TeaBERT: An Efficient Knowledge Infused Cross-Lingual Language Model for Mapping Chinese Medical Entities to the Unified Medical Language System
IEEE JOURNAL OF BIOMEDICAL AND HEALTH INFORMATICS(2023)
摘要
Medical entity normalization is an important task for medical information processing. The Unified Medical Language System (UMLS), a well-developed medical terminology system, is crucial for medical entity normalization. However, the UMLS primarily consists of English medical terms. For languages other than English, such as Chinese, a significant challenge for normalizing medical entities is the lack of robust terminology systems. To address this issue, we propose a translation-enhancing training strategy that incorporates the translation and synonym knowledge of the UMLS into a language model using the contrastive learning approach. In this work, we proposed a cross-lingual pre-trained language model called TeaBERT, which can align synonymous Chinese and English medical entities across languages at the concept level. As the evaluation results showed, the TeaBERT language model outperformed previous cross-lingual language models with Acc@5 values of 92.54%, 87.14% and 84.77% on the ICD10-CN, CHPO and RealWorld-v2 datasets, respectively. It also achieved a new state-of-the-art cross-lingual entity mapping performance without fine-tuning. The translation-enhancing strategy is applicable to other languages that face the similar challenge due to the absence of well-developed medical terminology systems.
更多查看译文
关键词
Cross-lingual pre-trained language model,deep learning,entity linking,UMLS
AI 理解论文
溯源树
样例
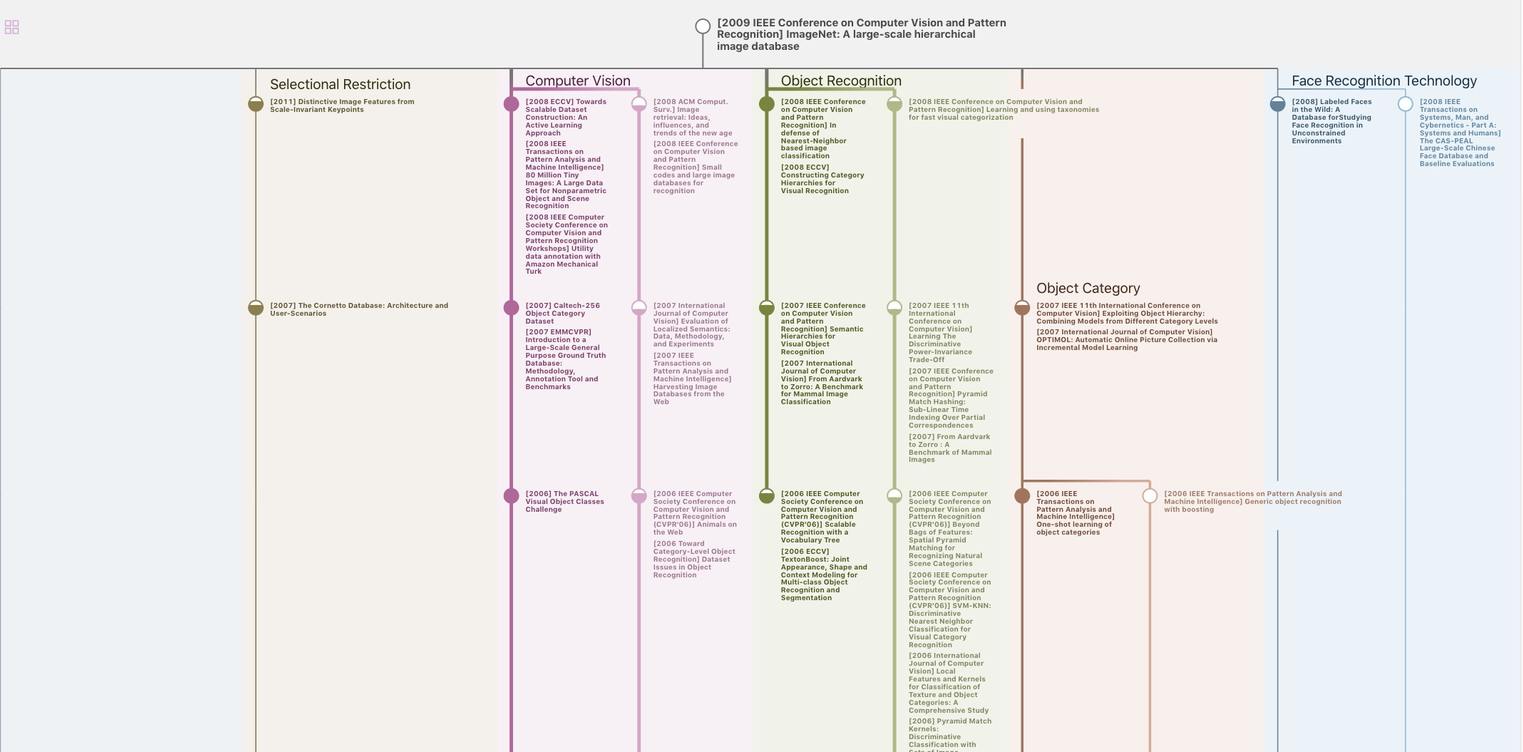
生成溯源树,研究论文发展脉络
Chat Paper
正在生成论文摘要