Self-training Strategies for Sentiment Analysis: An Empirical Study
CoRR(2023)
摘要
Sentiment analysis is a crucial task in natural language processing that
involves identifying and extracting subjective sentiment from text.
Self-training has recently emerged as an economical and efficient technique for
developing sentiment analysis models by leveraging a small amount of labeled
data and a large amount of unlabeled data. However, given a set of training
data, how to utilize them to conduct self-training makes a significant
difference in the final performance of the model. We refer to this methodology
as the self-training strategy. In this paper, we present an empirical study of
various self-training strategies for sentiment analysis. First, we investigate
the influence of the self-training strategy and hyper-parameters on the
performance of traditional small language models (SLMs) in various few-shot
settings. Second, we also explore the feasibility of leveraging large language
models (LLMs) to help self-training. We propose and empirically compare several
self-training strategies with the intervention of LLMs. Extensive experiments
are conducted on three real-world sentiment analysis datasets.
更多查看译文
关键词
instance selection strategies,sentiment analysis,empirical study,self-training
AI 理解论文
溯源树
样例
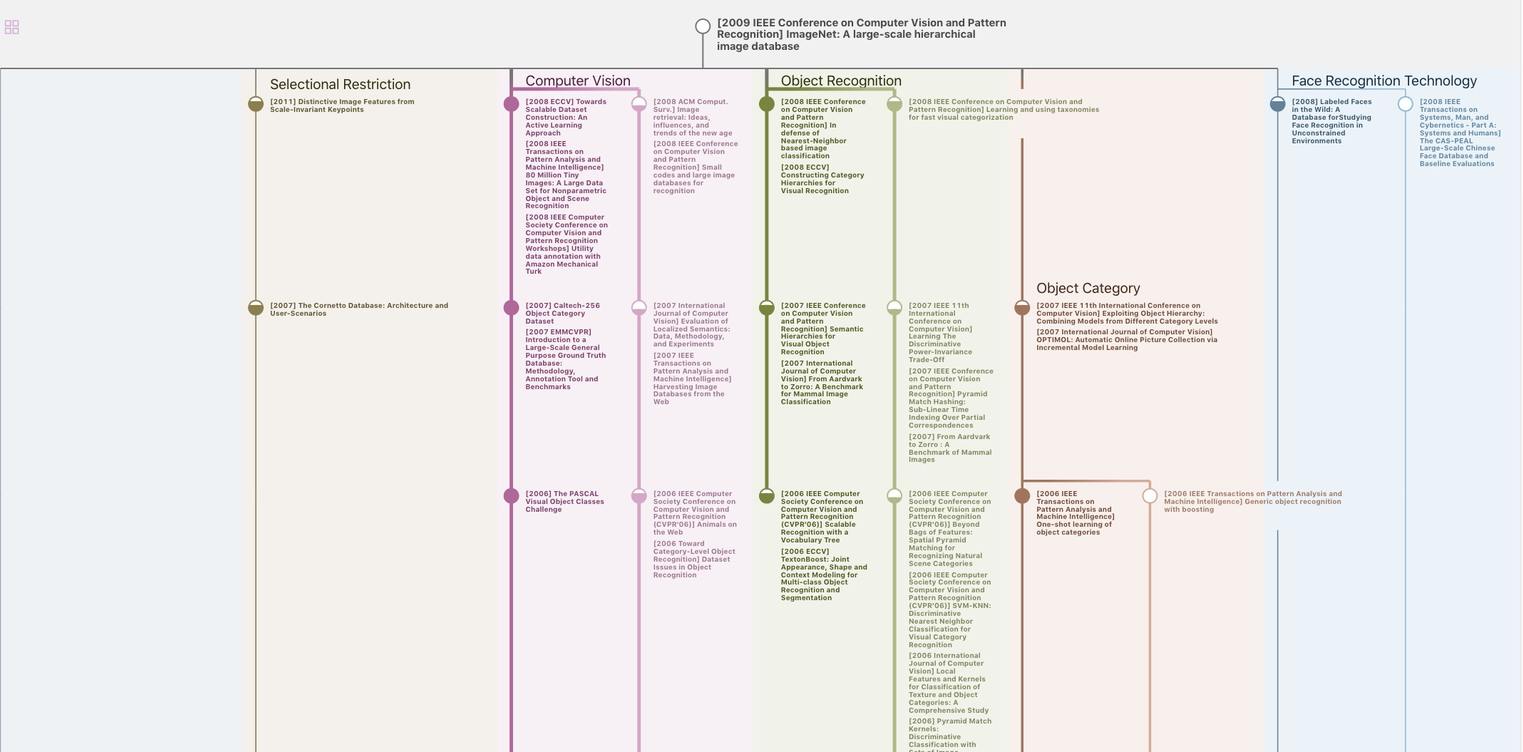
生成溯源树,研究论文发展脉络
Chat Paper
正在生成论文摘要