Unified Brain MR-Ultrasound Synthesis Using Multi-modal Hierarchical Representations
MEDICAL IMAGE COMPUTING AND COMPUTER ASSISTED INTERVENTION, MICCAI 2023, PT X(2023)
摘要
We introduce MHVAE, a deep hierarchical variational auto-encoder (VAE) that synthesizes missing images from various modalities. Extending multi-modal VAEs with a hierarchical latent structure, we introduce a probabilistic formulation for fusing multi-modal images in a common latent representation while having the flexibility to handle incomplete image sets as input. Moreover, adversarial learning is employed to generate sharper images. Extensive experiments are performed on the challenging problem of joint intra-operative ultrasound (iUS) and Magnetic Resonance (MR) synthesis. Our model outperformed multi-modal VAEs, conditional GANs, and the current state-of-the-art unified method (ResViT) for synthesizing missing images, demonstrating the advantage of using a hierarchical latent representation and a principled probabilistic fusion operation. Our code is publicly available (https://github.com/ReubenDo/MHVAE).
更多查看译文
关键词
Variational Auto-Encoder,Ultrasound,Brain Resection,Image Synthesis
AI 理解论文
溯源树
样例
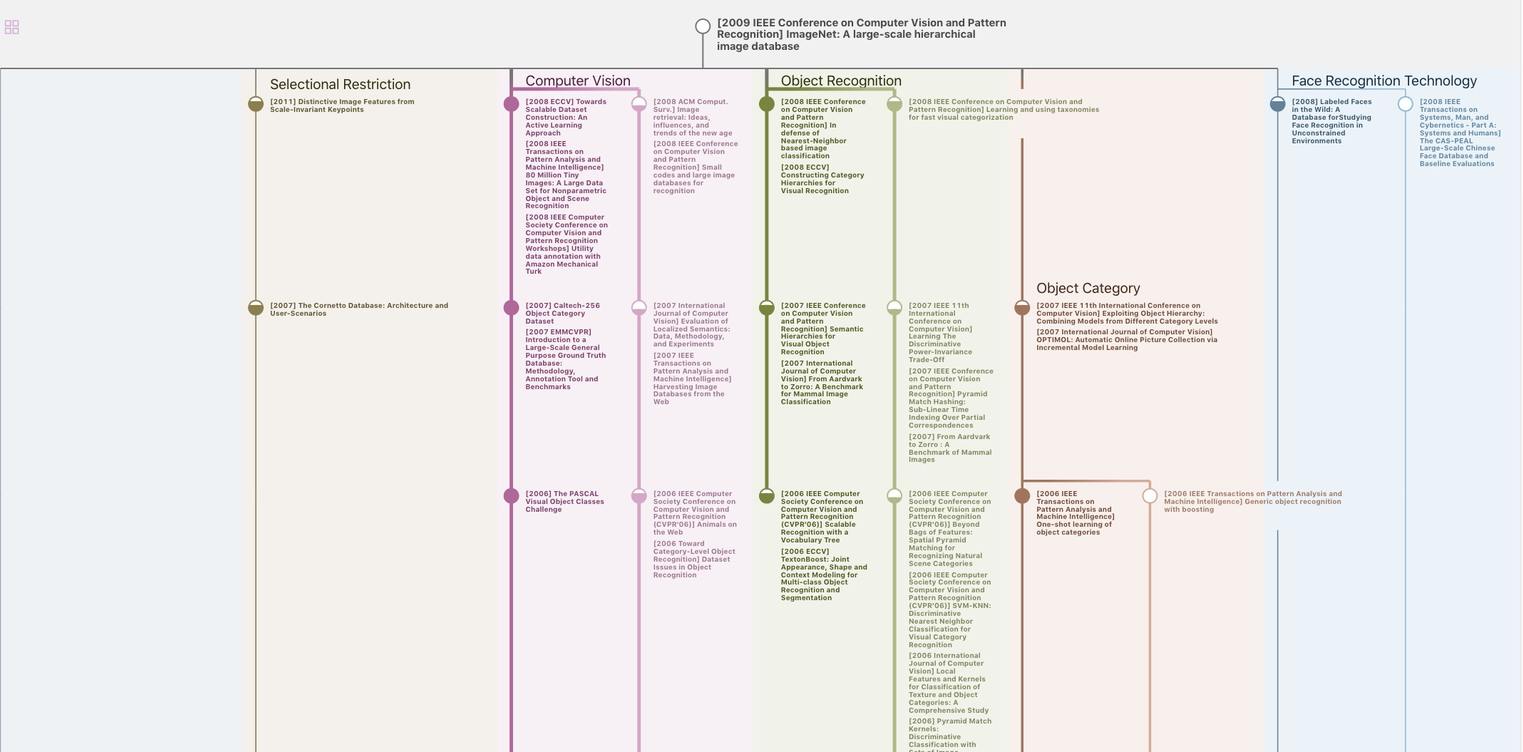
生成溯源树,研究论文发展脉络
Chat Paper
正在生成论文摘要