CoLLD: Contrastive Layer-to-layer Distillation for Compressing Multilingual Pre-trained Speech Encoders
ICASSP 2024 - 2024 IEEE International Conference on Acoustics, Speech and Signal Processing (ICASSP)(2023)
摘要
Large-scale self-supervised pre-trained speech encoders outperform conventional approaches in speech recognition and translation tasks. Due to the high cost of developing these large models, building new encoders for new tasks and deploying them to on-device applications are infeasible. Prior studies propose model compression methods to address this issue, but those works focus on smaller models and less realistic tasks. Thus, we propose Contrastive Layer-to-layer Distillation (CoLLD), a novel knowledge distillation method to compress pre-trained speech encoders by leveraging masked prediction and contrastive learning to train student models to copy the behavior of a large teacher model. CoLLD outperforms prior methods and closes the gap between small and large models on multilingual speech-to-text translation and recognition benchmarks.
更多查看译文
关键词
Self-supervised learning,knowledge distillation,model compression,multilingual speech translation
AI 理解论文
溯源树
样例
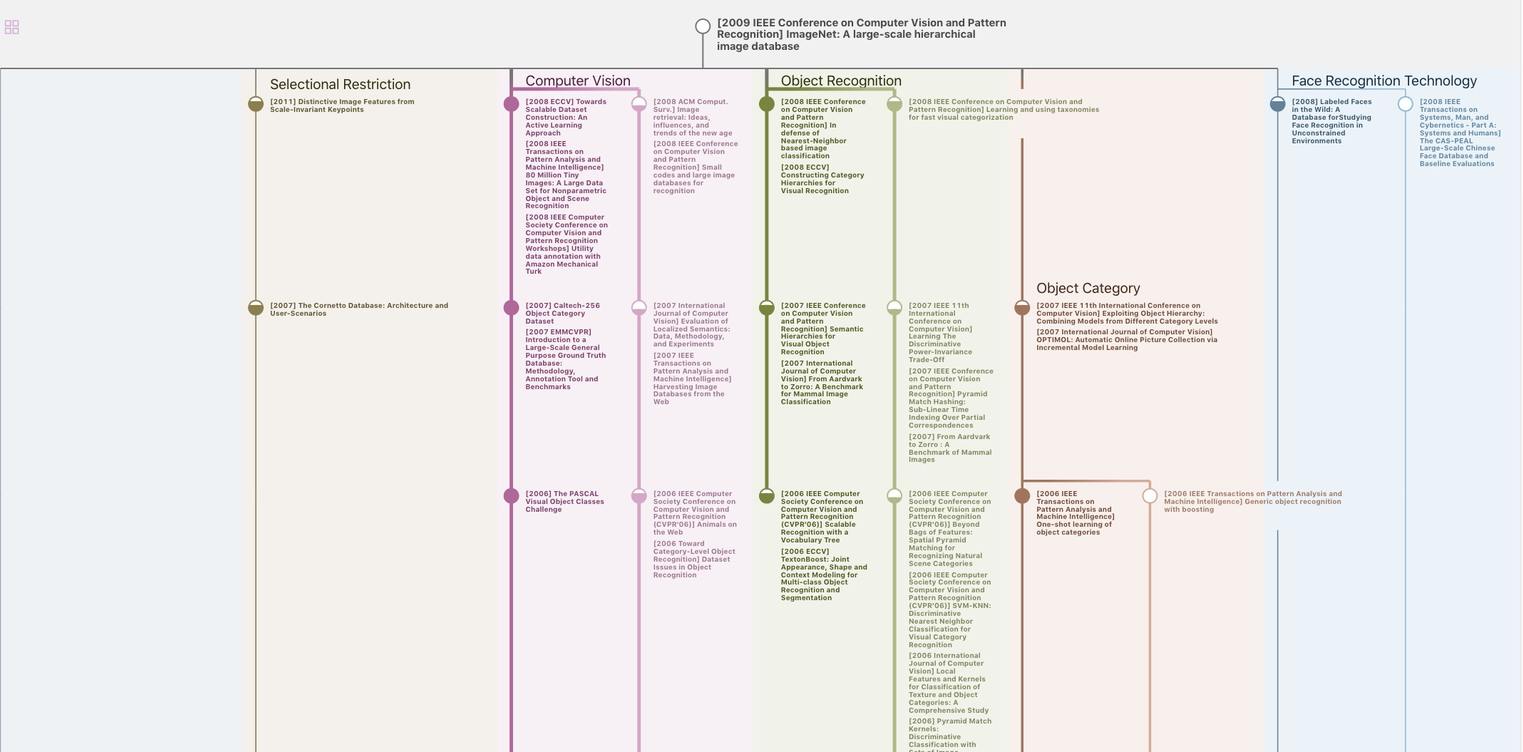
生成溯源树,研究论文发展脉络
Chat Paper
正在生成论文摘要