Learning heterogeneous reaction kinetics from X-ray videos pixel by pixel
Nature(2023)
摘要
Reaction rates at spatially heterogeneous, unstable interfaces are notoriously difficult to quantify, yet are essential in engineering many chemical systems, such as batteries 1 and electrocatalysts 2 . Experimental characterizations of such materials by operando microscopy produce rich image datasets 3 – 6 , but data-driven methods to learn physics from these images are still lacking because of the complex coupling of reaction kinetics, surface chemistry and phase separation 7 . Here we show that heterogeneous reaction kinetics can be learned from in situ scanning transmission X-ray microscopy (STXM) images of carbon-coated lithium iron phosphate (LFP) nanoparticles. Combining a large dataset of STXM images with a thermodynamically consistent electrochemical phase-field model, partial differential equation (PDE)-constrained optimization and uncertainty quantification, we extract the free-energy landscape and reaction kinetics and verify their consistency with theoretical models. We also simultaneously learn the spatial heterogeneity of the reaction rate, which closely matches the carbon-coating thickness profiles obtained through Auger electron microscopy (AEM). Across 180,000 image pixels, the mean discrepancy with the learned model is remarkably small (<7%) and comparable with experimental noise. Our results open the possibility of learning nonequilibrium material properties beyond the reach of traditional experimental methods and offer a new non-destructive technique for characterizing and optimizing heterogeneous reactive surfaces.
更多查看译文
关键词
heterogeneous reaction kinetics,pixel,learning,x-ray
AI 理解论文
溯源树
样例
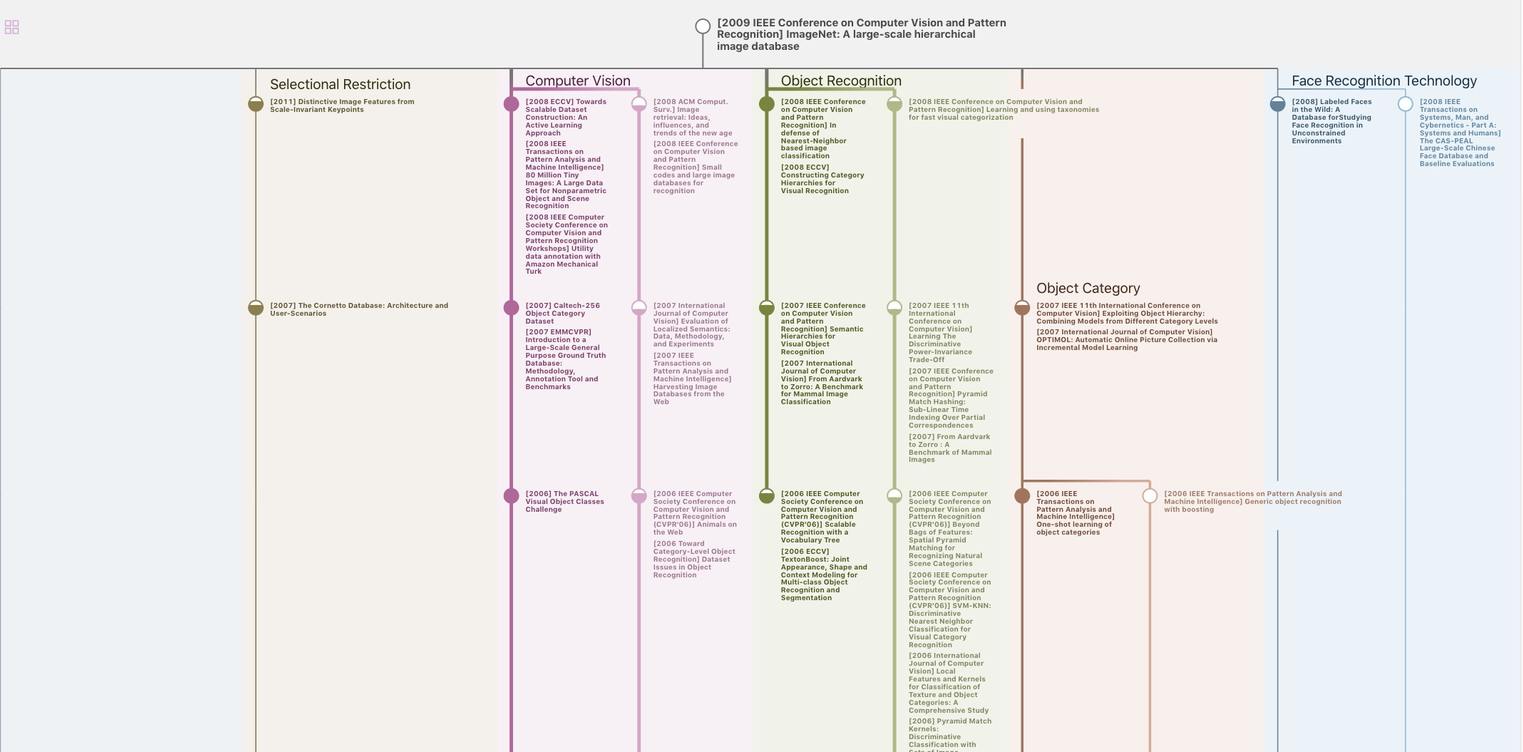
生成溯源树,研究论文发展脉络
Chat Paper
正在生成论文摘要