Scaling Laws for Sparsely-Connected Foundation Models
ICLR 2024(2023)
摘要
We explore the impact of parameter sparsity on the scaling behavior of Transformers trained on massive datasets (i.e., "foundation models"), in both vision and language domains. In this setting, we identify the first scaling law describing the relationship between weight sparsity, number of non-zero parameters, and amount of training data, which we validate empirically across model and data scales; on ViT/JFT-4B and T5/C4. These results allow us to characterize the "optimal sparsity", the sparsity level which yields the best performance for a given effective model size and training budget. For a fixed number of non-zero parameters, we identify that the optimal sparsity increases with the amount of data used for training. We also extend our study to different sparsity structures (such as the hardware-friendly n:m pattern) and strategies (such as starting from a pretrained dense model). Our findings shed light on the power and limitations of weight sparsity across various parameter and computational settings, offering both theoretical understanding and practical implications for leveraging sparsity towards computational efficiency improvements.
更多查看译文
关键词
sparsity,scaling,optimal sparsity,efficiency,foundational models,transformers,structured sparsity,pruning
AI 理解论文
溯源树
样例
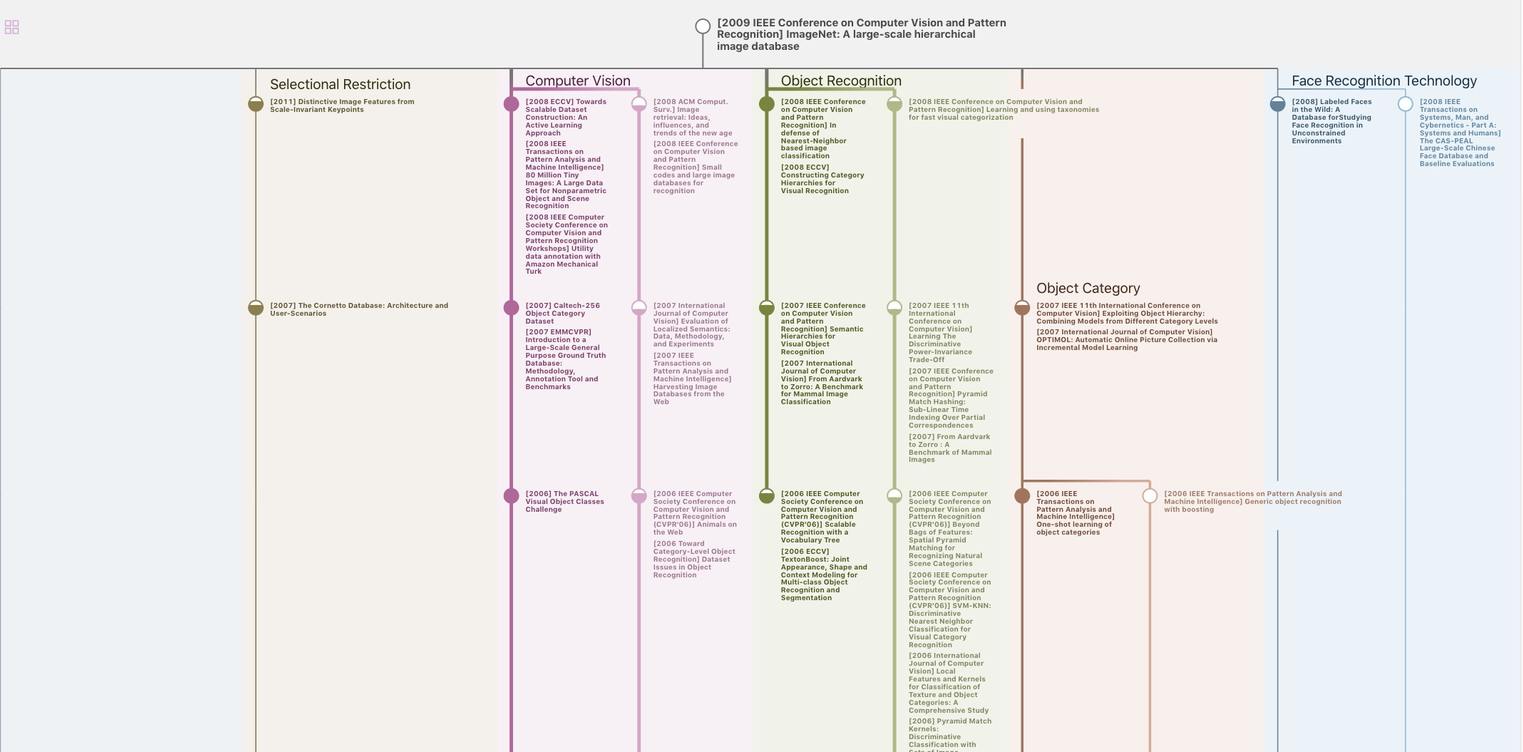
生成溯源树,研究论文发展脉络
Chat Paper
正在生成论文摘要