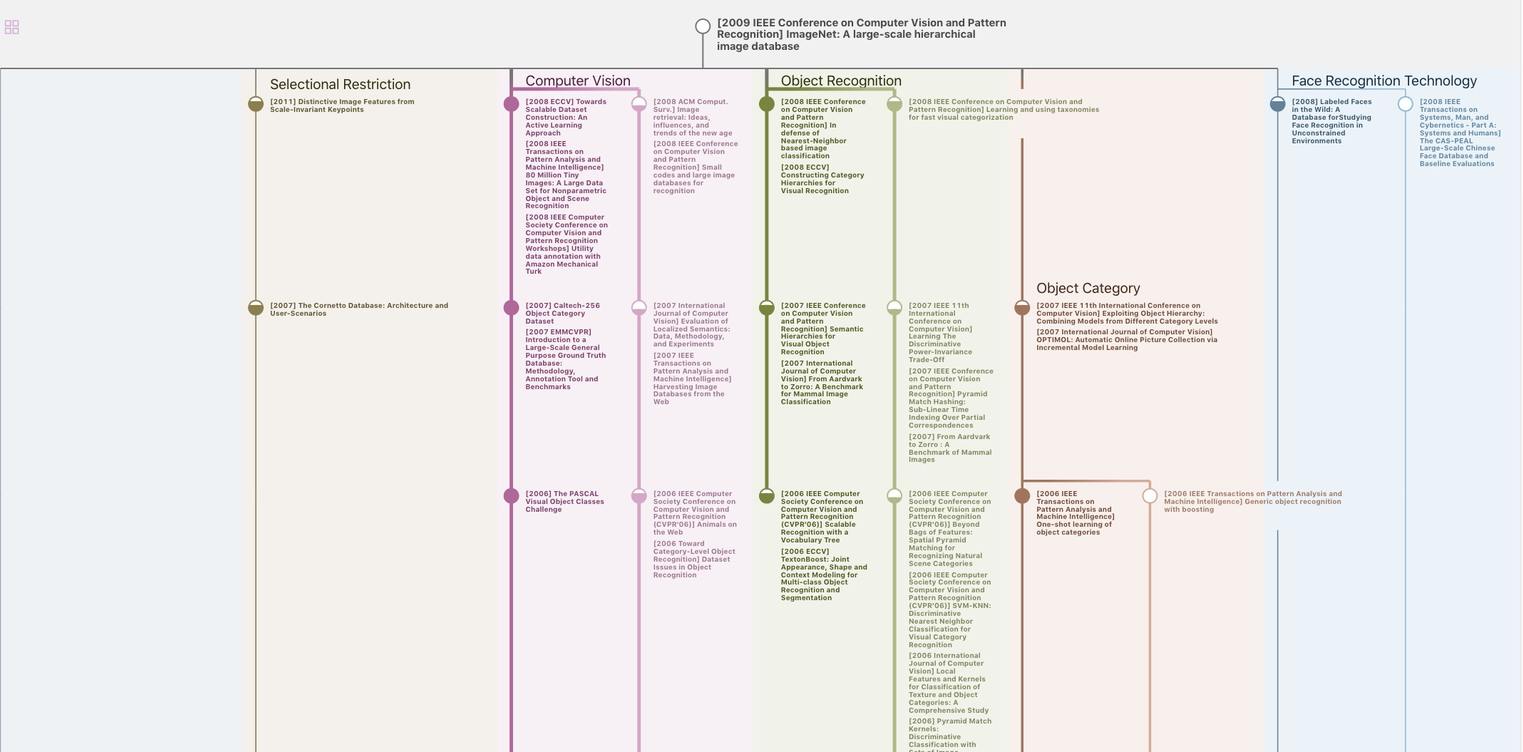
生成溯源树,研究论文发展脉络
Chat Paper
正在生成论文摘要