Yet Another Generative Model for Room Impulse Response Estimation
2023 IEEE Workshop on Applications of Signal Processing to Audio and Acoustics (WASPAA)(2023)
摘要
Recent neural room impulse response (RIR) estimators typically comprise an encoder for reference audio analysis and a generator for RIR synthesis. Especially, it is the performance of the generator that directly influences the overall estimation quality. In this context, we explore an alternate generator architecture for improved performance. We first train an autoencoder with residual quantization to learn a discrete latent token space, where each token represents a small time-frequency patch of the RIR. Then, we cast the RIR estimation problem as a reference-conditioned autoregressive token generation task, employing transformer variants that operate across frequency, time, and quantization depth axes. This way, we address the standard blind estimation task and additional acoustic matching problem, which aims to find an RIR that matches the source signal to the target signal’s reverberation characteristics. Experimental results show that our system is preferable to other baselines across various evaluation metrics.
更多查看译文
关键词
generative model,room,estimation
AI 理解论文
溯源树
样例
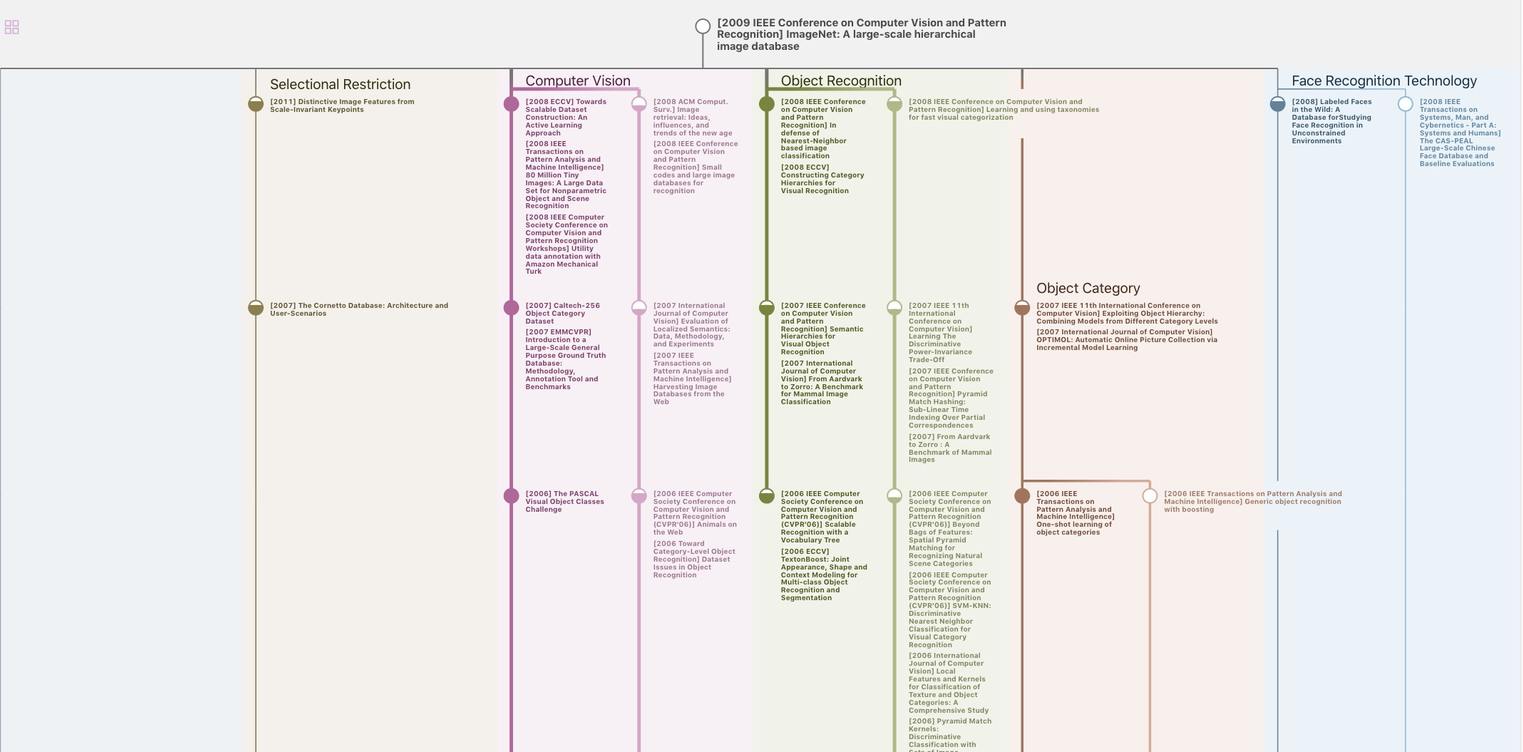
生成溯源树,研究论文发展脉络
Chat Paper
正在生成论文摘要