A Unified View Between Tensor Hypergraph Neural Networks And Signal Denoising
CoRR(2023)
摘要
Hypergraph Neural networks (HyperGNNs) and hypergraph signal denoising (HyperGSD) are two fundamental topics in higher-order network modeling. Understanding the connection between these two domains is particularly useful for designing novel HyperGNNs from a HyperGSD perspective, and vice versa. In particular, the tensor-hypergraph convolutional network (T-HGCN) has emerged as a powerful architecture for preserving higher-order interactions on hypergraphs, and this work shows an equivalence relation between a HyperGSD problem and the T-HGCN. Inspired by this intriguing result, we further design a tensor-hypergraph iterative network (T-HGIN) based on the HyperGSD problem, which takes advantage of a multi-step updating scheme in every single layer. Numerical experiments are conducted to show the promising applications of the proposed T-HGIN approach.
更多查看译文
关键词
tensor hypergraph neural networks,denoising,neural networks,signal
AI 理解论文
溯源树
样例
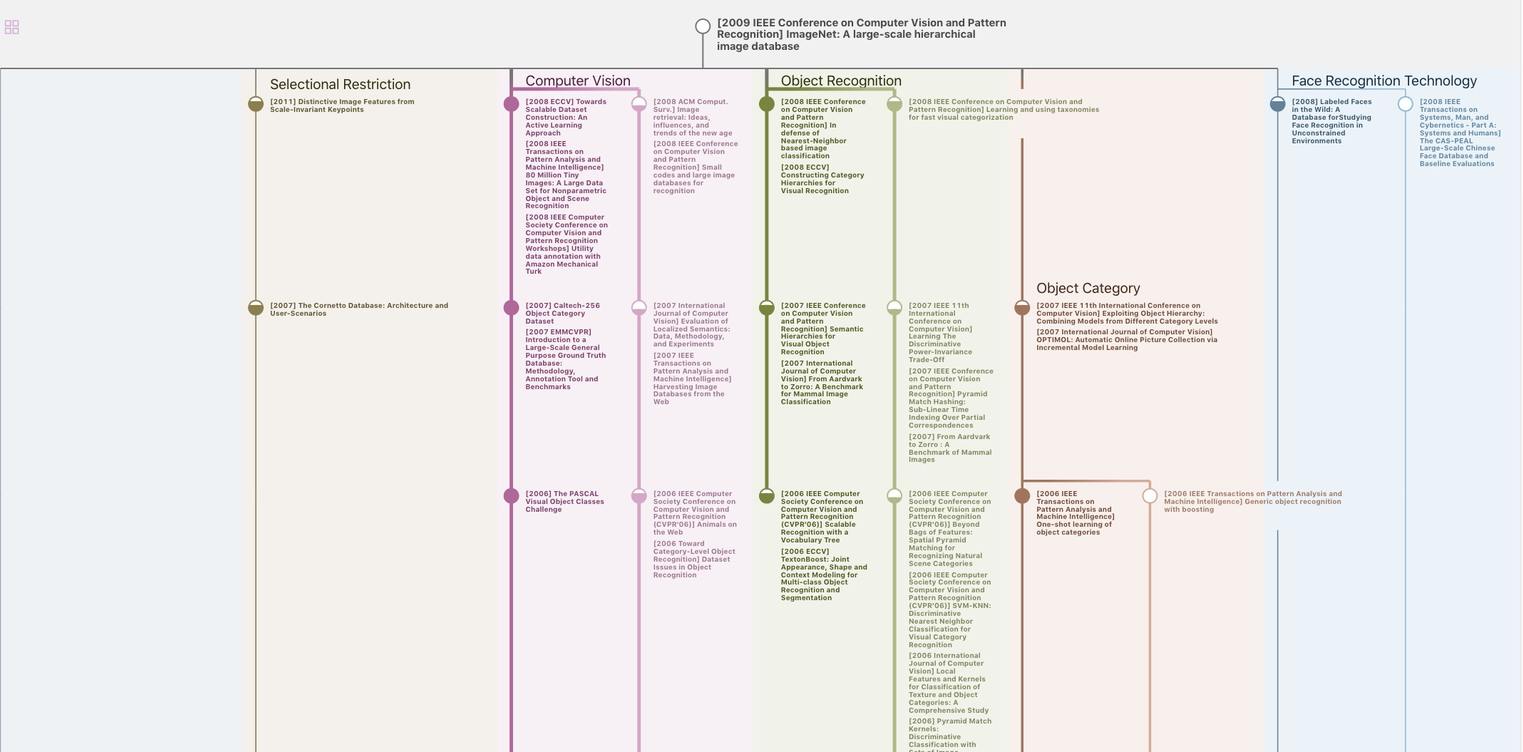
生成溯源树,研究论文发展脉络
Chat Paper
正在生成论文摘要