Physics constraint Deep Learning based radiative transfer model.
Optics express(2023)
摘要
Deep Learning (DL) open sources libraries such as TensorFlow, Keras, and PyTorch have been widely and successfully applied for many applications in a forward model. We have developed the DL radiative transfer model over Oceans under a clear-sky condition. However, the derived physical model from the DL forward model has difficulties in predicting physical properties such as the Jacobian, because multiple solutions can fit the forward model results during the deep learning training process. The Jacobian model in a radiative transfer can calculate radiance sensitivities on geophysical parameters, which are required by satellite radiance assimilation in support of weather forecasts and for retrieving environmental data records. In this study, we introduce a physics constraint into our deep learning training for deriving the forward model that retains right physics. With this physics constraint, the radiance sensitivities are well captured by this new DL radiative transfer.
更多查看译文
关键词
deep learning,physics,constraint
AI 理解论文
溯源树
样例
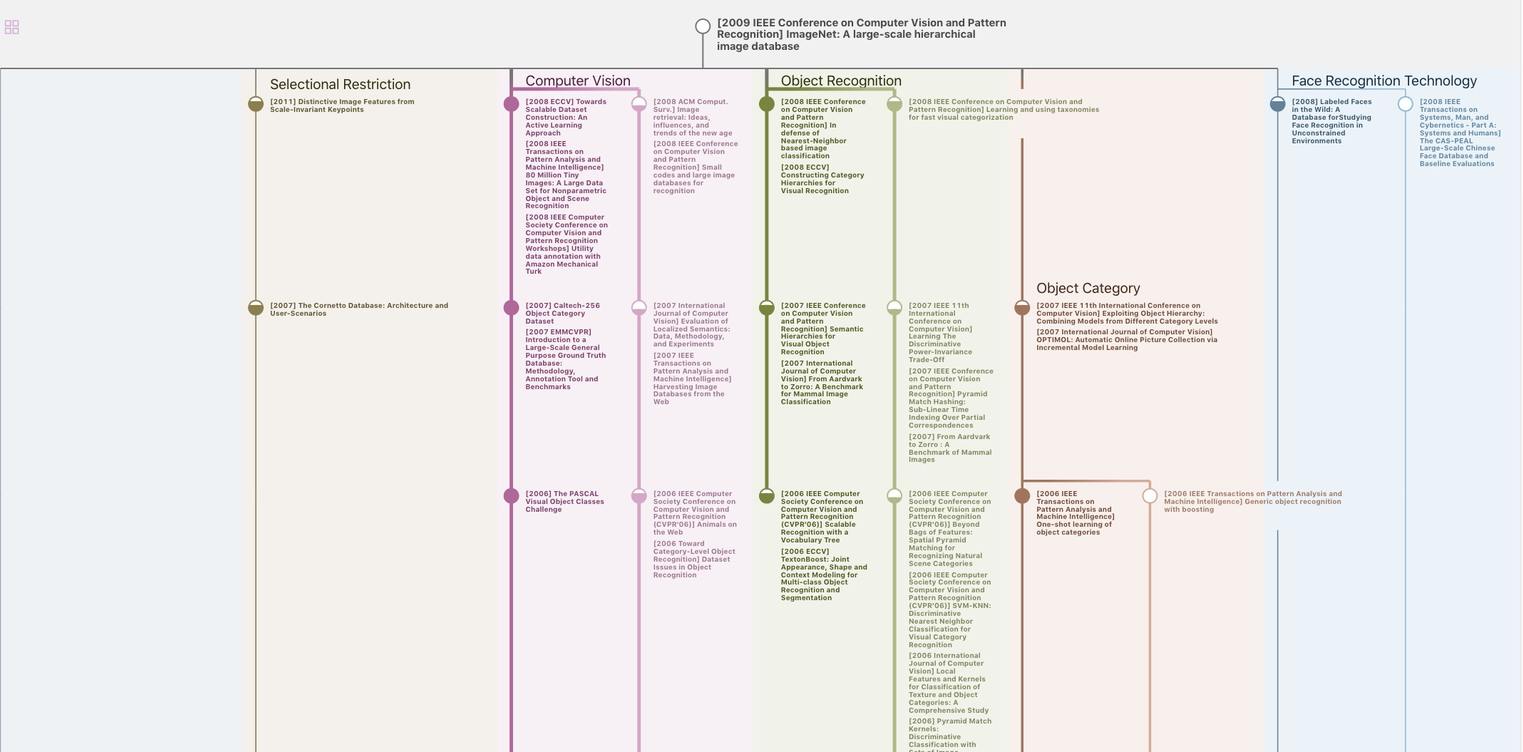
生成溯源树,研究论文发展脉络
Chat Paper
正在生成论文摘要