FLNA: An Energy-Efficient Point Cloud Feature Learning Accelerator with Dataflow Decoupling
2023 60th ACM/IEEE Design Automation Conference (DAC)(2023)
摘要
Grid-based feature learning network plays a key role in recent point-cloud based 3D perception. However, high point sparsity and special operators lead to large memory footprint and long processing latency, posing great challenges to hardware acceleration. We propose FLNA, a novel feature learning accelerator with algorithm-architecture co-design. At algorithm level, the dataflow-decoupled graph is adopted to reduce 86% computation by exploiting inherent sparsity and concat redundancy. At hardware design level, we customize a pipelined architecture with block-wise processing, and introduce transposed SRAM strategy to save 82.1% access power. Implemented on a 40nm technology, FLNA achieves 13.4 − 43.3× speedup over RTX 2080Ti GPU. It rivals the state-of-the-art accelerator by 1.21× energy-efficiency improvement with 50.8% latency reduction.
更多查看译文
关键词
Point Cloud, Feature Learning Accelerator, Algorithm-architecture Co-design, Sparsity Exploitation
AI 理解论文
溯源树
样例
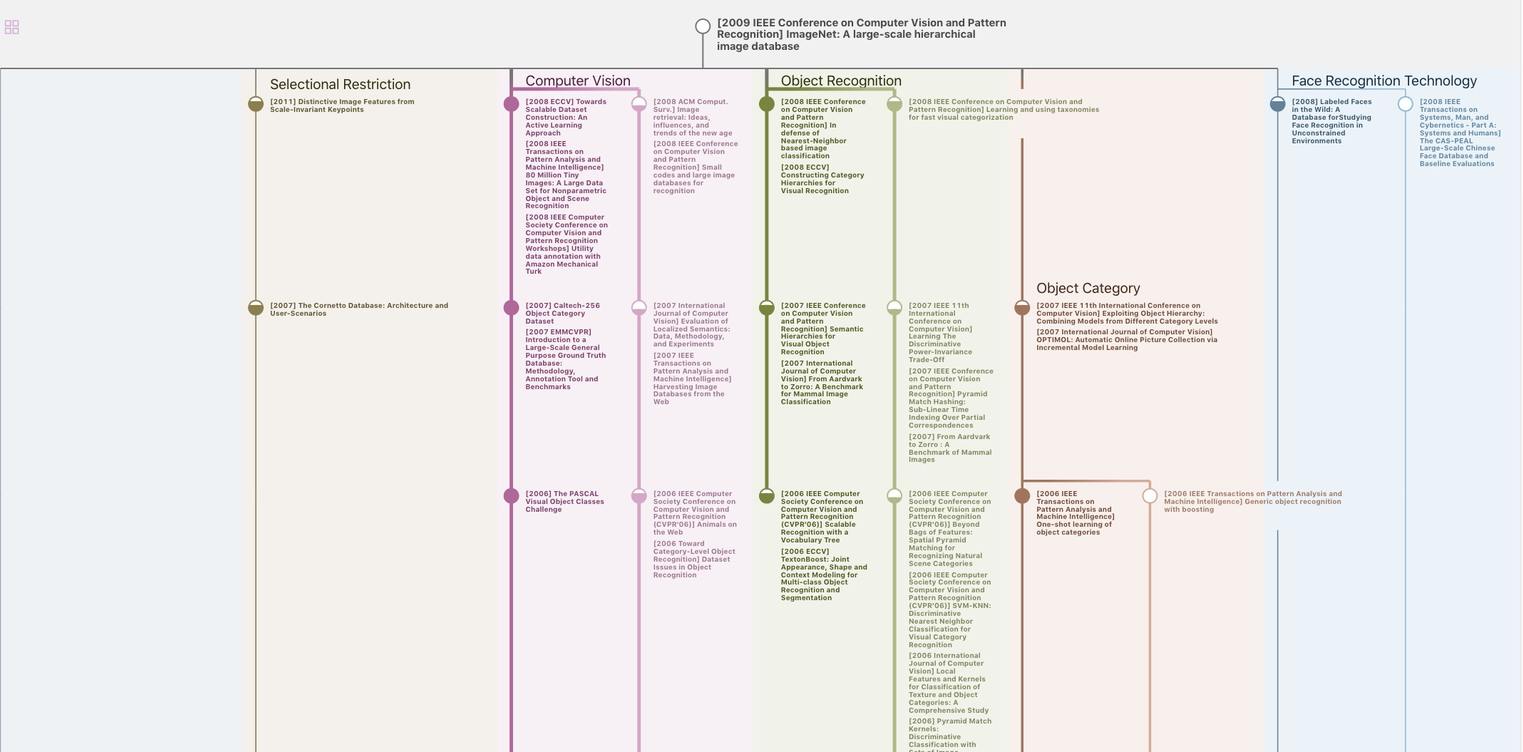
生成溯源树,研究论文发展脉络
Chat Paper
正在生成论文摘要