Do the Frankenstein, or how to achieve better out-of-distribution performance with manifold mixing model soup
CoRR(2023)
摘要
The standard recipe applied in transfer learning is to finetune a pretrained model on the task-specific dataset with different hyperparameter settings and pick the model with the highest accuracy on the validation dataset. Unfortunately, this leads to models which do not perform well under distribution shifts, e.g. when the model is given graphical sketches of the object as input instead of photos. In order to address this, we propose the manifold mixing model soup, an algorithm which mixes together the latent space manifolds of multiple finetuned models in an optimal way in order to generate a fused model. We show that the fused model gives significantly better out-of-distribution performance (+3.5 % compared to best individual model) when finetuning a CLIP model for image classification. In addition, it provides also better accuracy on the original dataset where the finetuning has been done.
更多查看译文
关键词
performance,out-of-distribution
AI 理解论文
溯源树
样例
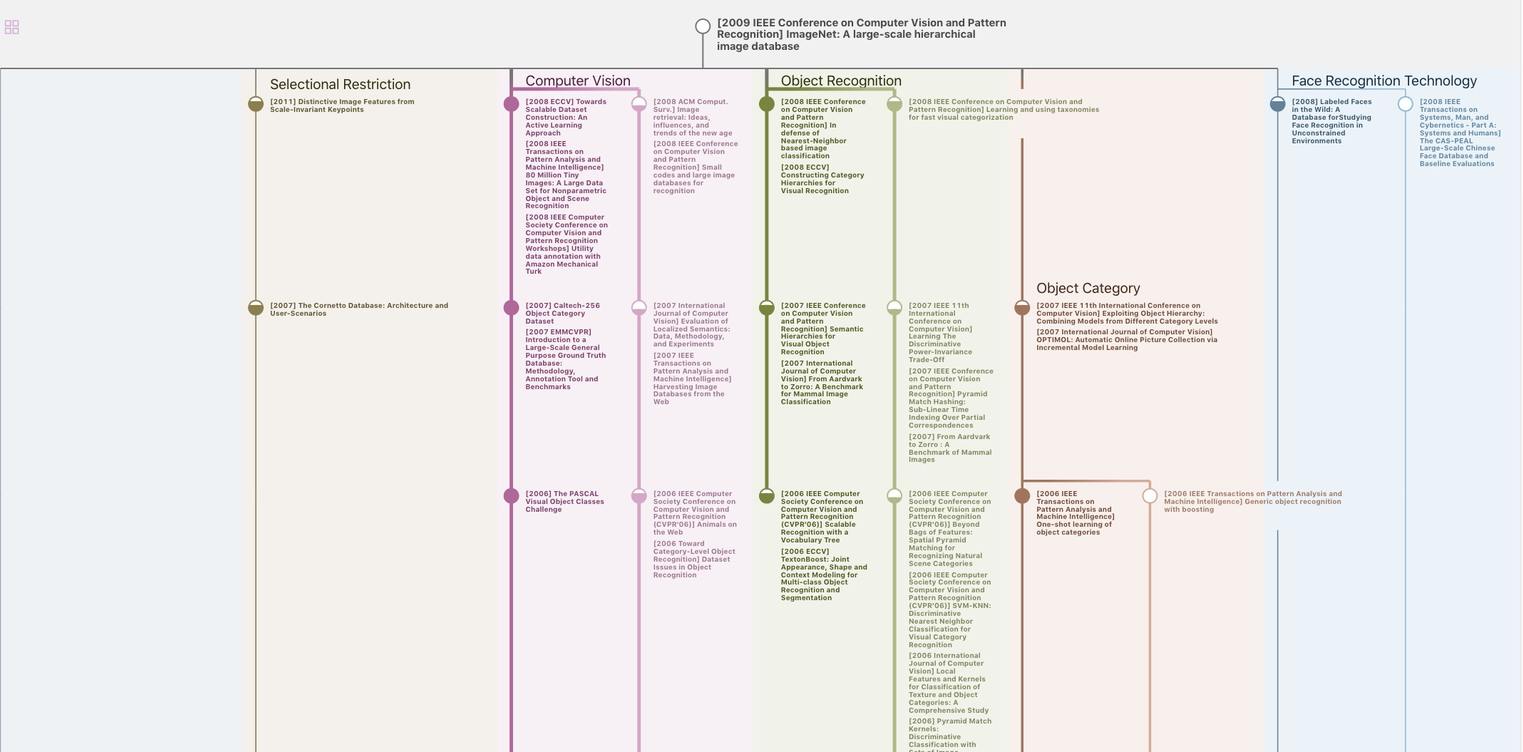
生成溯源树,研究论文发展脉络
Chat Paper
正在生成论文摘要