Charting the Realms of Mesoscale Cloud Organisation using Unsupervised Learning
arXiv (Cornell University)(2023)
摘要
Quantifying the driving mechanisms and effect on Earth's energy budget of mesoscale cloud organisation remains difficult, in part because quantifying the organisational state of the atmosphere through an objective means remains a challenge. We present the first technique to map the full continuum of convective organisation states by extracting the manifold of the internal representation used by a neural network trained through unsupervised learning. By compositing reanalysis and remote sensing observations onto this manifold, we are able to quantify how wind-speed and water vapour concentration are key environmental characteristics associated with changes in organisation. Using the manifold we place distinct organisational regimes, defined in prior work, into the full continuum of organisation. We quantify for the first time, how mesoscale shallow cloud organisation affects Earth's albedo in the tropics besides simply changing the cloud-fraction; changes in cloud-optical depth produce changes in albedo in the range of $\pm 1.4\%$ in addition to what would be predicted from cloud-fraction alone. And, finally we demonstrate how using the continuum representation of this manifold technique enables analysis of the temporal evolution of cloud organisation. The presented manifold of mesoscale cloud organisation provides a novel means to quantify cloud organisation, enabling the study of mechanisms behind transitions between regimes (in simulations and observations) by capturing the full continuum of organisational regimes. This will enable future work to quantify how well models of atmospheric flow capture mesoscale cloud organisation, in turn paving the way for better representation of cloud organisation in simulations of Earth's climate.
更多查看译文
关键词
mesoscale cloud organisation,unsupervised
AI 理解论文
溯源树
样例
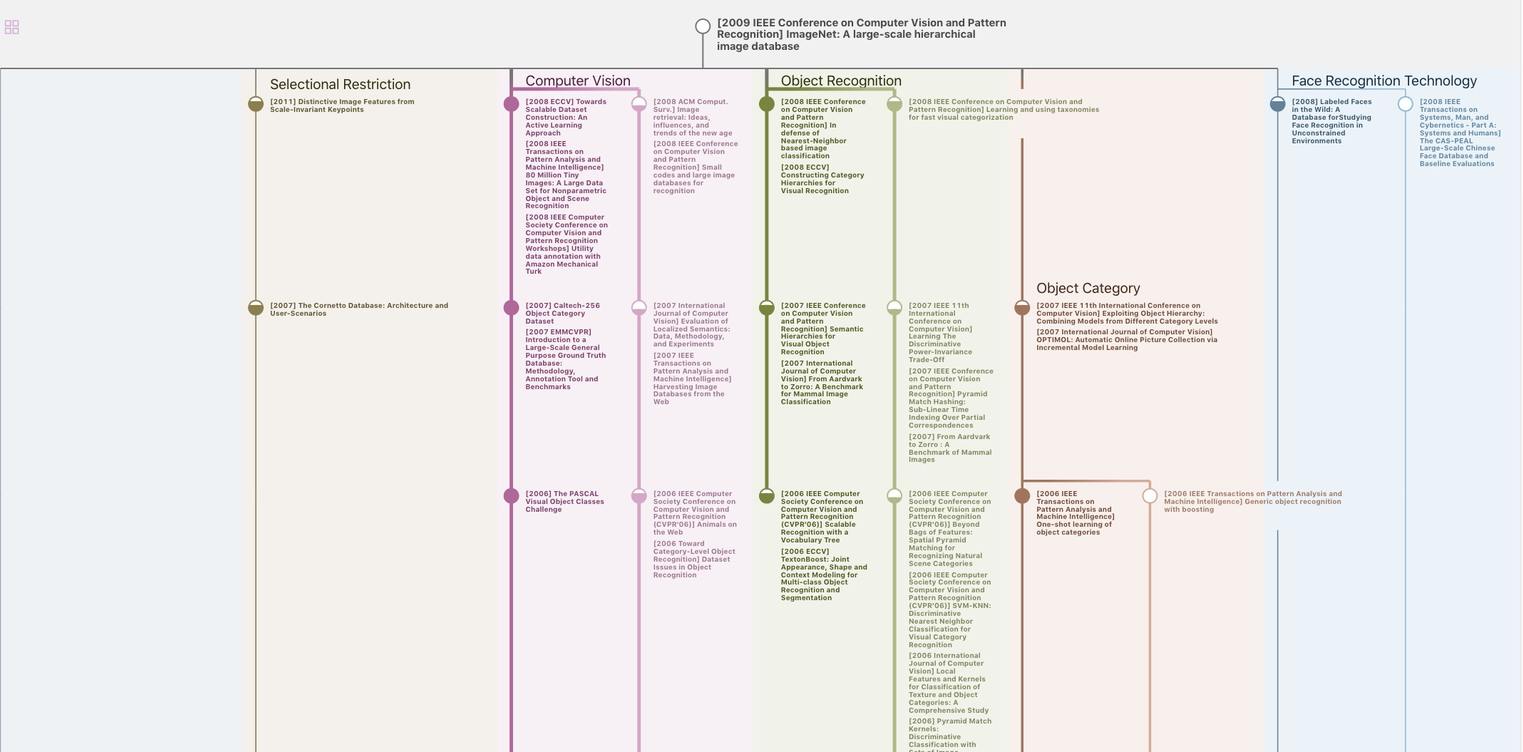
生成溯源树,研究论文发展脉络
Chat Paper
正在生成论文摘要