Predicting methane emissions of individual grazing dairy cows from spectral analyses of their milk samples
JOURNAL OF DAIRY SCIENCE(2024)
摘要
Data on the enteric methane emissions of individual cows are useful not just in assisting management decisions and calculating herd inventories but also as inputs for animal genetic evaluations. Data generation for many animal characteristics, including enteric methane emissions, can be expensive and time consuming, so being able to extract as much information as possible from available samples or data sources is worthy of investigation. The objective of the present study was to attempt to predict individual cow methane emissions from the information contained within milk samples, specifically the spectrum of light transmittance across different wavelengths of the mid-infrared (MIR) region of the electromagnetic spectrum. A total of 93,888 individual spot measures of methane (i.e., individual samples of an animal's breath when using the GreenFeed technology) from 384 lactations on 277 grazing dairy cows were collapsed into weekly averages expressed as grams per day; each weekly average coincided with a MIR spectral analysis of a morning or evening individual cow milk sample. Associations between the spectra and enteric methane measures were performed separately using partial least squares regression or neural networks with different tuning parameters evaluated. Several alternative definitions of the enteric methane phenotype (i.e., average enteric methane in the 6 d preceding or 6 d following taking the milk sample or the average of the 6 d before and after the milk sample, all of which also included the enteric methane emitted on the day of milk sampling), the candidate model features (e.g., milk yield, milk composition, and milk MIR) as well as validation strategy (i.e., cross -validation or leave-oneexperimental treatment-out) were evaluated. Irrespective of the validation method, the prediction accuracy was best when the average of the milk MIR from the morning and evening milk sample was used and the prediction model was developed using neural networks; concurrently including milk yield and days in milk in the prediction model generated superior predictions relative to just the spectral information alone. Furthermore, prediction accuracy was best when the enteric methane phenotype was the average of at least 20 methane spot measures across a 6-d period flanking each side of the milk sample with associated spectral data. Based on the strategy that achieved the best accuracy of prediction, the correlation between the actual and predicted daily methane emissions when based on 4-fold crossvalidation varied per validation stratum from 0.68 to 0.75; the corresponding range when validated on each of the 8 different experimental treatments focusing on alternative pasture grazing systems represented in the dataset varied from 0.55 to 0.71. The root mean square error of prediction across the 4-folds of cross -validation was 37.46 g/d, whereas the root mean square error averaged across all folds of leave-one-treatment-out was 37.50 g/d. Results suggest that even with the likely measurement errors contained within the MIR spectrum and gold standard enteric methane phenotype, enteric methane can be reasonably well predicted from the infrared spectrum of milk samples. What is yet to be established, however, is whether (a) genetic variation exists in this predicted enteric methane phenotype and (b) selection on estimates of genetic merit for this phenotype translate to actual phenotypic differences in enteric methane emissions.
更多查看译文
关键词
mid-infrared spectroscopy,CH4,electromagnetic spectrum
AI 理解论文
溯源树
样例
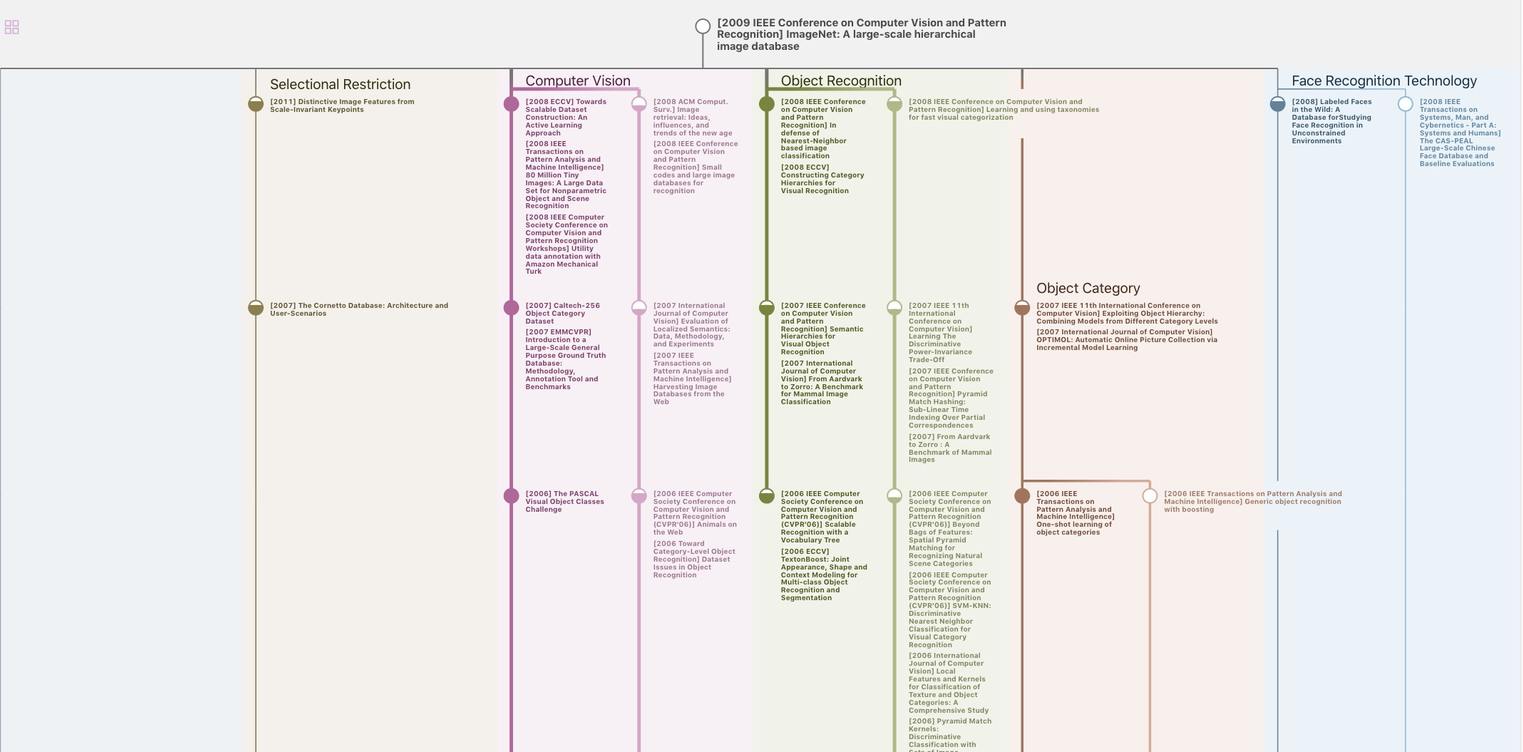
生成溯源树,研究论文发展脉络
Chat Paper
正在生成论文摘要