DEMOS: Clustering by Pruning a Density-Boosting Cluster Tree of Density Mounts
IEEE Transactions on Knowledge and Data Engineering(2023)
摘要
Most existing clustering algorithms require presetting cluster number and often fail to capture complex shapes. Herein, we propose a clustering algorithm by pruning a density-boosting cluster tree of density mounts—DEnsity MOuntains Separation clustering algorithm (DEMOS). A cluster is assumed to be a density-connected area with multiple (or a single) density mounts (i.e., single-peak clusters) and a relatively large dis-connectivity from density-connected areas of higher densities. Based on this assumption, DEMOS can easily detect the number of clusters and robustly reconstruct their complex shapes. It first builds the dataset into a peak graph, where each density peak represents a density mount. A multi-valley-link-based connectivity estimation method is embedded to efficiently estimate the connectivity between density peaks during peak graph building. Then, by applying a new linkage metric designed based on our assumption, DEMOS builds density mounts into a reasonably density-boosting cluster tree. After obtaining a robust center detection in a clarity-enhancing decision graph (i.e., a two-dimensional plot for detecting centers), DEMOS prunes the cluster tree into final clusters to finish clustering. Experimental results on both synthetic and real datasets demonstrated the effectiveness of DEMOS and its applicability to large-scale data clustering.
更多查看译文
关键词
clustering,pruning,density-boosting
AI 理解论文
溯源树
样例
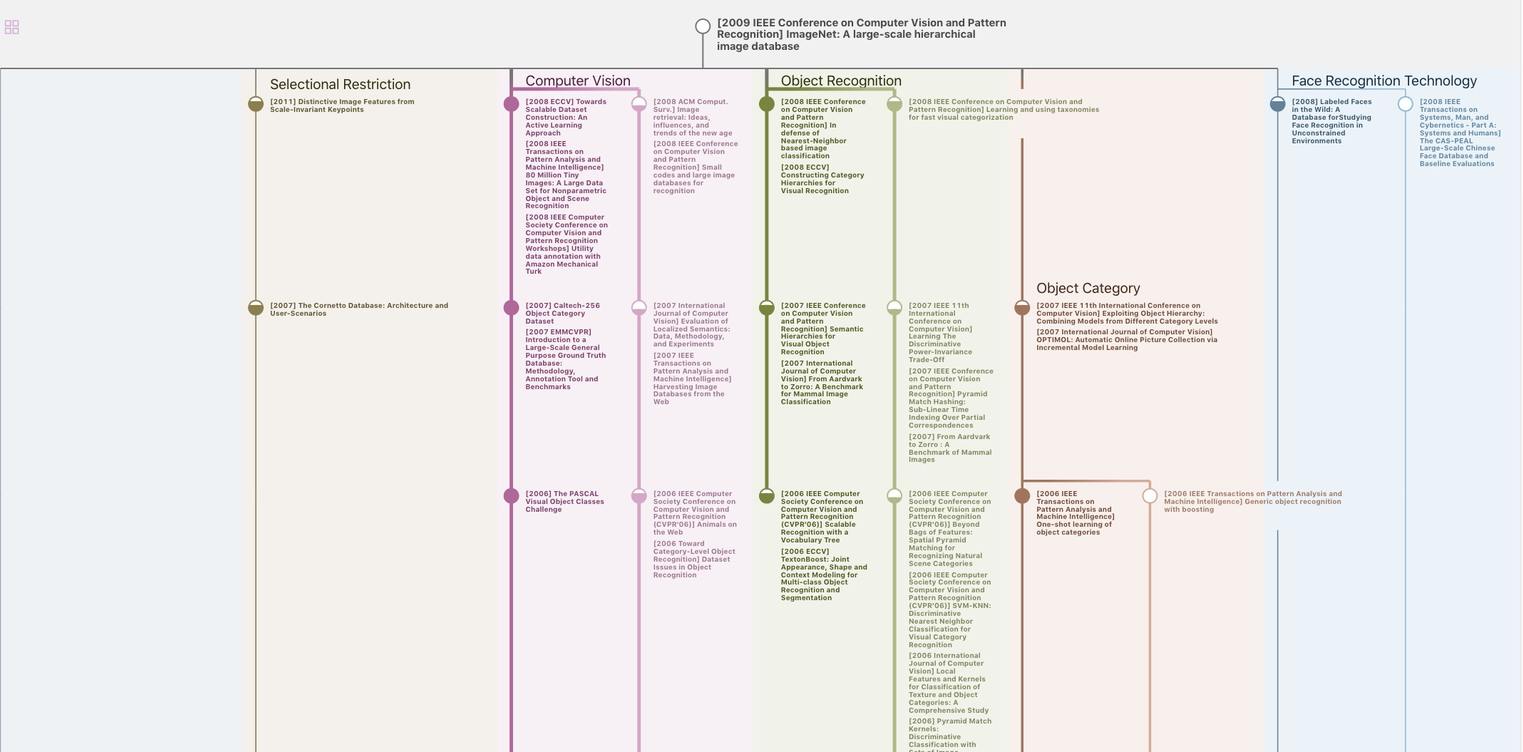
生成溯源树,研究论文发展脉络
Chat Paper
正在生成论文摘要