A new machine-learning model to predict long-term renal function impairment after minimally invasive partial nephrectomy: the Fundació Puigvert predictive model
World Journal of Urology(2023)
摘要
Purpose To provide a new model to predict long-term renal function impairment after partial nephrectomy (PN). Methods Data of consecutive patients who underwent minimally invasive PN from 2005 to 2022 were analyzed. A minimum of 12 months of follow-up was required. We relied on a machine-learning algorithm, namely classification and regression tree (CART), to identify the predictors and associated clusters of chronic kidney disease (CKD) stage migration during follow-up. Results 568 patients underwent minimally invasive PN at our center. A total of 381 patients met our inclusion criteria. The median follow-up was 69 (IQR 38–99) months. A total of 103 (27%) patients experienced CKD stage migration at last follow-up. Progression of CKD stage after surgery, ACCI and baseline CKD stage were selected as the most informative risk factors to predict CKD progression, leading to the creation of four clusters. The progression of CKD stage rates for cluster #1 (no progression of CKD stage after surgery, baseline CKD stage 1–2, ACCI 1–4), #2 (no progression of CKD stage after surgery, baseline CKD stage 1–2, ACCI ≥ 5), #3 (no progression of CKD stage after surgery and baseline CKD stage 3–4–5) and #4 (progression of CKD stage after surgery) were 6.9%, 28.2%, 37.1%, and 69.6%, respectively. The c-index of the model was 0.75. Conclusion We developed a new model to predict long-term renal function impairment after PN where the perioperative loss of renal function plays a pivotal role to predict lack of functional recovery. This model could help identify patients in whom functional follow-up should be intensified to minimize possible worsening factors of renal function.
更多查看译文
关键词
Renal function,CKD,AKI,Partial nephrectomy,Nephron-sparing surgery
AI 理解论文
溯源树
样例
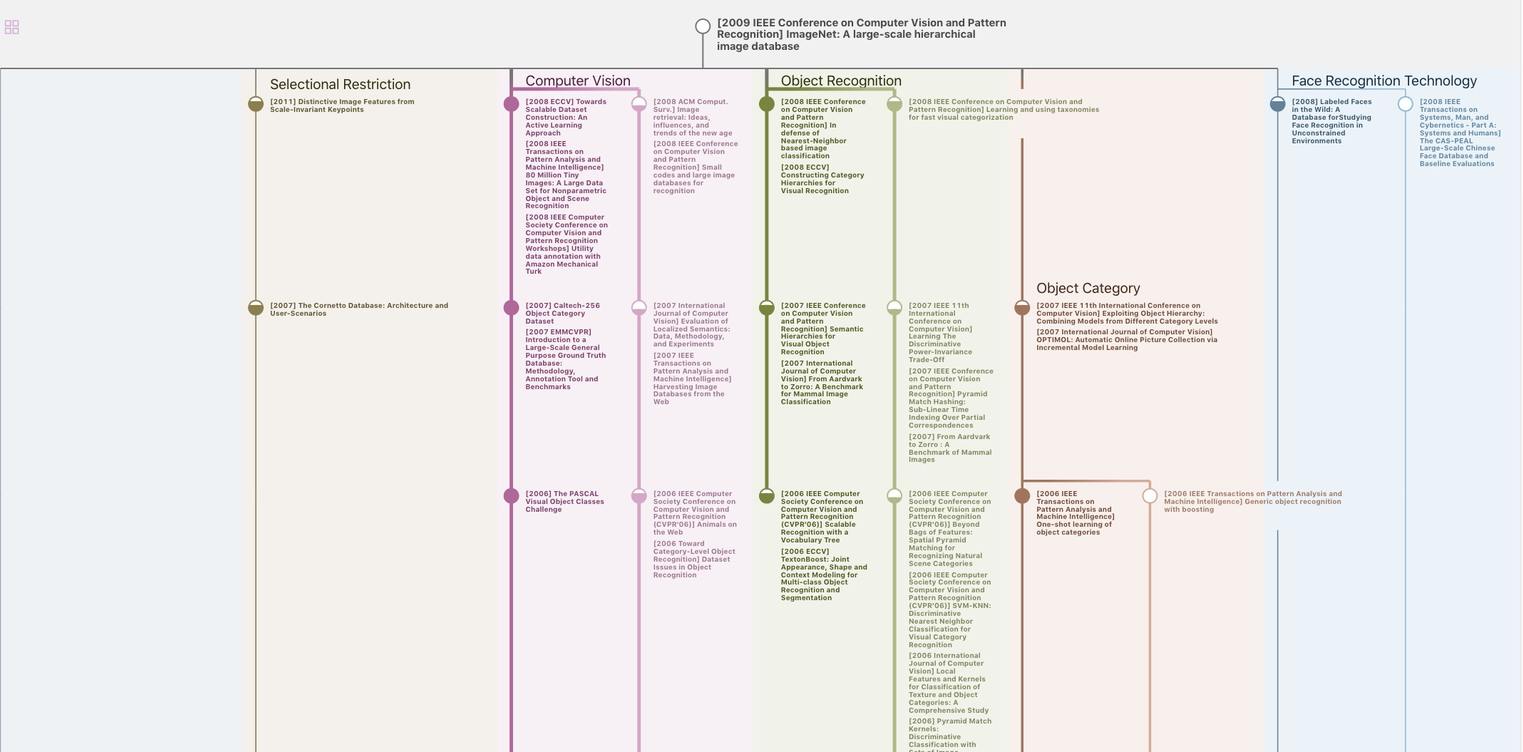
生成溯源树,研究论文发展脉络
Chat Paper
正在生成论文摘要