Semantic reconstruction of continuous language from MEG signals
arXiv (Cornell University)(2023)
摘要
Decoding language from neural signals holds considerable theoretical and practical importance. Previous research has indicated the feasibility of decoding text or speech from invasive neural signals. However, when using non-invasive neural signals, significant challenges are encountered due to their low quality. In this study, we proposed a data-driven approach for decoding semantic of language from Magnetoencephalography (MEG) signals recorded while subjects were listening to continuous speech. First, a multi-subject decoding model was trained using contrastive learning to reconstruct continuous word embeddings from MEG data. Subsequently, a beam search algorithm was adopted to generate text sequences based on the reconstructed word embeddings. Given a candidate sentence in the beam, a language model was used to predict the subsequent words. The word embeddings of the subsequent words were correlated with the reconstructed word embedding. These correlations were then used as a measure of the probability for the next word. The results showed that the proposed continuous word embedding model can effectively leverage both subject-specific and subject-shared information. Additionally, the decoded text exhibited significant similarity to the target text, with an average BERTScore of 0.816, a score comparable to that in the previous fMRI study.
更多查看译文
关键词
meg signals,semantic reconstruction,continuous language
AI 理解论文
溯源树
样例
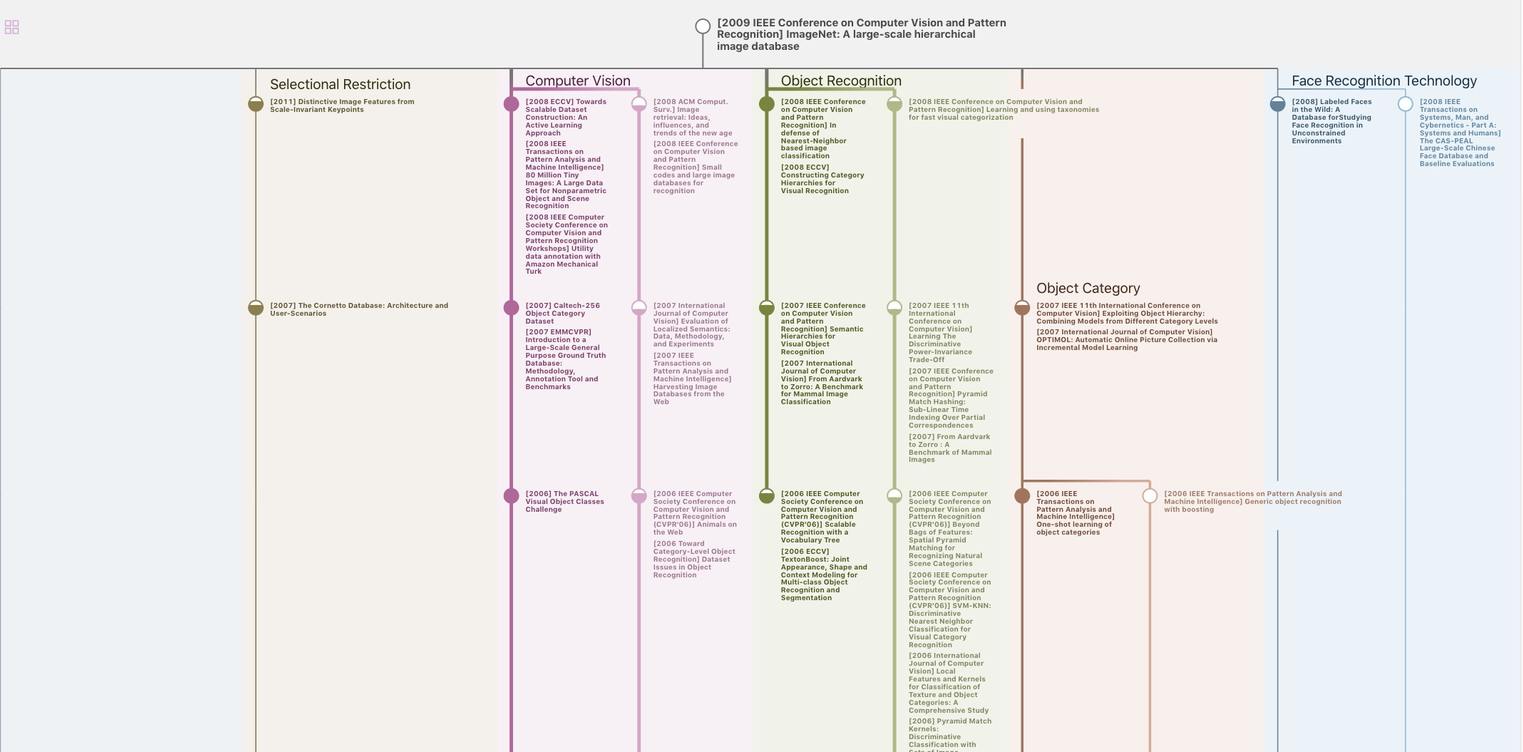
生成溯源树,研究论文发展脉络
Chat Paper
正在生成论文摘要